This article has been reviewed according to Science X's editorial process and policies. Editors have highlighted the following attributes while ensuring the content's credibility:
fact-checked
trusted source
proofread
AI and genetics could help doctors treat alcohol addiction, research shows
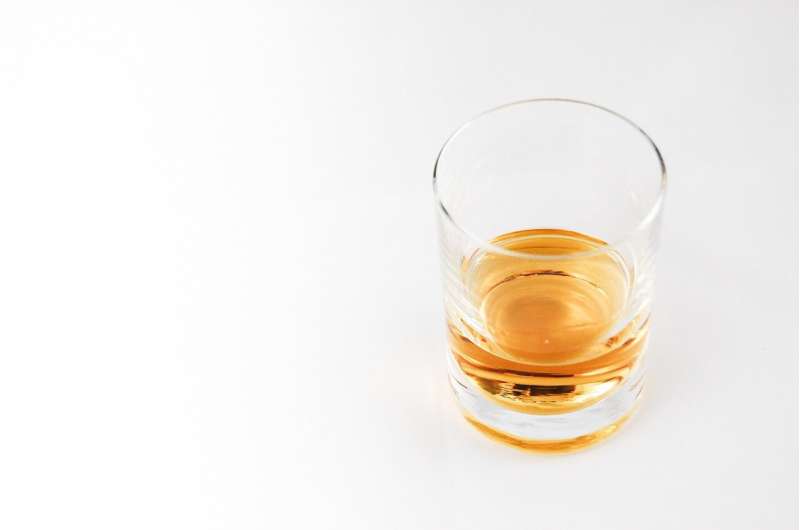
Imagine a patient has been diagnosed with alcohol use disorder, and their health care provider is reviewing medication options to help them curb their drinking. The provider asks the patient some basic questions, like alcohol cravings and stress levels, and collects a blood sample for genetic testing. A computer model uses this information to determine which medication would most likely support the patient with managing their alcohol use. With the help of the model, the provider gives a medication recommendation that is the best fit for their patient.
That is a possibility that Albert Arias, M.D., and his research team at Virginia Commonwealth University are trying to help make a reality.
For patients with alcohol-related health problems, abstinence is one of the most important interventions for reversing these issues or preventing them from becoming worse. A number of medications can help reduce a patient's desire to drink, though success varies between patients.
"How a patient responds to a particular drug is the result of many factors, including their genes, behaviors and environment. Some patients have a powerful response to a medication, and some patients have no response at all," said Arias, who is an associate professor in the VCU School of Medicine's Department of Psychiatry. "And while we think about risks, side effects, and other factors when considering medications for patients, we don't have a good way yet to specifically match a treatment to a person."
To address this issue, Arias and his colleagues are using artificial intelligence to better understand which individual factors can be used to predict how patients with alcohol use disorder respond to certain medications. He presented the results last fall at the inaugural academic symposium of the Stravitz-Sanyal Institute for Liver Disease and Metabolic Health at VCU, as well as the Research Society on Alcoholism's Annual Scientific Meeting.
For this study, the researchers analyzed data from a clinical trial in which participants who regularly drank heavily were either given a placebo or zonisamide, a drug that has shown promise with helping patients overcome alcohol addiction. They created predictive models to determine which combination of individual traits were most associated with fewer days spent drinking heavily following zonisamide treatment. The traits used to generate the models included alcohol cravings, anxiety, depression, mood states and genetic profiles associated with alcohol use.
The model proved to be accurate in determining which people would likely benefit from zonisamide. Among the patients predicted to respond well to the drug, those given zonisamide had better health outcomes than those given the placebo. Genetics was the most important factor for predicting treatment response, but the researchers also found that even without the genetic profiles, the models were still able to provide strong predictions.
"We're many years away from being able to map a single person's genetic profile rapidly, but we found that even basic clinical information could be used to calculate the likelihood of response to a certain medication for alcohol use disorder," Arias said.
Coming together to fight alcoholic and non-alcoholic liver disease
Up to 35% of heavy drinkers are estimated to develop alcohol-related liver disease, also known as alcoholic hepatitis, in their lifetime. The liver is responsible for breaking down alcohol. If a person drinks more alcohol than the liver can handle, the organ experiences inflammation. This kind of damage can also occur when excessive fat builds up in the liver. Over time, the liver can become covered with scars from chronic inflammation, which interferes with liver function and can ultimately lead to liver failure.
Alcoholic and nonalcoholic liver disease were some of the issues researchers, like Arias, shared insights into during the Stravitz-Sanyal Institute for Liver Disease and Metabolic Health's inaugural symposium.
"There has been a progressive increase in the number of deaths from alcoholic hepatitis in the United States, linked to the increase in alcohol consumption," said Arun J. Sanyal, M.D., director of the Stravitz-Sanyal Institute for Liver Disease and Metabolic Health.
As part of the symposium, experts discussed new discoveries, scientific trends and areas of opportunity for improving outcomes for patients navigating liver health problems.
For example, early research has shown that transplanting a healthy mix of gut microbes into patients with alcohol use disorder and liver disease could help with managing cravings and limiting alcohol intake. Researchers also shared results from investigating extracellular vesicles, small membrane-bound spheres that could potentially serve as biomarkers for detecting early stages of liver disease, as well as act as a natural delivery mechanism for therapeutics. Additionally, advances have been made in identifying therapies for liver regeneration.
"Our research community has the potential for doing amazing things that will be transformative for our patients," Sanyal said. "We need to continue to bring the power of medicine, technology, engineering, sociology, economics, business, government, communications and justice to address different facets of this problem of liver disease and a larger problem of metabolic health."