This article has been reviewed according to Science X's editorial process and policies. Editors have highlighted the following attributes while ensuring the content's credibility:
fact-checked
peer-reviewed publication
trusted source
proofread
Researchers take new AI approach to analyzing tumors
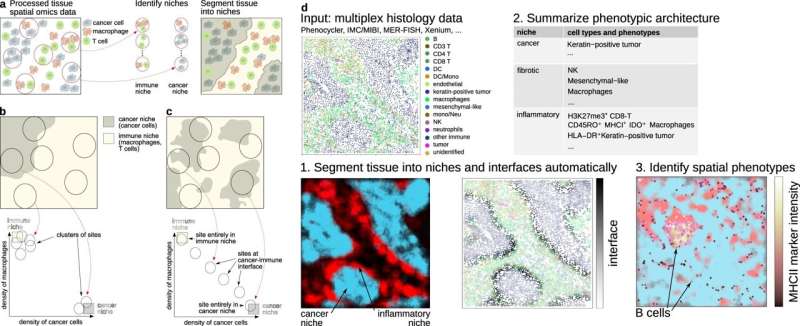
Researchers at Karolinska Institutet and SciLifeLab in Sweden have combined artificial intelligence (AI) techniques used in satellite imaging and community ecology to interpret large amounts of data from tumor tissue. The method, presented in the journal Nature Communications, could contribute to more personalized treatment of cancer patients.
While recent advances in tumor imaging provide a great insight into the microscopic world of tumors, the challenge is to interpret the huge amount of data generated. With hundreds of molecules being measured simultaneously in tens or hundreds of thousands of cells, it has become difficult for researchers to know what molecules and cells to focus on.
AI methods can in principle help researchers analyze this avalanche of data and determine what to focus on. However, traditional AI such as deep neural networks often performs tasks without providing clear explanations that are understandable to humans. Details of how the process works are hidden or difficult to access in a so-called black box.
The research team at Karolinska Institutet and SciLifeLab recognized the limitations of such methods and sought inspiration from other fields. They identified well-established analysis techniques in satellite imaging and ecology dating back to the 2000s and 1950s, respectively.
Similar to interpreting satellite images
New AI methods are continuously developed to interpret data from satellite images, for example, to automatically identify cities, lakes, forests, and deserts within large satellite images. In ecology, advanced techniques are used to reveal how species of plants, animals, and micro-organisms cohabit as communities within a given geographical area.
"We realized that the interpretation of tumor images is similar to the interpretation of satellite images and that the relationships between cells in a tissue are similar to the relationships between species in ecology," explains Jean Hausser, senior researcher at the Department of Cell and Molecular Biology, Karolinska Institutet, who led the research. "By combining techniques used in satellite imaging and ecology and adapting them for the analysis of tumor tissue, we have now been able to turn complex data into new insights into how cancer works."
The next step is to apply the new method in clinical trials. The researchers are collaborating with a major cancer hospital in Lyon, France, to seek answers to why only some patients respond to cancer immunotherapy. In another collaboration with the Mayo Clinic in the US, they are investigating why some breast cancer patients don't need chemotherapy.
"With our new method, we can reveal important details in tumor tissue that can determine whether a cancer treatment works or not. The long-term goal is to be able to tailor cancer treatments to individual needs and avoid unnecessary side effects," says Jean Hausser.
More information: Anissa El Marrahi et al, NIPMAP: niche-phenotype mapping of multiplex histology data by community ecology, Nature Communications (2023). DOI: 10.1038/s41467-023-42878-z