AI predicts efficacy of breast cancer treatment directly from tumor architecture
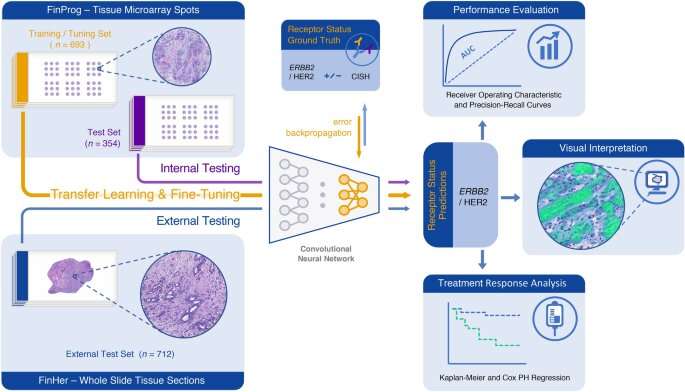
Researchers from the University of Helsinki have demonstrated the possibilities of artificial intelligence-based algorithms in predicting the efficacy of a targeted cancer therapy based on the tumor tissue architecture only, without specific molecular tests. The results suggest that AI can reveal previously hidden patterns in tumor samples and allow discovery of novel tumor features predictive of outcome and efficacy of treatment.
Artificial intelligence (AI) in the form of machine learning is increasingly used in cancer research and holds great potential in support of medical diagnostics. Algorithms have already been trained to tackle many complicated tasks such as detection of cancerous tissue and tumor grading. Also, prediction of disease outcome directly from a tumor sample without expert interpretation has shown promising results.
In the study published in Scientific Reports on Feb 17, a team led by Professor Johan Lundin aimed to push the capabilities of these approaches even further.
The researchers focused on developing a tool that could detect tumor morphological features typical for ERBB2-positive breast cancer. ERBB2 (also frequently called HER2) is a well-known oncoprotein that promotes the growth of cancer cells. Approximately every fifth breast cancer patient has extra copies of the ERBB2 gene and their tumors overexpress the ERBB2 protein. These patients can benefit from therapy with monoclonal antibodies against the ERBB2 (HER2) receptor.
The results of the study showed that the AI-algorithm was able to learn patterns predictive of the ERBB2 status of a tumor directly from the tumor morphology in a nationwide series of patients with breast cancer (the FinProg Study), without the use of specific molecular assays.
"Our results show that morphological features of tumors contain vast information about the biology of the disease that can be extracted with machine learning methods. This valuable data can aid in clinical decision-making," said the first author of the study, Dmitrii Bychkov from the Institute for Molecular Medicine Finland FIMM, University of Helsinki.
To test the applicability of the method further, the researchers next applied the AI-algorithm to tissue samples from breast cancer patients that had participated in a large clinical trial (the FinHer trial) on anti-ERBB2 treatment and whose ERBB2 status and outcomes were known.
Interestingly, the algorithm was able to discriminate the patients treated with anti-ERBB2 therapy (trastuzumab), a targeted treatment for ERBB2-positive cancers, into two prognostically different groups.Those patients in whose tumors the AI-algorithm predicted to be ERBB2-positive based on tumor morphology were shown to have a more favorable disease outcome than those predicted by the AI to be ERBB2 negative.
"These AI-based methods open up new opportunities to reveal patterns hidden in the tissue architecture that drive tumor progression and can in a longer perspective contribute to more precise diagnostics and better personalized treatment decisions in breast cancer," says MD, Associate Professor Nina Linder, who co-supervised the study.
The observations of the study also suggest that some of the tumors that were ERBB2-negative according to molecular tests have morphological features typical for ERBB2-positive tumors. According to the researchers, these patients might potentially benefit from treatments tailored for ERBB2-positive patients.
"The AI-based methods might not only complement the current molecular diagnostic methods but might go even beyond and lead to improved selection of some targeted cancer treatments for patients. We may need to design clinical trials to test this hypothesis. Importantly, the assay can be done from standard tumor section," says Professor Heikki Joensuu from the HUS Comprehensive Cancer Center and University of Helsinki who co-authored the study.
"This is one of the very first studies showing that AI applied to tumor samples can not only predict outcome of the disease, but also the efficacy or a molecularly targeted cancer treatment," said Professor Johan Lundin.
More information: Deep learning identifies morphological features in breast cancer predictive of cancer ERBB2 status and trastuzumab treatment efficacy. Sci Rep 11, 4037 (2021). doi.org/10.1038/s41598-021-83102-6