Sports playbook helps doctors predict cancer patient outcomes
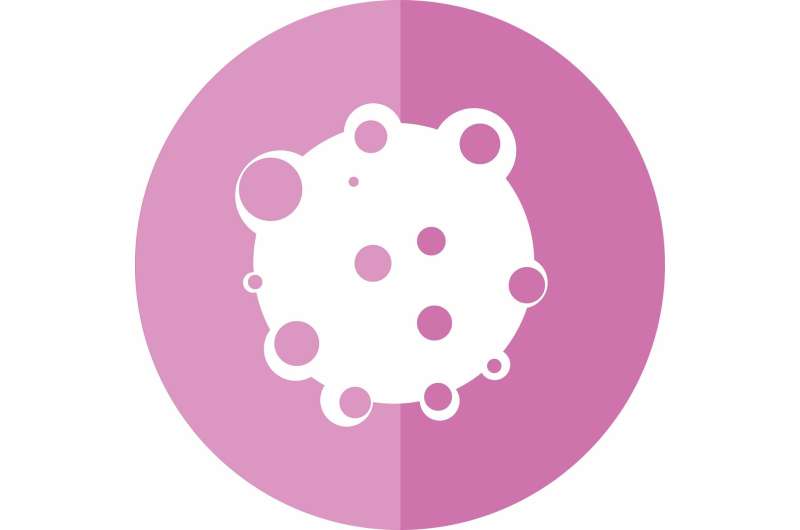
In this season of global soccer competitions and hotly contested political primaries, bookies and pundits are scouring every evolving scrap of information and sifting through mountains of data in an effort to predict the outcome of the next game or election. These predictions can change on a dime, however, based on a player's poor pass or a candidate's stellar debate performance.
Statisticians refer to the technique of incorporating a variety of continuously generated information—who is on the bench, who was injured in the first half of the match, who polled well in Iowa yesterday—as calculating in-game win probability, and it's been used for decades to predict the outcome of ongoing sports matches or elections.
Now researchers at the Stanford University School of Medicine have taken a page from this playbook to generate more accurate prognoses for cancer patients. They've done so by designing a computer algorithm that can integrate many different types of predictive data—including a tumor's response to treatment and the amount of cancer DNA circulating in a patient's blood during therapy—to generate a single, dynamic risk assessment at any point in time during a patient's course of treatment. Such an advance could be deeply meaningful for patients and their doctors.
"When we care for our patients, we are walking on eggshells for a profound period of time while we try to determine whether the cancer is truly gone, or if it is likely to return," said associate professor of medicine Ash Alizadeh, MD, Ph.D. "And patients are wondering 'Should I be planning to attend my child's wedding next summer, or should I prioritize making my will?' We are trying to come up with a better way to predict at any point during a patient's course of treatment what their outcome is likely to be."
Surprisingly, the researchers have also found that the approach, which they've termed CIRI for Continuous Individualized Risk Index, may also help doctors to pinpoint people who might benefit from early, more aggressive treatments as well as those who are likely to be cured by standard methods.
The study will be published online July 4 in Cell. Alizadeh, a Stanford Health Care oncologist who specializes in treating patients with blood cancers, shares senior authorship with associate professor of radiation oncology Maxmilian Diehn, MD, Ph.D. Instructor of medicine David Kurtz, MD, Ph.D., and postdoctoral scholars Mohammad Esfahani, Ph.D., and Florian Scherer, MD, are the lead authors.
Getting a more complete picture
The researchers began their study by looking at people previously diagnosed with diffuse large B-cell lymphoma, which is the most common blood cancer in the United States. Although nearly two-thirds of adults with DLBCL are cured with standard treatment protocols, the remaining third will likely die from the disease.
When a DLBCL patient is diagnosed, clinicians like Alizadeh, Diehn and Kurtz assess the initial symptoms, the cell type from which the cancer originated and the size and location of the tumor after the first imaging scan to generate an initial prognosis. More recently, clinicians have also been able to assess the amount of tumor DNA circulating in a patient's blood after the first one or two rounds of therapy to determine how the tumor is responding and estimate a patient's overall risk of succumbing to their disease.
But each of these situations gives a risk based on a snapshot in time rather than aggregating all the data available to generate a single, dynamic risk assessment that can be updated throughout the course of a patient's treatment.
"What we're doing now is somewhat like trying to predict the outcome of a basketball game by tuning in at halftime to check the score, or by watching only the tipoff," Diehn said, "when in reality we know that there are any number of things that could have happened during the first half that we aren't taking into account. We wanted to learn if it's best to look at the latest information available about a patient, the earliest information we gathered, or whether it's best to aggregate all of this data over many time points."
Alizadeh and his colleagues gathered data on more than 2,500 DLBCL patients from 11 previously published studies for whom the three most common predictors of prognosis were available. They used the data to train a computer algorithm to recognize patterns and combinations likely to affect whether a patient lived for at least 24 months after seemingly successful treatment without experiencing a recurrence of their disease. They also included information from 132 patients for whom data about circulating tumor DNA levels were available prior to and after the first and second rounds of treatment.
"Our standard methods of predicting prognoses in these patients are not that accurate," Kurtz said. "Using standard baseline variables it becomes almost a crystal ball exercise. If a perfectly accurate test has a score of 1, and a test that assigns patients randomly to one of two groups has a score of 0.5—essentially a coin toss—our current methods score at about 0.6. But CIRI's score was around 0.8. Not perfect, but markedly better than we've done in the past."
Identifying better treatment options
The researchers next tested CIRI's performance on data from previously published panels of people with a common leukemia and another on breast cancer patients. Although the prognostic indicators varied for each disease, they found that, by serially integrating the predictive information over time, CIRI outperformed the standard methods. Furthermore, it suggested that it might be useful to identify patients who might need more aggressive intervention within one or two rounds of treatment rather than waiting to see if the disease recurs.
"What I didn't expect was that aggregating all this information through time may also be predictive," Alizadeh said. "It might tell us 'you're going down the wrong path with this therapy, and this other therapy might be better.' Now we have a mathematical model that might help us identify subsets of patients who are unlikely to do well with standard treatments."
The researchers are next planning to test CIRI's predictive capabilities in people recently diagnosed with aggressive lymphoma.