This article has been reviewed according to Science X's editorial process and policies. Editors have highlighted the following attributes while ensuring the content's credibility:
fact-checked
proofread
Using medical knowledge graphs in smart applications for clinical diagnoses and more
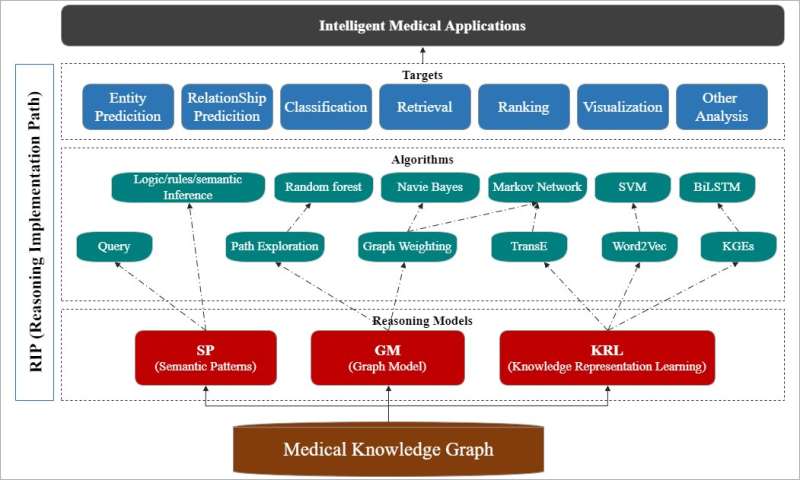
A medical knowledge graph is a specific way that researchers can organize and display information for use in medical research and clinical applications. The concept of a knowledge graph was originally developed by Google in 2012 as part of their search algorithm.
Since then, medical knowledge graphs have been shown to have a wide range of utility in the medical and medical research fields. For example, a knowledge graph could be created based on data pulled from electronic medical records, clinical trials, or other medical literature. When that information is plugged into a computer system, the medical knowledge graph can be the backbone of intelligent applications, such as chatbots, diagnosis and treatment plan recommendations, and clinical education.
In a paper published in Big Data Mining and Analytics on January 26, researchers set out to provide a comprehensive catalog of medical knowledge graphs, how they are created, and when they should be used.
"Medical knowledge graphs are the basis for intelligent health care, and they have been in use in a variety of medical applications," said Min Li, a professor and dean at the School of Computer Science at Central South University in Changsha, China.
"Understanding the research and application development of medical knowledge graphs will be crucial for future relevant research in the biomedical field. Our research mainly demonstrates the progress of medical knowledge graphs, including data sources, construction methods, reasoning methods, and applications."
One of the most important factors in how reliable and trustworthy a medical knowledge graph is is its data source. With an increase in the use of electronic medical records, online databases of clinical trial data, and more, it is possible to create extensive medical knowledge graphs. Researchers outline four potential sources for data, including real-world data acquired from services like electronic medical records and other available clinical data; scientific publications, such as journals, textbooks, guidelines, standard libraries, and open-and-shared medical knowledge databases, which are databases that are free, open, and accessible.
Researchers also analyze when to use knowledge graphs and different ways to organize the data. They identify disease diagnosis as an upcoming research hotspot, where researchers are using knowledge graphs to identify diseases and provide predictive results. They also note that medical knowledge graphs can be useful in developing drugs and research is underway to determine how to use these tools to develop COVID-19 drugs.
"Our research will hopefully allow readers to understand the research value, main research purpose, and the progress and challenges of medical knowledge graphs, which will help researchers in related fields look closely at these tools," said Li.
Looking ahead, researchers note the different challenges associated with medical knowledge graphs. One challenge is pulling data from disparate sources and making sure that it is cohesive and unified so that it can be read by the application. Another is that the medical domain is incredibly complex. Researchers going forward will need to continue to study how medical knowledge graphs can account for this complexity, especially when it comes to clinical diagnoses and therapies.
Another challenge is that as different medical knowledge graphs are created, they are all created using different frameworks, terminology, and technology. Finally, they posit that this type of knowledge graph will need to also incorporate common sense knowledge, in addition to specialized medical knowledge.
"The next step will focus on in-depth research on the construction and reasoning methods of medical knowledge graphs," said Li. "The ultimate goal is to build a large-scale and high-quality medical knowledge graph and propose more novel reasoning methods and applications in precision medicine."
More information: Xuehong Wu et al, Medical Knowledge Graph: Data Sources, Construction, Reasoning, and Applications, Big Data Mining and Analytics (2023). DOI: 10.26599/BDMA.2022.9020021