This article has been reviewed according to Science X's editorial process and policies. Editors have highlighted the following attributes while ensuring the content's credibility:
fact-checked
peer-reviewed publication
trusted source
proofread
Self-constructed machine-learning model reveals early predictors of glioma evolution
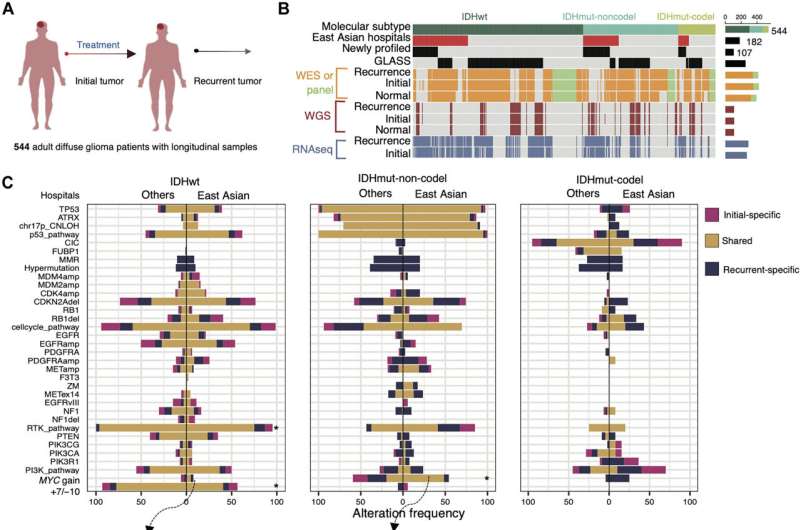
An international research team led by Hong Kong University of Science and Technology (HKUST) has unveiled how primary brain tumors evolve under therapy, and has developed an artificial intelligence (AI)-powered model for patients to predict their prognosis, shedding light on better patient management strategies and precision oncology.
Malignant diffuse gliomas are the most common primary brain tumors in adults, which are primarily treated through a combination of surgery, radiotherapy, and the chemotherapy agent temozolomide (TMZ). However, TMZ treatment can usually only prolong patients' overall survival time for around three months, as they almost inevitably suffer a relapse of aggressive gliomas. Until now, the molecular mechanisms driving glioma evolution under the standard therapy have remained elusive.
To unravel the mystery, a research team led by Prof. Wang Jiguang, Padma Harilela Associate Professor of Life Science in the HKUST's Division of Life Science and Department of Chemical and Biological Engineering, comprehensively analyzed molecular and clinical data of tumor samples from 544 glioma patients, including 182 patients of East Asian descent, to identify genomic and transcriptomic predictors of tumor evolution in all glioma subtypes.
The research is published in the journal Science Translational Medicine.
After analyzing the big data, the team found early predictors associated with TMZ-resistance and rapid progression of gliomas. These predictors include increased expression of regulator gene MYC or MYC-targets activation at initial diagnosis, which lead to treatment-induced hypermutation. Additionally, the deletion of CDKN2A gene at the time of diagnosis was also found to strongly predict the tumor's accelerated advancement to later stages.
The team further discovered that East Asian brain tumors have remarkably different genetic mutations from their Caucasian counterparts. East Asian patients see a reduced frequency of chromosomes 7-gain and 10-loss, as well as an increased frequency of MYC copy number gain. Additionally, rs55705857(G)—a known high-glioma risk factor found in Caucasian patients—is less frequently observed in East Asian population.
"These findings emphasize the significance of tailored therapies targeting cancers," said Prof. Wang. "We believe the discovery of these early predictors in glioma evolution could help develop precision medicine of this aggressive type of brain tumor, especially for recurrent patients."
To help better evaluate patient prognosis, the research team developed a machine learning model named CELLO2 (Cancer EvoLution for LOngitudinal data version 2), to evaluate patient prognosis after the initial diagnosis. The trained model could accurately predict whether the recurrent tumor will progress into more aggressive forms under the TMZ treatment, and to identify patients of higher risk.
To facilitate public access to the predictive model, the team developed an interactive and openly available web portal (CELLO2) for patients and doctors to explore longitudinal glioma data resource and make predictions regarding the development of treatment-induced hypermutation and grade progression based on clinical and genomic features. The platform serves as a valuable tool for patients, allowing them to gain a deeper understanding of the aggressiveness of their brain tumors.
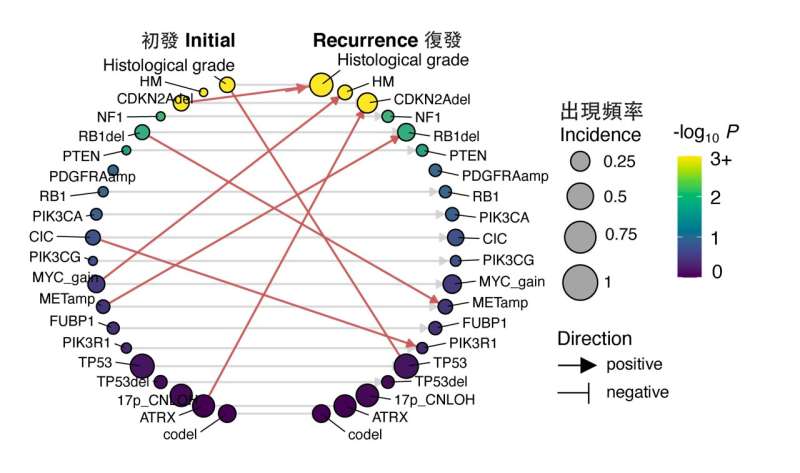
Prof. Jiang Tao, Professor of Beijing Tiantan Hospital at Capital Medical University and Director of Beijing Neurosurgical Institute, said, "CELLO2 is the first valuable tool to predict the potential increase in recurrent tumor grade or drug resistance based on molecular features of the primary tumor. It provides important references for clinical management and prognosis estimation."
The research was conducted in collaboration with Beijing Tiantan Hospital, Samsung Medical Center, and Prince of Wales Hospital at the Chinese University of Hong Kong (CUHK).
Moving forward, the team plans to further enhance the machine-learning model by integrating additional patient data, which could help explore other molecular mechanisms that also play a role in the development of drug resistance within gliomas. The team is also working with CUHK, Beijing Tiantan Hospital and Shanghai Huashan Hospital to develop an AI platform that combines imaging and multi-omics data to advance precision neuro-oncology.
More information: Quanhua Mu et al, Identifying predictors of glioma evolution from longitudinal sequencing, Science Translational Medicine (2023). DOI: 10.1126/scitranslmed.adh4181