Psychological science—the good, the bad, and the statistically significant
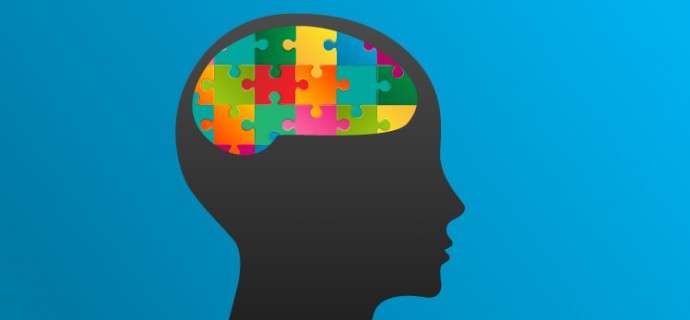
Discussing political science's (possible) contributions to scientific understanding of our current bizarre political scene here last week, I mentioned long-standing critiques of social science research, psychology in particular. Meantime, a new set of critiques of the psychology literature had erupted. In the pages of the journal Science, no less, making said eruption exceptionally newsworthy.
The new paper was an attack on a previous Science paper, which I wrote about here at On Science Blogs when it appeared last August. That paper was a massive attempt at replication of 100 selected research projects published in the top psychology journals in 2008. It showed that only a little more than a third of the papers came up with results consistent with the original study the researchers were trying to confirm.
The new paper argued that the August paper was itself methodologically flawed, and that, contrary to its assertions, "the reproducibility of psychological science is quite high."
In the same issue, Science also published a critique of the critique from several authors of the August 2015 paper, arguing that the new paper was methodologically flawed: the "very optimistic assessment is limited by statistical misconceptions and by causal inferences from selectively interpreted, correlational data."
This group opted to split the difference, concluding that "both optimistic and pessimistic conclusions about reproducibility are possible, and neither are yet warranted." Two authors of the August paper were less dispassionate in yet another reply at Retraction Watch.
Is there a replication crisis in psychology?
Reading the commentaries on these commentaries, it seems likely to this outsider that the latter Science paper's exceptional even-handedness is no more warranted than is the former's optimism. As Ed Yong points out at The Atlantic, there is considerable evidence of trouble in the psychology literature even if you disregard the findings of last August's paper. There have been many failures to replicate. Methodological problems are widespread and often obvious.
If you doubt, read many posts by Andrew Gelman at Statistical Modeling, Causal Inference, and Social Science–especially this one. Part of the problem, Gelman says, is that "Lots of bad stuff is being published in top journals and being promoted in the media." Amen, but there's another big problem too: "Part of my 'pessimistic conclusions about reproducibility' come from the fact that, when problems are revealed, it's a rare researcher who will consider that their original published claim may be mistaken."
At Cross-Check, John Horgan notes, "The exchange [in Science] reveals that psychologists cannot even agree on basic methods for arriving at 'truth,' whatever that is." He worries about the debilitating effect on young hopefuls aiming at a career in psychology. And he tries to put a positive spin on the revelations, asserting that "psychology is arguably healthier than many other fields precisely because psychologists are energetically exposing its weaknesses and seeking ways to overcome them."
Developmental neuropsychologist Dorothy Bishop, at BishopBlog, points out that many many psychology findings remain robust and trustworthy. Still, she bets that things have nonetheless gotten worse. One hypothetical: is it possible that psychology has already plucked the low-hanging fruit and is now focused on investigations where the signal can easily be overwhelmed by noise?
She also notes that psychologists must have training in statistics. "In principle, this is thoroughly good thing, but in practice it can be a disaster if the psychologist is simply fixated on finding p-values less than .05 – and assumes that any effect associated with such a p-value is true."
Which is the perfect segue to:
All about p-values
The American Statistical Association has just pronounced on the fraught topic of p-values, its first official statement ever on a statistical practice. The hope is for illumination, especially for scientists and journalists who haven't really understood what the p-value means. Retraction Watch's Alison McCook describes the statement briefly and does a Q&A with Ron Wasserstein, ASA Executive Director.
In her brave attempt at an explainer at FiveThirtyEight, Christie Aschwanden observes that the ASA's year-long internecine battle over the statement left metaphorical blood on the floor. Which suggests that statisticians themselves don't agree on what a p-value is and does.
The first task was to define the term. Aschwanden says, "They eventually settled on this: 'Informally, a p-value is the probability under a specified statistical model that a statistical summary of the data (for example, the sample mean difference between two compared groups) would be equal to or more extreme than its observed value.' That definition is about as clear as mud." She stoically stands by her declaration in a post from last fall: "I've come to think that the most fundamental problem with p-values is that no one can really say what they are."
No way in the world can I summarize Aschwanden's posts adequately. If you need to understand this stuff in order to do your work, they are a must-read–and so, probably, is the ASA statement and the accompanying 20 commentaries from statisticians that she links to. Here's the latest link again. Also Retraction Watch's Wasserstein interview.
But I will leave you with these very basic cautionary remarks:
From Aschwanden: "A common misconception among nonstatisticians is that p-values can tell you the probability that a result occurred by chance." [About this she is certainly correct; I was taught this in my grad school cookbook statistics course.] "This interpretation is dead wrong . . . The p-value only tells you something about the probability of seeing your results given a particular hypothetical explanation—it cannot tell you the probability that the results are true or whether they're due to random chance."
Got it?
As Wasserstein told McCook: "This is perhaps subtle, but it is not quibbling. It is a most basic logical fallacy to conclude something is true that you had to assume to be true in order to reach that conclusion."
More information: D. T. Gilbert et al. Comment on "Estimating the reproducibility of psychological science", Science (2016). DOI: 10.1126/science.aad7243
This story is republished courtesy of PLOS Blogs: blogs.plos.org.