This article has been reviewed according to Science X's editorial process and policies. Editors have highlighted the following attributes while ensuring the content's credibility:
fact-checked
peer-reviewed publication
trusted source
proofread
Social media algorithms exploit how humans learn from their peers
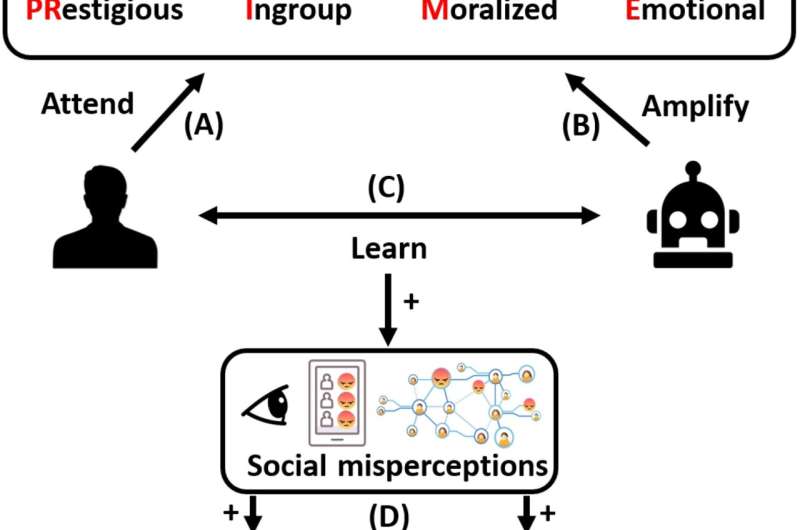
In prehistoric societies, humans tended to learn from members of our ingroup or from more prestigious individuals, as this information was more likely to be reliable and result in group success. However, with the advent of diverse and complex modern communities—and especially in social media—these biases become less effective. For example, a person we are connected to online might not necessarily be trustworthy, and people can easily feign prestige on social media.
In a review published in the journal Trends in Cognitive Science on August 3rd, a group of social scientists describe how the functions of social media algorithms are misaligned with human social instincts meant to foster cooperation, which can lead to large-scale polarization and misinformation.
"Several user surveys now both on Twitter and Facebook suggest most users are exhausted by the political content they see. A lot of users are unhappy, and there's a lot of reputational components that Twitter and Facebook must face when it comes to elections and the spread of misinformation," says first author William Brady, a social psychologist in the Kellogg School of Management at Northwestern.
"We wanted to put out a systematic review that's trying to help understand how human psychology and algorithms interact in ways that can have these consequences," says Brady. "One of the things that this review brings to the table is a social learning perspective. As social psychologists, we're constantly studying how we can learn from others. This framework is fundamentally important if we want to understand how algorithms influence our social interactions."
Humans are biased to learn from others in a way that typically promotes cooperation and collective problem-solving, which is why they tend to learn more from individuals they perceive as a part of their ingroup and those they perceive to be prestigious. In addition, when learning biases were first evolving, morally and emotionally charged information was important to prioritize, as this information would be more likely to be relevant to enforcing group norms and ensuring collective survival.
In contrast, algorithms are usually selecting information that boosts user engagement in order to increase advertising revenue. This means algorithms amplify the very information humans are biased to learn from, and they can oversaturate social media feeds with what the researchers call Prestigious, Ingroup, Moral, and Emotional (PRIME) information, regardless of the content's accuracy or representativeness of a group's opinions.
As a result, extreme political content or controversial topics are more likely to be amplified, and if users are not exposed to outside opinions, they might find themselves with a false understanding of the majority opinion of different groups.
"It's not that the algorithm is designed to disrupt cooperation," says Brady. "It's just that its goals are different. And in practice, when you put those functions together, you end up with some of these potentially negative effects."
To address this problem, the research group first proposes that social media users need to be more aware of how algorithms work and why certain content shows up on their feed. Social media companies don't typically disclose the full details of how their algorithms select for content, but one start might be offering explainers for why a user is being shown a particular post.
For example, is it because the user's friends are engaging with the content or because the content is generally popular? Outside of social media companies, the research team is developing their own interventions to teach people how to be more conscious consumers of social media.
In addition, the researchers propose that social media companies could take steps to change their algorithms, so they are more effective at fostering community. Instead of solely favoring PRIME information, algorithms could set a limit on how much PRIME information they amplify and prioritize presenting users with a diverse set of content. These changes could continue to amplify engaging information while preventing more polarizing or politically extreme content from becoming overrepresented in feeds.
"As researchers we understand the tension that companies face when it comes to making these changes and their bottom line. That's why we actually think these changes could theoretically still maintain engagement while also disallowing this overrepresentation of PRIME information," says Brady. "User experience might actually improve by doing some of this."
More information: Algorithm-mediated social learning in online social networks, Trends in Cognitive Sciences (2023). DOI: 10.1016/j.tics.2023.06.008