This article has been reviewed according to Science X's editorial process and policies. Editors have highlighted the following attributes while ensuring the content's credibility:
fact-checked
proofread
Predictive model of oxaliplatin-induced liver injury based on artificial neural network and logistic regression
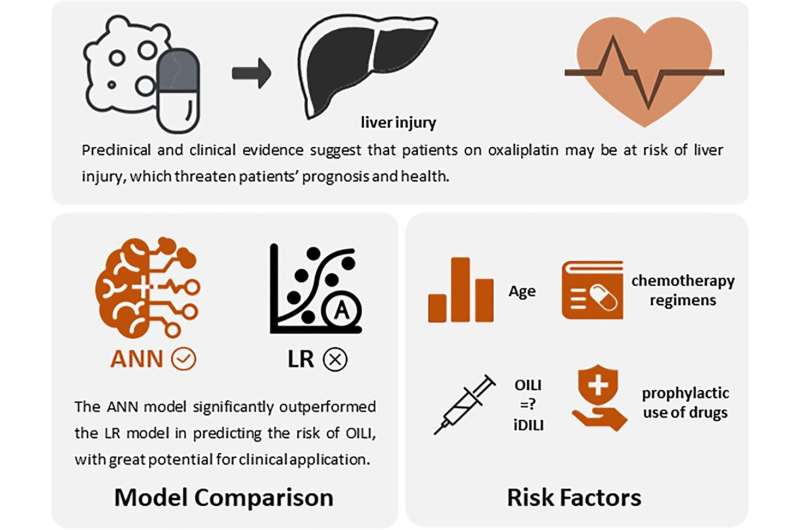
Since 2004, several clinical studies have reported that patients with OXA frequently experienced adverse effects of liver injury (LI), typically characterized by hepatic sinusoidal injury, splenomegaly, decreased platelet count, and noncirrhotic portal hypertension, which can progress to nodular regenerative hyperplasia with long-term treatment.
LI also decreases hepatic functional reserve and aggravates the postoperative course of colorectal cancer patients after hepatectomy, and may affect intraoperative bleeding, postoperative morbidity, and overall survival. LI can further progress to liver fibrosis and liver cirrhosis, both of which would be detrimental to the patients' health.
The risk of OXA-induced LI (OILI) can be greatly reduced if people potentially at high risk of OILI can be identified and then treated and prevented accordingly in advance.
Although there is a preliminary understanding of the clinical features and disease characteristics, studies on OILI's prediction or risk factors are rare. There is a lack of exploration in terms of patient and medication characteristics, and there is also a lack of clinical prediction tools for OILI. Based on a previous safety evaluation of OXA, OILI was explored in depth.
Artificial Neural Network(Ann) and logistic regression (LR) models were selected to predict the risk of OILI, and the performance of the two models was evaluated and compared, in the expectation of identifying patients at high risk of OILI, achieving timely intervention and appropriate management, and improving the safety of OXA administration.
The medical information of patients treated with oxaliplatin between May and November 2016 at 10 hospitals was collected prospectively. The researchers used the updated Roussel Uclaf causality assessment method (RUCAM) to identify cases of OILI and summarized the patient and medication characteristics. Furthermore, the ANN and LR models for predicting the risk of OILI were developed and evaluated.
The incidence of OILI was 3.65%. The median RUCAM score with interquartile range was 6 (4, 9). The ANN model performed similarly to the LR model in sensitivity, specificity, and accuracy. In discrimination, the area under the curve of the ANN model was larger (0.920>0.833, p=0.019). In calibration, the ANN model was slightly improved.
The important predictors of both models overlapped partially, including age, chemotherapy regimens and cycles, single and total dose of OXA, glucocorticoid drugs, and antihistamine drugs.
When discriminative and calibration abilities were given priority, the ANN model significantly outperformed the traditional LR model in predicting the risk of OILI. It. has great potential for clinical application. A comprehensive analysis found that age, chemotherapy regimens, prophylactic use of glucocorticoids, and prophylactic use of antihistamines were associated with the risk of OILI.
Other chemotherapy drugs in OXA-based chemotherapy regimens may have different degrees of impact on OILI and deserve further attention. The researchers suspect that OILI may be an iDILI, and chemotherapy dose factors may be weakly correlated. Decision-making on prophylactic medications needs to be carefully considered, and the actual preventive effect also needs to be supported by more evidence.
Based on the ANN model and potential risk factors, early screening of high-risk groups can be carried out. Medical decision-making can be optimized. Timely intervention and nursing can be realized. The ultimate goal is to reduce the adverse effects of OILI and promote drug safety in cancer patients.
The paper is published in the Journal of Clinical and Translational Hepatology.
More information: Rui Huang et al, Predictive Model of Oxaliplatin-induced Liver Injury Based on Artificial Neural Network and Logistic Regression, Journal of Clinical and Translational Hepatology (2023). DOI: 10.14218/JCTH.2023.00399