If we want medicine to be evidence-based, what should we think when the evidence doesn't agree?
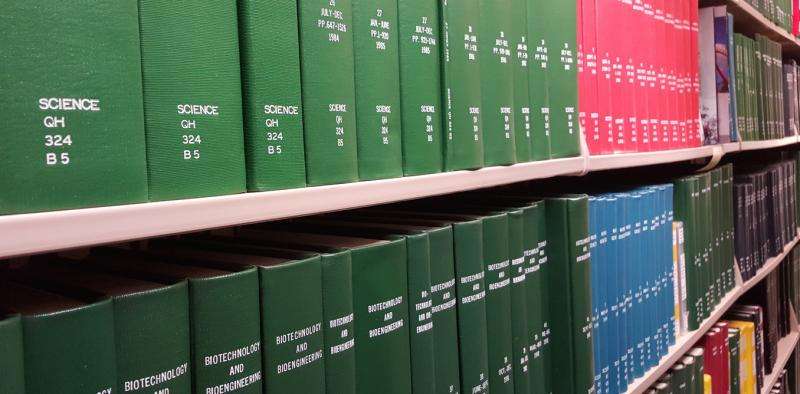
To understand if a new treatment for an illness is really better than older treatments, doctors and researchers look to the best available evidence. Health professionals want a "last word" in evidence to settle questions about what the best modes of treatment are.
But not all medical evidence is created equal. And there is a clear hierarchy of evidence: expert opinion and case reports about individual events are at the lowest tier, and well-conducted randomized controlled trials are near the top. At the very top of this hierarchy are meta-analyses – studies that combine the results from multiple studies that asked the same question. And the very, very top of this hierarchy are meta-analyses performed by a group called the Cochrane Collaboration.
To be a member of the Cochrane Collaboration, individual researchers or research groups are required to adhere to very strict guidelines about how meta-analyses are to be reported and conducted. That's why Cochrane reviews are generally considered to be the best meta-analyses.
However, no one has ever asked if the results in meta-analyses performed by the Cochrane Collaboration are different from meta-analyses from other sources. In theory, if you compared a Cochrane and non-Cocrhane meta-analysis, both published within a similar time frame, you'd tend to expect that they'd have chosen the same studies to analyze, and that their results and interpretation would more or less match up.
Our team at Boston University's School of Public Health decided to find out. And surprisingly, that's not what we found.
What is a meta-analysis, anyway?
Imagine you have five small clinical trials that all found a generally positive benefit for, let's say, taking aspirin to prevent heart attacks. But because each of the studies only had a small number of study subjects, none could confidently state that the beneficial effects weren't simply due to chance. In statistical-speak, such studies would be deemed "underpowered."
There is a good way to increase the statistical power of those studies: combine those five smaller studies into one. That's what a meta-anaysis does. Combining several smaller studies into one analysis and taking the average of those studies can sometimes tip the scales, and let the medical community know with confidence whether a given intervention works, or not.
Meta-analyses are efficient and cheap because they don't require running new trials. Rather, it's a matter of finding all of the relevant studies that have already been published, and this can be surprisingly difficult. Researchers have to be persistent and methodical in their search. Finding studies and deciding whether they are good enough to trust is where the art – and error – of this science becomes a critical issue.
That's actually a major reason why the Cochrane Collaboration was founded. Archie Cochrane, a health services researcher, recognized the power of meta-analyses, but also the tremendous importance of doing them right. The Cochrane Collaboration meta-analyses must adhere to very high standards of transparency and methodological rigor and reproducibility.
Unfortunately, few can commit the time and effort to join the Cochrane Collaboration, and that means that the vast majority of meta-analyses are not conducted by the Collaboration, and are not bound to adhere to their standards. But does this actually matter?
How different can two meta-analyses be?
To find out, we started by identifying 40 pairs of meta-analyses, one from Cochrane and one not, that covered the same intervention (e.g., aspirin) and outcome (e.g., heart attacks), and then compared and contrasted them.
First, we found that almost 40 percent of the Cochrane and non-Cochrane meta-analyses disagreed in their bottom-line statistical answers. That means that typical readers, doctors or health policymakers, for instance, would come up with a fundamentally different interpretation of whether the intervention was effective or not, depending on which meta-anlyses they happened to read.
Second, these differences appeared to be systematic. The non-Cochrane reviews, on average, tended to suggest that the interventions they were testing were more potent, more likely to cure the condition or avert some medical complication than the Cochrane reviews suggested. At the same time, the non-Cochrane reviews were less precise in their accuracy, meaning that there was a higher chance that the findings were merely due to chance.
A meta-analysis is nothing more than just a fancy weighted average of its component studies. We were surprised to find that approximately 63 percent of the included studies were unique to one or the other set of meta-analyses. In other words, despite the fact that the two sets of meta-analyses would presumably look for the same papers, using similar search criteria, over a similar period of time and from similar databases, only about a third of the papers the two sets had included were the same.
It seems likely that most or all of these differences come down to the fact that Cochrane insists on tougher criteria. A meta-analysis is only as good as the studies it includes, and taking the average of poor research can lead to a poor result. As the saying goes, "garbage in, garbage out."
Interestingly, the analyses that reported much higher effect sizes tended to get cited again in other papers at a much higher rate than the analyses reporting the lower effect size. This is a statistical embodiment of the old journalistic saying "If it bleeds, it leads." Big and bold effects get more attention than results showing marginal or equivocal outcomes. The medical community is, after all, just human.
Why does this matter?
At its most basic level, this shows that Archie Cochrane was absolutely correct. Methodological consistency and rigor and transparency are essential. Without that, there's a risk of concluding that something works when it doesn't, or even just overhyping benefits.
But at a higher level this shows us, yet again, how very difficult it is to generate a unified interpretation of the medical literature. Meta-analyses are often used as the final word on a given subject, as the arbiters of ambiguity.
Clearly that role is challenged by the fact that two meta-analyses, ostensibly on the same topic, can reach different conclusions. If we view the meta-analysis as the "gold standard" in our current era of "evidence-based medicine," how is the average doctor or policymaker or even patient to react when two gold standards contradict each other? Caveat emptor.
This article was originally published on The Conversation. Read the original article.