This article has been reviewed according to Science X's editorial process and policies. Editors have highlighted the following attributes while ensuring the content's credibility:
fact-checked
peer-reviewed publication
trusted source
proofread
Unlocking the human genome: Innovative machine learning tool predicts functional consequences of genetic variants
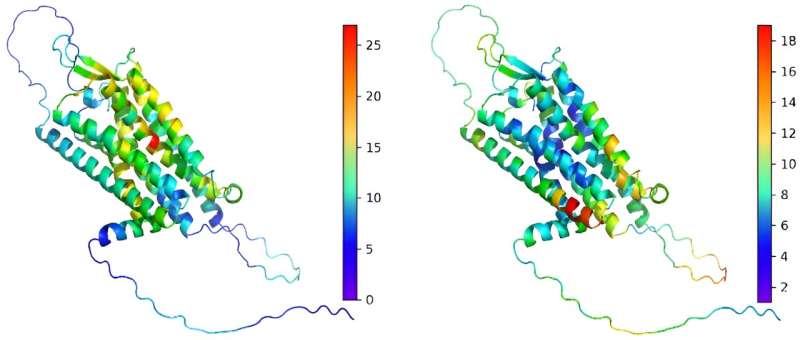
In a novel study, researchers from the Icahn School of Medicine at Mount Sinai have introduced LoGoFunc, an advanced computational tool that predicts pathogenic gain and loss-of-function variants across the genome.
Unlike current methods that predominantly focus on loss of function, LoGoFunc distinguishes among different types of harmful mutations, offering potentially valuable insights into diverse disease outcomes. The findings are described in Genome Medicine.
Genetic variations can alter protein function, with some mutations boosting activity or introducing new functions (gain of function), while others diminish or eliminate function (loss of function). These changes can have significant implications for human health and the treatment of disease.
"Tools presently available fall short in differentiating between gain and loss of function, which motivated us to develop LoGoFunc. This matters because these variants impact protein activity differently, influencing disease outcomes. We created an innovative tool that addresses a critical gap in the field, providing a practical way to understand the functional consequences of genetic variations on a larger scale," says co-senior corresponding author Yuval Itan, Ph.D., Associate Professor of Genetics and Genomic Sciences and a core member of The Charles Bronfman Institute for Personalized Medicine at Icahn Mount Sinai.
LoGoFunc uses machine learning trained on a database of known pathogenic gain-of-function and loss-of-function mutations identified in the literature. It considers a wide range of 474 biological features, including data from protein structures predicted by AlphaFold2 and network features reflecting human protein interactions. Tested on sets from the Human Gene Mutation Database and ClinVar, LoGoFunc demonstrated high accuracy in predicting gain-of-function, loss-of-function, and neutral variants, according to the investigators.
"Beyond personalized medicine, LoGoFunc has implications for drug discovery, genetic counseling, and accelerating genetic research. Its accessibility promotes collaboration and offers a comprehensive view of variant impact across the human genome," says co-senior corresponding author Avner Schlessinger, Ph.D., Professor of Pharmacological Sciences and Associate Director of the Mount Sinai Center for Therapeutics Discovery.
"Therefore, we believe that LoGoFunc holds promise for precision medicine, enabling the possibility of more tailored treatments based on an individual's genetic makeup."
The investigators caution that while these findings are a significant step forward, translating them into clinical applications requires further validation and integration with other medical information. LoGoFunc's predictions are based on training data and inherent assumptions. Ongoing validation efforts are crucial for reliable outcomes. As genetic data continues to grow, refining LoGoFunc's capabilities and extending its scope are priorities for future research.
"By bridging the gaps, the tool enhances our understanding of genetic variations that contribute to diseases, paving the way for personalized treatment strategies and drug discovery," says study first author David Stein, a Ph.D. candidate at Icahn Mount Sinai. "We believe that LoGoFunc will be a powerful tool for deciphering the functional consequences of genetic variations. While its potential applications are vast, ongoing validation efforts will ensure its real-world impact."
The tool's predictions for missense variants across the entire genome are accessible for non-commercial use and analysis.
More information: David Stein et al, Genome-wide prediction of pathogenic gain- and loss-of-function variants from ensemble learning of a diverse feature set, Genome Medicine (2023). DOI: 10.1186/s13073-023-01261-9