September 4, 2018 report
Using AI to make cancer evolution more predictable
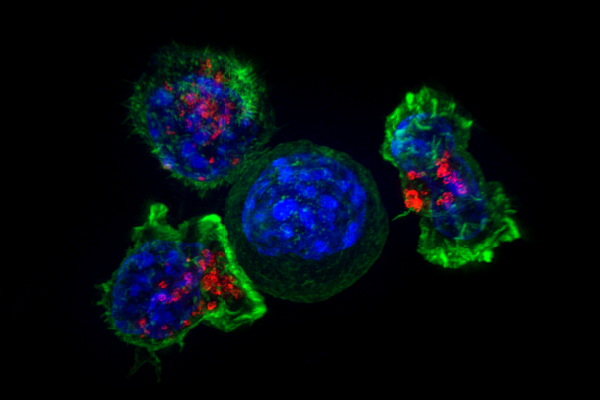
A team of researchers affiliated with several institutions in the U.K. and one in the U.S. has developed a way to use artificial intelligence to predict how cancer might change and spread in patients. The results are published in Nature Methods.
Over many years of cancer research, scientists have discovered that tumors actually evolve, allowing them to change their form and the way they spread. Understanding how this evolutionary process works is considered by many in the field to be a key part of learning how to prevent it from happening. As part of this effort, scientists have collected tissue samples from patients hoping to find a pattern in how they change. But this method has proven to be difficult, because when tumors grow, they also tend to develop mutations that have no impact on their ability to spread. In this new effort, the researchers sought to add machine learning to the process in an effort to track evolutionary changes that are involved in spreading. They have named their new system Revolver.
The new application uses a machine learning algorithm to study mutation data and detect patterns. They fed their system data describing 768 tumors from 178 patients—each of whom had breast, kidney, bowel or lung cancer. The system sought mutation patterns between patients that appeared to be related to changes that allowed the tumor to spread. Next, they applied what the system had learned to new patients as a way to assess the state of newly developing tumors—it was correctly identified gene mutations in 95 colorectal patients who had mutations that had been previously identified as drivers of evolution in breast, kidney and lung cancers.
The researchers note that Revolver is just one of the first steps toward developing computer-based tools to better predict how tumors will evolve—such tools should make it easier for doctors to formulate the best treatment plan for a given patient, hopefully, improving their prognosis.
More information: Giulio Caravagna et al. Detecting repeated cancer evolution from multi-region tumor sequencing data, Nature Methods (2018). DOI: 10.1038/s41592-018-0108-x
Abstract
Recurrent successions of genomic changes, both within and between patients, reflect repeated evolutionary processes that are valuable for the anticipation of cancer progression. Multi-region sequencing allows the temporal order of some genomic changes in a tumor to be inferred, but the robust identification of repeated evolution across patients remains a challenge. We developed a machine-learning method based on transfer learning that allowed us to overcome the stochastic effects of cancer evolution and noise in data and identified hidden evolutionary patterns in cancer cohorts. When applied to multi-region sequencing datasets from lung, breast, renal, and colorectal cancer (768 samples from 178 patients), our method detected repeated evolutionary trajectories in subgroups of patients, which were reproduced in single-sample cohorts (n = 2,935). Our method provides a means of classifying patients on the basis of how their tumor evolved, with implications for the anticipation of disease progression.
© 2018 Medical Xpress