This article has been reviewed according to Science X's editorial process and policies. Editors have highlighted the following attributes while ensuring the content's credibility:
fact-checked
proofread
Pre-training in medical data: A survey
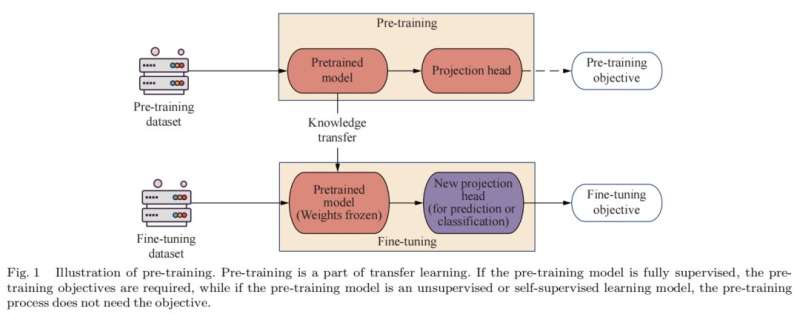
In a paper published in Machine Intelligence Research, a team of researchers summarizes a large number of related publications and the existing benchmarking in the medical domain. Notably, the survey briefly describes how some pre-training methods are applied to or developed for medical data.
From a data-driven perspective, the researchers examine the extensive use of pre-training in many medical scenarios. Moreover, based on the summary of recent pre-training studies, they identify several challenges in this field to provide insights for future studies.
Artificial intelligence (AI) has become a tremendously ubiquitous technique in the current world. Medical data analysis is one of the most important subfields in AI. The task mainly focuses on processing and analyzing the medical data from variant data modalities to extract essential information that aims to help physicians make precise decisions during the diagnosis process.
It is anticipated that computer-aided systems will be influential tools in health monitoring and disease diagnosis, and many related studies have achieved success. However, some works found that data scarcity is one of the primary challenges of applying the DNN for processing medical data. To deal with the problem, some researchers proposed the pre-training to address the issue of lack of annotated data. The pre-training technique is specially related to transfer and self-supervised learning.
Considering the fact that there are few systematic and comprehensive introductions to pre-training models and there is no comprehensive survey about pre-training in the medical domain, the researchers from the University of Queensland and the University of Adelaide aim to present a systematic introduction to recent advances and new frontiers of pre-training-based techniques in the medical domain.
They first briefly introduce the publicly available medical benchmark datasets and general pre-training strategies. Then, they investigate the extensive use of pre-training in different scenarios in the medical domain from four perspectives: images, bio-signal data, EHR data, and multi-modality data. At the end of this survey, they discuss the challenges and their possible solutions.
This paper provides a high-level introduction to benchmark datasets in the medical domain and representative pre-training strategies, as this paper focuses on pre-training in the medical domain, which will make the readers who are not specialized in pre-training techniques quickly and clearly learn about the developments of the related methods and the latest techniques.
As to the use of pre-training in the medical domain. Firstly, the main progress of medical images comes from the new field proposed by computer vision, and the impact of pre-training on traditional machine learning and deep learning is huge. Transfer learning and self-supervised learning solve the problem of image labeling and the problem of fewer data in pre-training, and the accuracy of pre-training segmentation and diagnosis can generally achieve more accurate results than traditional supervised learning.
Secondly, a summarization about recent studies that pre-train feature representations and use the pre-trained model on downstream tasks on bio-signal data is given. For bio-signals, a specific pre-training framework is required to explore to get further improvements in the performance. Thirdly, researchers summarize the recent advanced studies in pre-training on EHR data. There is no doubt that the transformer-based model is the mainstream for EHR data pre-training-related works.
The development of a privacy-related pre-training framework seems to be a promising topic in EHR studies. Fourthly, the paper gives an introduction of the multi-modality in pre-training in the medical domain.
Many researchers have tried to introduce pre-training to process the multimodality data. However, most of the current research only focuses on generating clinical reports and tries to use the model to interpret the radiological examination, and the main reason is that there are many large datasets for this task. In contrast, the lack of task-related datasets limits the progress of research on multi-modality pre-training.
Researchers point out that there remain some challenges that may hinder the development of high-performance model for medical tasks, such as data scarcity, privacy concerns and class imbalance. They also propose further development directions in the future study. More efforts are expected to be devoted to this field.
More information: Yixuan Qiu et al, Pre-training in Medical Data: A Survey, Machine Intelligence Research (2023). DOI: 10.1007/s11633-022-1382-8