This article has been reviewed according to Science X's editorial process and policies. Editors have highlighted the following attributes while ensuring the content's credibility:
fact-checked
peer-reviewed publication
trusted source
proofread
AI-driven mobile health algorithm uses phone camera to detect blood vessel oxygen levels
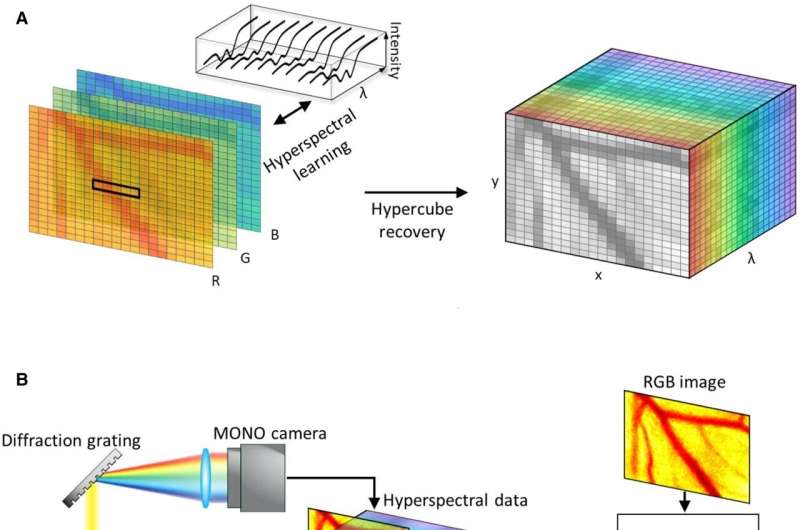
You may already use your smartphone for remote medical appointments. Why not use some of the onboard sensors to gather medical data? That's the idea behind AI-driven technology developed at Purdue University that could use a smartphone camera to detect and diagnose medical conditions like anemia faster and more accurately than highly specialized medical equipment being developed for the task.
"There are at least 15 different sensors in your smartphone, and our goal is to take advantage of those sensors so people can access health care outside of a doctor's office," said lead researcher Young Kim, professor and associate head for research in Purdue's Weldon School of Biomedical Engineering. "To the best of our knowledge, we believe that we demonstrated the fastest hemodynamic imaging in existence, using a commercially available smartphone."
While a smartphone camera is convenient, it captures measurements of only red, green and blue wavelengths of light in each pixel, limiting its medical utility. Hyperspectral imaging can capture all wavelengths of visible light in each pixel and could be used to detect a variety of skin and retinal conditions and some cancers.
Researchers are exploring hyperspectral imaging health care applications, but most of the work is aimed at improving specialized equipment, which is relatively bulky, slow and expensive. By pairing deep learning and statistical techniques with their knowledge of light-tissue interactions, Purdue researchers are able to reconstruct the full spectrum of visible light in each pixel of an ordinary smartphone camera image. The patent-pending approach, from a lab with expertise in mobile health, could improve access to health care.
As reported in PNAS Nexus, the team tested its method against commercially available hyperspectral imaging equipment when gathering information about the movement of blood oxygen in volunteers' eyelids, in models meant to mimic human tissue, and in a chick embryo.
Results show the smartphone camera produced hyperspectral information more quickly, more cheaply and just as accurately as those captured using specialized equipment. The smartphone approach can produce images in a single millisecond that would take conventional hyperspectral imaging three minutes to capture.
Kim said the work reported in PNAS Nexus focused on building the smartphone hyperspectral imaging algorithm rather than specific applications. But in other studies, the team has used its approach to measure blood hemoglobin for tissue oximetry and inflammation. Kim's lab used a computational approach that the researchers have dubbed "hyperspectral learning."
The process begins with a smartphone camera on an ultra-slow-motion setting that produces video at about 1,000 frames per second. Each pixel in each frame contains information for red, green and blue color intensity. The information is fed through a machine learning algorithm that infers full-spectrum information for each pixel.
That is used to produce the measurements of blood flow, particularly of the amount of oxygenated and deoxygenated hemoglobin in each pixel. These hemodynamic parameters can also be used to produce images and video that show oxygen saturation in their subjects over time.
As with conventional machine learning, the team trains its algorithms on a data set, feeding it smartphone images and the corresponding hyperspectral images and fine-tuning the algorithm until it can predict the correct relationship between the two data sets. But by building the algorithms with equations derived from tissue optics—an approach sometimes called "informed learning"—the researchers require a far smaller training data set.
And whereas conventional hyperspectral imaging equipment must gather massive amounts of data, limiting either the spectral resolution or temporal resolution, the team's approach begins with video files that are hundreds of times smaller than hyperspectral imaging files, allowing them to maintain a high standard on both fronts.
"Usually there's a trade-off to collect this information in an efficient manner. But with our approach, we have high spatial and spectral resolution at the same time," said Yuhyun Ji, first author and a graduate student in Kim's lab, which is currently working on applying this method to other mobile health applications, such as cervix colposcopy and retinal fundus imaging.
More information: Yuhyun Ji et al, mHealth hyperspectral learning for instantaneous spatiospectral imaging of hemodynamics, PNAS Nexus (2023). DOI: 10.1093/pnasnexus/pgad111