This article has been reviewed according to Science X's editorial process and policies. Editors have highlighted the following attributes while ensuring the content's credibility:
fact-checked
peer-reviewed publication
trusted source
proofread
Can AI help hospitals spot patients in need of extra non-medical assistance?
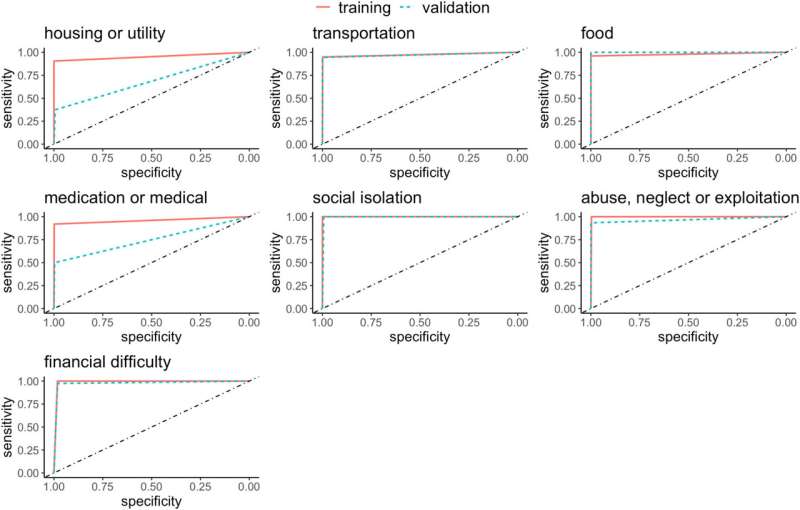
In the rush to harness artificial intelligence and machine learning tools to make care more efficient at hospitals nationwide, a new study points to another possible use: identifying patients with non-medical needs that could affect their health and ability to receive care.
These social determinants of health—everything from transportation and housing to food supply and availability of family and friends as supports—can play a major role in a patient's health and use of health care services.
The new study focuses on a patient population with especially complex needs: people with Alzheimer's disease or other forms of dementia. Their condition can make them especially reliant on others to get them to medical appointments and social activities, handle medications and finances, shop and prepare food, and more.
The results of the study show that a rule-based natural language processing tool successfully identified patients with unstable access to transportation, food insecurity, social isolation, financial problems and signs of abuse, neglect, or exploitation.
The researchers found that a rule-based NLP tool—a kind of AI that analyzes human speech or writing—was far superior to deep learning and regularized logistic regression algorithms for identifying patients' social determinants of health.
However, even the NLP tool did not do well enough at identifying needs related to housing or affording or taking medication.
The study was led by Elham Mahmoudi, Ph.D., a health economist at Michigan Medicine, the University of Michigan's academic medical center, and Wenbo Wu, Ph.D., who completed the work while earning a doctorate at the U-M School of Public Health and is now at New York University. Mahmoudi and two other authors are in the Department of Family Medicine.
They and their colleagues compared the SDOH-spotting capabilities of three different AI techniques, first training them on a set of 700 patient records to teach them the kinds of words and phrases to look for, and then using them on 300 records and judging the results.
The tools only looked at the anonymized contents of emergency department and inpatient social worker notes made between 2015 and 2019 in the electronic health records of 231 patients with dementia.
Mahmoudi says the team is now working to prospectively validate the NLP algorithm against the SDOH questionnaire that has recently begun to be provided to all primary care patients at Michigan Medicine. That will allow them to compare what the computer program finds and what patients say in response to questions about their situation.
"We are also preparing a pilot program that will evaluate the feasibility of an intervention that addresses these social determinants of health, and connect identified people with community resources," said Mahmoudi. "In the meantime, we hope our current results show that this algorithm can be used by clinicians, case managers and social workers to proactively address the social needs of patients with dementia, and potentially other vulnerable patient populations."
In addition to Mahmoudi and Wu, the study's authors are Kaes J. Holkeboer and Lorrie Carbone LMSW of the U-M Department of Family Medicine, and Temidun O. Kolawole of Johns Hopkins University.
More information: Wenbo Wu et al, Natural language processing to identify social determinants of health in Alzheimer's disease and related dementia from electronic health records, Health Services Research (2023). DOI: 10.1111/1475-6773.14210