This article has been reviewed according to Science X's editorial process and policies. Editors have highlighted the following attributes while ensuring the content's credibility:
fact-checked
peer-reviewed publication
trusted source
proofread
Researchers apply artificial intelligence for early risk forecasting of Alzheimer's disease
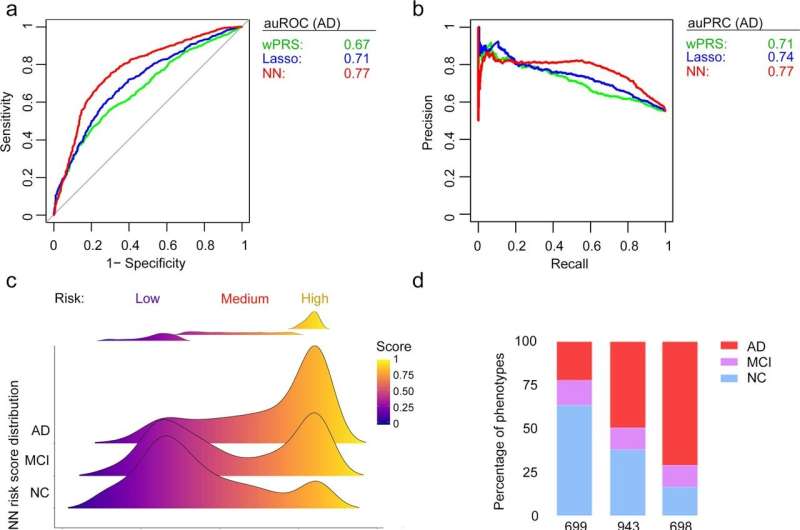
An international research team led by the Hong Kong University of Science and Technology (HKUST) has developed an artificial intelligence (AI)-based model that uses genetic information to predict an individual's risk of developing Alzheimer's disease (AD) well before symptoms occur. This study paves the way for using deep learning methods to predict the risks of diseases and uncover their molecular mechanisms; this could revolutionize the diagnosis of, interventions for, and clinical research on AD and other common diseases such as cardiovascular diseases.
Researchers led by HKUST's President, Prof. Nancy Ip, in collaboration with the Chair Professor and Director of HKUST's Big Data Institute, Prof. Chen Lei, investigated whether AI—specifically deep learning models—can model AD risk using genetic information. The team established one of the first deep learning models for estimating AD polygenic risks in both European-descent and Chinese populations. Compared to other models, these deep learning models more accurately classify patients with AD and stratify individuals into distinct groups based on disease risks associated with alterations of various biological processes.
In current daily practice, AD is diagnosed clinically, using various means including cognitive tests and brain imaging, but often when patients are showing symptoms, it is already well past the optimal intervention window. Therefore, early forecasting of AD risk can greatly aid diagnosis and the development of intervention strategies. By combining the new deep learning model with genetic testing, an individual's lifetime risk of developing AD can be estimated with more than 70% accuracy.
AD is a heritable disorder that can be attributed to genomic variants. As these variants are present from birth and remain constant throughout life, examining an individual's DNA information can help predict their relative risk of developing AD, thereby enabling early intervention and timely management. While FDA-approved genetic testing for the APOE-ε4 genetic variant can estimate AD risk, it may be insufficient to identify high-risk individuals, because multiple genetic risks contribute to the disease. Therefore, it is essential to develop tests that integrate information from multiple AD risk genes to accurately determine an individual's relative risk of developing AD over their lifetime.
"Our study demonstrates the efficacy of deep learning methods for genetic research and risk prediction for Alzheimer's disease. This breakthrough will greatly accelerate population-scale screening and staging of Alzheimer's disease risk. Besides risk prediction, this approach supports the grouping of individuals according to their disease risk and provides insights into the mechanisms that contribute to the onset and progression of the disease," said Ip.
Lei remarked that, "this study exemplifies how the application of AI to the biological sciences can significantly benefit biomedical and disease-related studies. By utilizing a neural network, we effectively captured nonlinearity in high-dimensional genomic data, which improved the accuracy of Alzheimer's disease risk prediction. In addition, through AI-based data analysis without human supervision, we categorized at-risk individuals into subgroups, which revealed insights into the underlying disease mechanisms. Our research also highlights how AI can elegantly, efficiently, and effectively address interdisciplinary challenges. I firmly believe that AI will play a vital role in various health care fields in the near future."
The study was conducted in collaboration with researchers at the Shenzhen Institute of Advanced Technology and University College London as well as clinicians at local Hong Kong hospitals including Prince of Wales Hospital and Queen Elizabeth Hospital. The findings were recently published in Communications Medicine. The research team is now refining the model and aims to ultimately incorporate it into standard screening workflows.
More information: Xiaopu Zhou et al, Deep learning-based polygenic risk analysis for Alzheimer's disease prediction, Communications Medicine (2023). DOI: 10.1038/s43856-023-00269-x