New AI model learns from thousands of possibilities to suggest medical diagnoses and tests
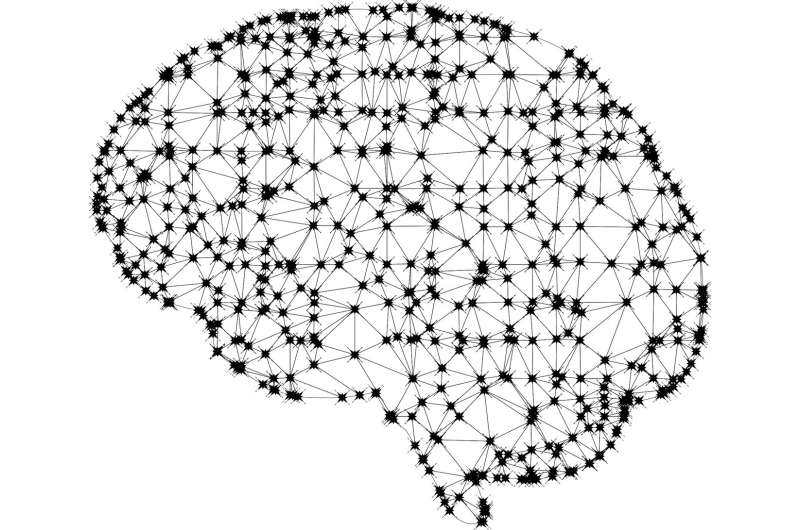
AI has, for some time, been applied to diagnose medical conditions in specific fields. It can build on knowledge of particular disciplines to hone in on details such as the shape of a tumor that suggests breast cancer or abnormal cells that indicate cervical cancer. While AI is very good when trained on years of human data in specific domains, it has not been able to deal with the huge number of diagnostic tests (about 5000) and disorders (about 14,000) of modern clinical practice. Now, a new algorithm developed by engineers at the USC Viterbi School of Engineering can think and learn just like a doctor but with essentially infinite experience.
The work comes out of the lab of Gerald Loeb, a professor of biomedical engineering, pharmacy and neurology at USC Viterbi School of Engineering and a trained physician. Loeb spent years applying AI algorithms to haptics and building robots to sense and identify materials and objects. His previous research on this surpassed the state of the art. While the state of AI for haptics was to identify about 10 objects with about 80 percent accuracy, Loeb and Jeremy Fishel, his graduate student at the time, were able to identify 117 objects with 95 percent accuracy. When they extended it to 500 objects and 15 different possible tests, their algorithm got even faster and more accurate. That, Loeb says, is when he started thinking about adapting it for medical diagnosis.
Loeb's new form of AI suggests the best diagnostic strategies by mining electronic healthcare records in databases. This could lead to faster, better and more efficient diagnoses and treatments. The work was published in the Journal of Biomedical Informatics.
The algorithm works just like a doctor- "thinking about what to do next at each stage of the medical work-up," said Loeb, a pioneer in the field of neural prosthetics and one of the original developers of the cochlear implant, now widely used to treat hearing loss. "The difference is that it has the benefit of all the experiences in the collective healthcare records."
How it Works
Conventional AI has long used a specific algorithm to suggest to physicians the most likely diagnoses given a set of observations. Called Bayesian Inference, it uses whatever information is currently available to suggest which diagnoses are the most likely.
Loeb's algorithm reverses this process and instead seeks those tests that would most likely identify the correct illness or condition, no matter how obscure. He calls this Bayesian Exploration. The algorithm can also take into account the costs and delays associated with various diagnostic tests.
"This hasn't been done before," he said. "This is new."
Loeb said his new algorithm has several benefits.First, this algorithm could help doctors make better diagnostic and testing decisions by suggesting several good options, including some a practitioner might not have otherwise considered. Next, the diagnostic software would automatically update and improve, as myriad physicians input additional data into electronic medical records.
In addition, Loeb believes doctors would more easily generate complete and accurate medical records. Instead of having to hunt for codes or work their way through many drop-down menus, they could simply select a particular illness or diagnostic procedure suggested by the AI, which would automatically input the correct information into the electronic records.
Loeb emphasizes that physicians could, of course, override the AI and go with their own judgment.
"The algorithm isn't meant to make decisions for doctors or replace them," Loeb said. "It's meant to complement and support them."
Looking to the future
Loeb believes this algorithm could revolutionize medical and testing diagnostics. But the USC Viterbi and Keck School of Medicine professor acknowledges the huge financial and technological challenges of applying AI to electronic health records. The United States' balkanized medical system and spotty usage of electronic medical records he believes make it an inhospitable environment for his technology to take root.
Loeb says his system would be much easier to introduce in other countries, for example in Scandinavia or in South Korea, places with nationalized healthcare and the widespread usage of electronic medical records. However, its implementation would face major challenges even there, including the large expense and brainpower needed to develop and deploy the massive database and user interfaces for the widespread adoption and integration of his algorithm.
Instead, Loeb puts his faith in tech. He believes that Amazon, Microsoft and Google have the resources and know-how to disrupt American healthcare the way Uber and Lyft upended the taxicab industry.
"If the promise of success is great enough, then people are going to be motivated to do it," Loeb said. "And that's what we think this algorithm provides: the possibility, the promise of offering a solution to a huge problem that wastes a lot of resources, trillions of dollars' worth."
More information: Gerald E. Loeb. A New Approach to Medical Diagnostic Decision Support, Journal of Biomedical Informatics (2021). DOI: 10.1016/j.jbi.2021.103723