This article has been reviewed according to Science X's editorial process and policies. Editors have highlighted the following attributes while ensuring the content's credibility:
fact-checked
trusted source
proofread
Promising heart drugs identified by cutting-edge combo of machine learning, human learning
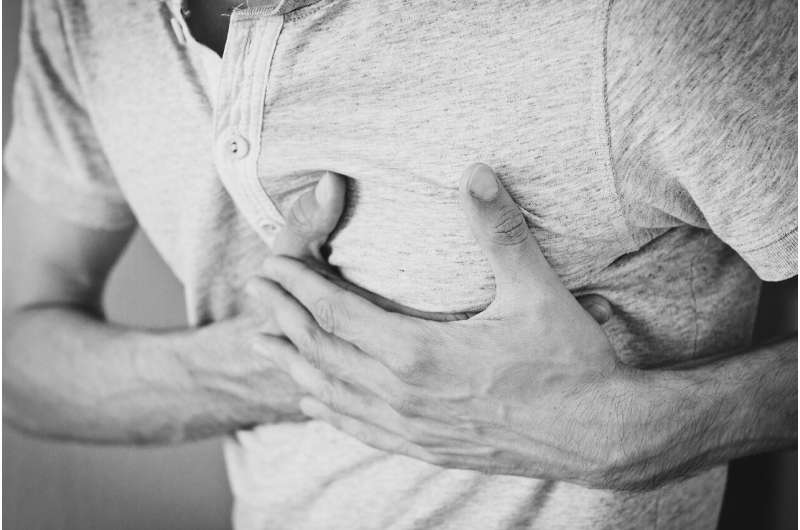
University of Virginia scientists have developed a new approach to machine learning—a form of artificial intelligence—to identify drugs that help minimize harmful scarring after a heart attack or other injuries. The findings are published in the journal Proceedings of the National Academy of Sciences.
The new machine-learning tool has already found a promising candidate to help prevent harmful heart scarring in a way distinct from previous drugs. The UVA researchers say their cutting-edge computer model has the potential to predict and explain the effects of drugs for other diseases as well.
"Many common diseases such as heart disease, metabolic disease and cancer are complex and hard to treat," said researcher Anders R. Nelson, Ph.D., a computational biologist and former student in the lab of UVA's Jeffrey J. Saucerman, Ph.D. "Machine learning helps us reduce this complexity, identify the most important factors that contribute to disease and better understand how drugs can modify diseased cells."
"On its own, machine learning helps us to identify cell signatures produced by drugs," said Saucerman, of UVA's Department of Biomedical Engineering, a joint program of the School of Medicine and School of Engineering.
"Bridging machine learning with human learning helped us not only predict drugs against fibrosis [scarring] but also explain how they work. This knowledge is needed to design clinical trials and identify potential side effects."
Combining machine learning, human learning
Saucerman and his team combined a computer model based on decades of human knowledge with machine learning to better understand how drugs affect cells called fibroblasts. These cells help repair the heart after injury by producing collagen and contract the wound. But they can also cause harmful scarring, called fibrosis, as part of the repair process.
Saucerman and his team wanted to see if a selection of promising drugs would give doctors more ability to prevent scarring and, ultimately, improve patient outcomes.
Previous attempts to identify drugs targeting fibroblasts have focused only on selected aspects of fibroblast behavior, and how these drugs work often remains unclear.
This knowledge gap has been a major challenge in developing targeted treatments for heart fibrosis. So Saucerman and his colleagues developed a new approach called "logic-based mechanistic machine learning" that not only predicts drugs but also predicts how they affect fibroblast behaviors.
They began by looking at the effect of 13 promising drugs on human fibroblasts, then used that data to train the machine learning model to predict the drugs' effects on the cells and how they behave.
The model was able to predict a new explanation of how the drug pirfenidone, already approved by the federal Food and Drug Administration for idiopathic pulmonary fibrosis, suppresses contractile fibers inside the fibroblast that stiffen the heart. The model also predicted how another type of contractile fiber could be targeted by the experimental Src inhibitor WH4023, which they experimentally validated with human cardiac fibroblasts.
Additional research is needed to verify the drugs work as intended in animal models and human patients, but the UVA researchers say their research suggests mechanistic machine learning represents a powerful tool for scientists seeking to discover biological cause-and-effect.
The new findings, they say, speak to the great potential the technology holds to advance the development of new treatments—not just for heart injury but for many diseases.
"We're looking forward to testing whether pirfenidone and WH4023 also suppress the fibroblast contraction of scars in preclinical animal models," Saucerman said.
"We hope this provides an example of how machine learning and human learning can work together to not only discover but also understand how new drugs work."
More information: Anders R. Nelson et al, Logic-based mechanistic machine learning on high-content images reveals how drugs differentially regulate cardiac fibroblasts, Proceedings of the National Academy of Sciences (2024). DOI: 10.1073/pnas.2303513121