This article has been reviewed according to Science X's editorial process and policies. Editors have highlighted the following attributes while ensuring the content's credibility:
fact-checked
trusted source
proofread
Mathematicians build 'attentive' neural network that recognizes breast cancer with 99.6% accuracy
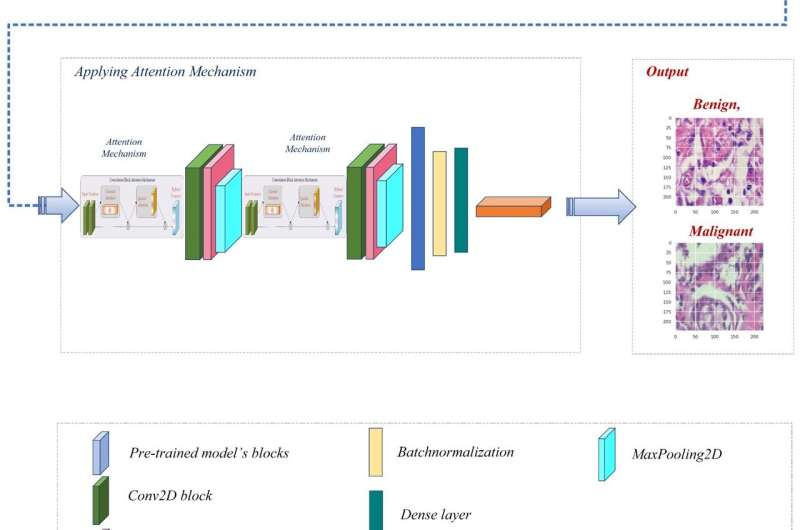
Mathematicians from RUDN University have built a neural network that recognizes breast cancer on histological samples with almost 100% accuracy. This was achieved with the help of a module, which "sharpened the attention" of the model. The results were published in Life.
The prognosis for a patient with breast cancer is largely determined by the stage at which the diagnosis was made. Histological examination is considered the gold standard for diagnosis. However, the histology result is influenced by subjective factors, as well as the quality of the sample. Inaccuracies lead to an erroneous diagnosis.
A RUDN mathematician and colleagues from China and Saudi Arabia have developed a machine learning model that will help more accurately recognize cancer in histological images. Thanks to an additional module that improved the "attention" of the neural network, accuracy reached almost 100%.
"Computer classification of histological images will reduce the burden on doctors and increase the accuracy of tests. Such technologies will improve the treatment and diagnosis of breast cancer. Deep learning methods have shown promising results in medical image analysis problems in recent years," Ammar Muthanna, Ph.D., Director of the Scientific Center for Modeling Wireless 5G Networks at RUDN University said.
The mathematicians tested several convolutional neural networks, which they supplemented with two convolutional attention modules—additional modules that are needed to detect objects in images. The model was trained and tested on the BreakHis data set. It consists of almost 10,000 histological images of different scales obtained from 82 patients.
The best results were shown by a model composed of the DenseNet211 convolutional network with attention modules. The accuracy of this model reached 99.6%. Mathematicians have noticed that the recognition of cancerous formations is influenced by scale. The fact is that at different levels of zoom, the images appear to be of different quality, and the cancerous objects themselves are visible in different ways. Therefore, in a real application scenario, a suitable approximation will need to be considered.
"The attention modules in the model improved feature extraction and the overall performance of the model. With their help, the model focused on significant areas of the image and highlighted the necessary information. It shows the importance of attention mechanisms in the analysis of medical images," Ammar Muthanna, Ph.D., director of the Scientific Center for Modeling Wireless 5G Networks at RUDN University said.
More information: Asadulla Ashurov et al, Improved Breast Cancer Classification through Combining Transfer Learning and Attention Mechanism, Life (2023). DOI: 10.3390/life13091945