This article has been reviewed according to Science X's editorial process and policies. Editors have highlighted the following attributes while ensuring the content's credibility:
fact-checked
trusted source
proofread
AI-supported image analysis: How metrics determine quality
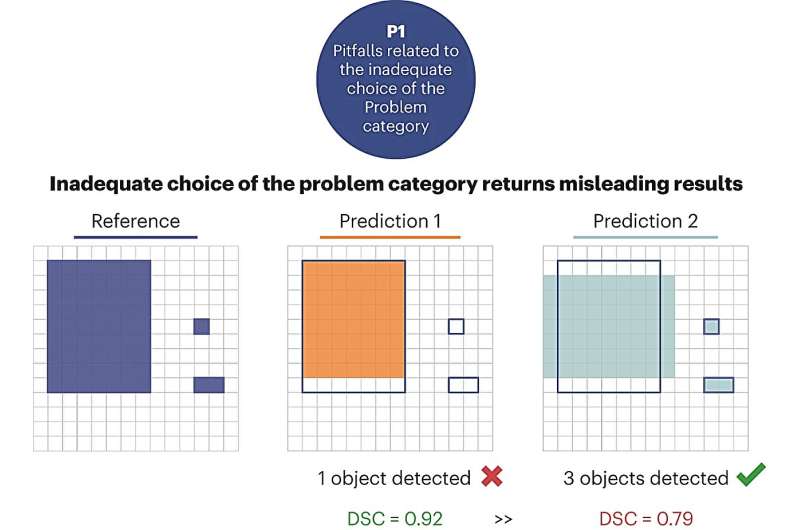
More and more areas of medicine are relying on support from artificial intelligence (AI). This is particularly true for the wide range of questions based on the evaluation of image data: for example, doctors search mammograms for the tiny foci of cancer or calculate the volume of a brain tumor based on the tomographic images from an MRI.
They use endoscopic images of the intestine to track down polyps, and when evaluating microscopic tissue sections, subtle changes in individual cells must be detected.
But are the algorithms used for these different types of image analysis really always suitable for the task in hand? This depends to a large extent on which measured variables, referred to as "metrics" in technical terms, they record—and whether these are actually suitable for the task in question.
"We often notice that validation metrics are used that are not at all relevant to the task from a clinical perspective," says Lena Maier-Hein from the DKFZ, citing an example: "When searching for metastases in the brain, it is initially more important that the algorithm detects even the tiniest lesions than that it can define the contours of each individual metastasis with high precision."
Lena Maier-Hein and her colleagues fear that the use of unsuitable validation metrics can hinder scientific progress and delay the introduction of important image analysis methods into clinical practice.
But which metrics are suitable for a given clinical question, considering all strengths, weaknesses, and limitations? To find out, the DKFZ data scientists used a multi-stage, structured process to survey opinion leaders from academia and industry from over 70 research institutions worldwide. The survey allowed them to gather information that was previously only available in scattered locations around the world.
"With this work, we are making reliable and comprehensive information on the problems and pitfalls associated with validation metrics in image analysis available to experts for the first time," says Annika Reinke, one of the lead authors.
As a structured body of information that can be accessed by researchers from all disciplines, the work aims to increase understanding of a key problem in AI-assisted image analysis. Although the focus is on the analysis of medical images, the information can also be transferred to other areas of image analysis.
In a second paper, the expert consortium led by the Heidelberg researchers now describes "Metrics Reloaded": A comprehensive framework to help physicians and scientists select metrics that are appropriate to the problem. "Metrics Reloaded" can be used as an online tool.
"Users are guided through a comprehensive set of questions to create a precise fingerprint of their image analysis problem. The tool also draws attention to specific problems that arise in certain biomedical issues," explains Paul Jäger, one of the senior authors of the two publications
Metrics Reloaded is suitable for all different categories of problems in image analysis, i.e., for the classification of images, object detection or the assignment of individual pixels (semantic segmentation). The tool works completely independently of the image source, so it can be used just as well for CT or MRI images as for microscopic images. Metrics Reloaded is also suitable for image analyses beyond biomedical issues.
"Metrics Reloaded is the first systematic guide that shows users of AI-based image analyses the way to the right algorithm. We hope that Metrics Reloaded will be used as widely as possible as quickly as possible, as this could significantly improve the quality and reliability of the results of AI-supported image analyses. This would also promote confidence in AI-supported image analysis in routine clinical practice," says Minu Tizabi, one of the lead authors.
The research was published as two papers in Nature Methods.
More information: Reinke, A. et al. Understanding metric-related pitfalls in image analysis validation, Nature Methods (2024). DOI: 10.1038/s41592-023-02150-0. www.nature.com/articles/s41592-023-02150-0
Maier-Hein, L. et al. Metrics reloaded: recommendations for image analysis validation, Nature Methods (2024). DOI: 10.1038/s41592-023-02151-z. www.nature.com/articles/s41592-023-02151-z