February 23, 2017 report
Digital pathology platforms can now determine the metastatic potential of cells in a tissue biopsy (Update)
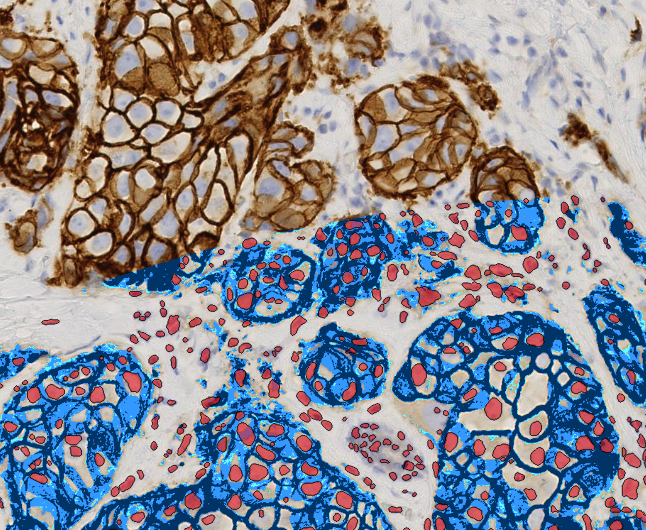
(Medical Xpress)—One major challenge in pathology is to determine if a group of cells are cancerous. By 'cancerous' one generally means that they have the potential to grow and spread, or that they have already spread from somewhere else and are therefore metastatic. Cancer cells tend to spread to other organs via the bloodstream. When they do this, they inevitably also make their way to the lymphatic system. By checking the so-called 'sentinel' lymph nodes—those nodes adjacent to a primary tumor—doctors can get a good idea of whether or not a cancer has gone rogue.
The problem is that the manual analysis of a lymph biopsy by a pathologist is not only tedious, but also fairly subjective. Furthermore, as cancer treatment regimens evolve and become more elaborate, the demand for expert tissue analysis continues to outstrip the supply of expert pathologists. While the trained human eye is and will continue to be the gold standard, technological solutions to help alleviate this burden are highly sought. A company called Proscia now has a cloud based digital pathology platform that has proven itself in classifying breast cancer metastasis.
One immediate goal is to come up with a solution for the accurate prediction of lymph node metastasis originating from primary lung tumors. This is critical for selecting which treatments, like endoscopic resection for example, will be optimal for PATIENTS. The lung cancers are generally classified as either small cell or non small cell tumors. Unfortunately, neither type has as a clear 'marker' gene or protein associated with it that would indicate metastatic potential or response to treatments.
By contrast, many other common cancers associated with various organs like breast, pancreatic, or colon cancer, secrete proteins which directly correlate to susceptibility certain drugs, probability of recurrence, or likely success of tissue resection. For example 'cancer antigen' CA125 in ovarian cancer, or CD1D in pancreatic cancer. These markers should not be confused with those genes or proteins used merely for screening purposes, such as prostate cancer PSA or breast cancer BRCA.
In the absence of a convenient marker, the only way to try to gauge lung cancer metastases from a lymph biopsy is to look at the raw tissue. Typically, tissue samples are histologically prepared with the hematoxylin and eosin stain to increase contrast and visibility. This labels the nucleus a blue/violet and the cytoplasmic structures a pink/red. In some cancers this enough to spot the hallmark topological rearrangement of cells indicative of a particular cancer. For lung cancers however, pathologists have found no tangible histological variables that stand up to scrutiny.
This is where Proscia's deep/machine learning (essentially convolutional neural networks) algorithms excel. Things like the size, shape, and distribution of organelles and whole cells are perturbed in the altered metabolisms driving cancer cells. While not always definable by the human eye, they can nonetheless often be classified by machines. Automated methods can be calibrated for variability in the staining from day-to-day and lab-to-lab. By default, they also eliminate any human subjectivity in the metastasis prediction itself.
To better see what it is these networks are actually doing briefly consider the retina. Its primary job is to compress the visual scene for package on the optic nerve. The front end of any image analysis system is the place where you want to compensate for changes in light level. The retina does this on different time and spatial scales by a combination of shutters, biochemical adaptations in photoreceptors, and local rewiring of retinal connections. On top of this, the retina also throws in a fair bit of feature extraction for things like moving bars and edges. It does this by combining just a few layers of cells extracting detail with weighted center-surround receptive fields. These features are further refined at each higher level in the visual system, perhaps most famously in the so-called 'simple cells' of the primary visual cortex which respond favorably to oriented edges and gratings.
Somewhat analogously, Proscia's methods involve a similar image preprocessing steps that include stain renormalization and color deconvolution. The actual discrimination between normal tissue and tumor is then done by a deep learning convolutional network. As an example, a typical architecture used might consist of five convolutional layers followed by three fully connected learning layers which ultimately classify the output. There are likely also so-called polling network layers which effectively subdivide each region into smaller blocks of pixels where only the highest value pixel is 'polled' and transmitted to the next layer. Ultimately the slide image is converted into a tumor probability heatmap where each pixel is assigned a color value that indicates the probability of metastasis.
The convolution layers are one place where the networks can be tweaked to learn which kinds of patterns are good and which are bad. In this context, a convolution is basically a filter that replaces each pixel with a specially weighted combination of its nearby neighbors. Depending on how many neighbors are involved in the operation (the so-called 'stride' of the operation), and how the filter matrix is weighted, different kinds of averages or detection of edges and features can be obtained. The whole network gets trained using slides of tissues that have been correctly classified by real pathologists. They are then evaluated by presenting additional images for classification.
While the sensitivity, specificity and error rates of these networks may provide fodder for the FDA to chew on for some time going forward, there are already some positive signs that these kinds of deep learning techniques are going mainstream. One example is a new medical imaging deep learning network from Arterys was recently approved by the FDA for diagnosing heart issue. Another artifical intelligence application in this vein is IBM's Watson Health computer which can scan published clinical trial reports and classify patients for acceptance.
I recently spoke with CEO David West Jr. and technical expert Hunter Jackson to get a picture of where things are headed at Proscia. They indicated to me that their diagnostic algorithms are in development and they anticipate entering the clinical marker having gone through some regulatory process. Proscia also provides essential cloud services which complement these deep learning methods. For example, the volume of the data alone in digital pathology can be staggering. With patients having up to 12 or so 5GB images, a single hospital lab must manage terabytes of data per month. Proscia can now organize this volume of data so pathologists can view images on a case-by-case basis rather than just a single image from a single patient.
For the time being, pathology labs deal with two-dimensional slide sections of tumor. To get a further glimpse of where new technology may be heading in practical medicine it is often useful to look at what cutting edge biology labs are doing now. Extracting and analyzing cellular and subcellular profiles has already been taken to extreme form in neuroscience where the full 3-D picture can now be obtained. To get it, neuroscientists slice and dice tiny grains of cortex, etch it away layer-by-layer with a fancy gallium ion beam, scan it using an electron microscope, and then fully reconstruct the membrane topology and organelle distribution in silico.
Where a terabyte may now represent a hospital's path lab metastatic tissue sample output, it only represents a millimeter scale cube of brain data captured in this way. Within that cube, not just the nucleus, but also each mitochondria and secretory vesicle can be captured and analyzed. In the stressed and heterogeneous metabolisms now found in any given tumor researchers are slowing beginning to be able to associate unique states and functions to the fine details of cells and organelles. Analyzing this exploding minutia is probably not the best way to employ trained pathologists, but may make an excellent pastime for machines.
© 2017 Medical Xpress