This article has been reviewed according to Science X's editorial process and policies. Editors have highlighted the following attributes while ensuring the content's credibility:
fact-checked
peer-reviewed publication
trusted source
proofread
How AI inferences of race in medical images can improve or worsen health care disparities
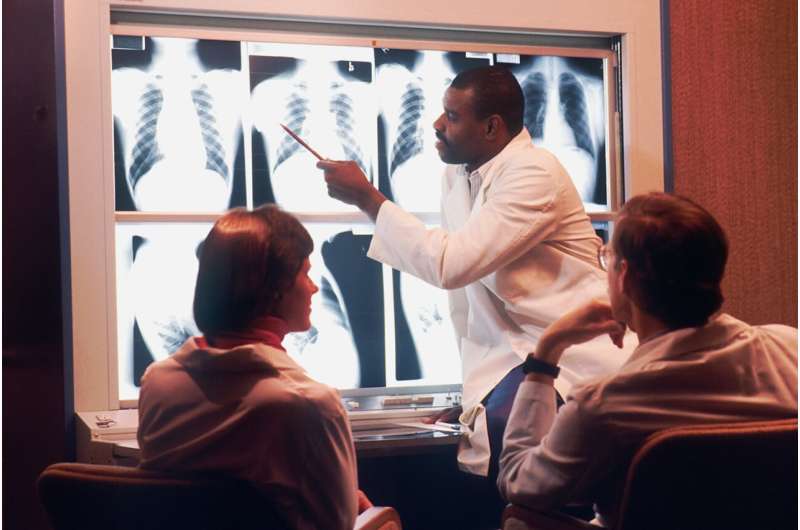
Among the hottest sectors for artificial intelligence (AI) adoption is health care. The Food and Drug Administration has now approved more than 500 AI devices—most within just the last couple of years—to assist doctors across a range of tasks, from gauging heart failure risk to diagnosing cancer.
Amid this surge, recent studies have revealed that AI models can predict the demographics of patients, including race, directly from medical images, even though no distinguishing anatomical or physiological features are evident to human clinicians.
These findings have sparked concern that AI systems could discriminate against patients and exacerbate health care disparity. By the same token, though, the systems could enhance monitoring of patient outcomes connectable to race, as well as identify new risk factors for disease.
Calling attention to these implications, James Zou, an affiliate of the Stanford Institute for Human-Centered AI, and colleagues have penned a new "Perspective" article for the journal Science. In this interview, Zou—an assistant professor of biomedical data science and, by courtesy, of computer science and of electrical engineering at Stanford—discusses the promise and peril of AI in predicting patient demographics.
Why is race a potentially problematic concept in health care settings?
Zou: Race is a complex social construct with no biological basis. The concept of who is this or that "race" varies across time and different contexts and environments; it depends on what country you're in, what century you're in.
There are other human variables, such as genetic ancestry and genetic risks of different diseases, that are more nuanced and likely more relevant for health care.
Is AI plausibly discerning biological differences that human clinicians and anatomists have overlooked in medical imagery or is it more likely that AI is drawing inferences based on other features in available data?
Many AI models are still essentially uninterpretable "black boxes," in the sense that users do not really know what features and information the AI algorithms are using to arrive at particular predictions. That said, we don't have evidence that there are biological differences in these images across different groups that the AI is picking up on.
We do think that the quality and features of the data and of the training sets used to develop the AI play a major role. For instance, patients in one hospital area or clinic location may be more likely to have certain co-morbidities, or other medical conditions, which actually have various manifestations in the images. And the algorithm might pick those manifestations up as artifacts and make spurious correlations to race, because patients of a particular racial category happen to live near and thus go to that hospital or clinic.
Another possibility is systemic technical artifacts, for instance from the types of machines and the methods used to collect medical images. Even in the same hospital, there can be two different imaging centers, maybe even using the same equipment, but it could just be that staff are trained differently in one imaging room compared with the next, such as on how long to image a patient or from what angle. Those variances could lead to different outputs that can show up as systemic patterns in the images that the AI correlates with racial or ethnic demographics, "rightly" or "wrongly," of course keeping in mind that these demographic categories can be crude and arbitrary.
How could AI's discernment of hidden race variables exacerbate health care inequalities?
If the algorithm uses race or some race proxy to make its diagnostic predictions, and doctors are not aware that race is being used, that could lead to dangerous under- or over-diagnosing of certain conditions. Looking deeper at the imaging machines and training sets I mentioned before, suppose patients of a certain race were likelier to have scans done on Type A X-ray machines because those machines are deployed where those people live.
Now suppose that positive cases of, say, lung diseases in the training set for the AI algorithms were collected mostly from Type B X-ray machines. If the AI learns to factor in machine type when predicting race variables and whether patients have lung disease, the AI may be less likely to predict lung disease for people tested on Type A machines.
In practice, that would mean the people getting scanned by Type A machines could be under-diagnosed for lung diseases, leading to health care disparity.
On the flip side, how can AI's ability to infer racial variables advance goals of health care equity?
AI could be used to monitor, assess, and reduce health care disparity in instances where medical records or research studies do not capture patient demographic data. Without these data, it's very hard to know whether a certain group of patients is actually getting similar care or having similar outcomes compared with other groups of patients. In this way, if AI can accurately infer race variables for patients, we could use the imputed race variables as a proxy to assess health care efficacy across populations and reduce disparities in care. These assessments would also feed back in helping us audit the AI's performance in distinguishing patient demographics and making sure the inferences the AI is making are not themselves perpetuating or introducing health care disparity.
Another important benefit is that AI can potentially provide us with far more granular classifications of demographic groups than the standard, discrete categories of race we often encounter. For example, anyone of Asiatic descent—whether from South Asia or East Asia, or whether Chinese or Japanese—is usually grouped under one crude umbrella, "Asian," on standard survey forms or medical records.
In contrast, AI algorithms often represent individual patients on a continuous spectrum of variation in ancestry. So there's interesting potential there with AI to learn about more granular patient subgroups and evaluate medical services provided to them and their outcomes.
More information: James Zou et al, Implications of predicting race variables from medical images, Science (2023). DOI: 10.1126/science.adh4260