This article has been reviewed according to Science X's editorial process and policies. Editors have highlighted the following attributes while ensuring the content's credibility:
fact-checked
trusted source
proofread
Reliable emergency room translations might be job for humans, AI together
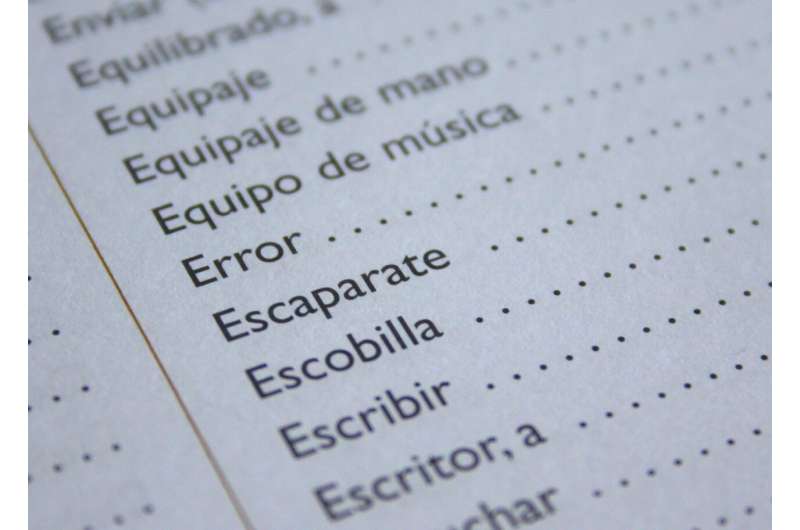
While the garbled translation of a newspaper article in a foreign language may be nothing more than an annoyance, uses of machine translation technology extend to higher-stakes settings as well: In a hospital emergency room, incorrectly translated discharge instructions or medication protocols could have life-threatening consequences.
Researchers from the University of Maryland's Computational Linguistics and Information Processing (CLIP) Lab looked into this problem, studying data collected from English-to-Chinese machine translation systems used in emergency rooms at the University of California, San Francisco.
The paper is published in the journal Proceedings of the 2023 Conference on Empirical Methods in Natural Language Processing
They found that neither an artificial intelligence tool to monitor translation quality nor more manual approaches could fully overcome errors—but that combining human and computerized abilities held promise for improving such systems.
For this study, the CLIP team reviewed data from 65 English-speaking physicians to evaluate two distinct methods for assessing the quality of machine-generated translations used for Chinese-speaking patients.
One group of physicians used a quality estimation tool—AI-driven software that can automatically predict the accuracy of a machine translation output. According to the researchers, this tool helped doctors rely on machine translation more appropriately by deciding to show "good" translations to patients overall. But the tool was not perfect; it failed to flag some critical errors that could harm the health of the patient.
A second set of doctors used a technique known as backtranslation, where the user retranslates the Chinese output using Google Translate to assess its English output. The researchers observed complementary trends for these doctors: backtranslation does not improve their ability to assess translation quality on average, but does help identify clinically critical errors that quality estimation tools fail to flag.
The CLIP team believes its study paves the way for future work in designing methods that combine the strengths of both methods tested, resulting in a human-centered evaluation design that can be used to further improve machine translation tools used in clinical settings.
"Our study confirms that lay users often trust AI systems even when they should not, and that the strategies that people develop on their own to decide whether to trust an output—such as backtranslation—can be misleading," said Marine Carpuat, an associate professor of computer science who co-authored the study.
"However, we show that AI techniques can also be used to provide feedback that helps people calibrate their trust in systems. We view this as a first step toward developing trustworthy AI."
Sweta Agrawal Ph.D. '23, a co-author on the study who is now a postdoctoral fellow at the Instituto de Telecomunicações in Portugal, said that the project has important implications for medical care and society at large.
"This work provides support for the usefulness of providing actionable feedback to users in high-risk scenarios," she said. "Moreover, the findings contribute to the ongoing research efforts to design reliable metrics, especially for critical domains like health care."
Other UMD co-authors included Ge Gao, an assistant professor of information studies and Yimin Xiao, a third-year information studies doctoral student; researchers from the University of California (UC) Berkeley, and UC San Francisco also numbered among the co-authors.
Carpuat and Gao both have appointments in the University of Maryland Institute for Advanced Computer Studies, which provides technical and administrative support for their work in the CLIP Lab.
Based on their findings, the researchers will develop new techniques to assist people in using these imperfect systems more effectively.
More information: Nikita Mehandru et al, Physician Detection of Clinical Harm in Machine Translation: Quality Estimation Aids in Reliance and Backtranslation Identifies Critical Errors, Proceedings of the 2023 Conference on Empirical Methods in Natural Language Processing (2023). DOI: 10.18653/v1/2023.emnlp-main.712