This article has been reviewed according to Science X's editorial process and policies. Editors have highlighted the following attributes while ensuring the content's credibility:
fact-checked
proofread
Advancing drug discovery with AI: Introducing the KEDD framework
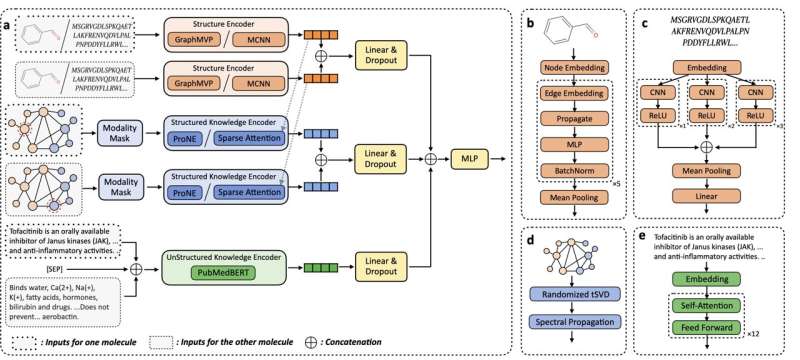
A study published in Health Data Science introduces an end-to-end deep learning framework, known as Knowledge-Empowered Drug Discovery (KEDD), aimed at revolutionizing the field of drug discovery. This innovative framework adeptly integrates structured and unstructured knowledge, enhancing the AI-driven exploration of molecular dynamics and interactions.
Traditionally, AI applications in drug discovery have been constrained by their focus on singular tasks, neglecting the rich tapestry of structured and unstructured data that could enrich their predictive accuracy. These limitations are particularly pronounced when dealing with novel compounds or proteins, where existing knowledge is scant or absent, often hampered by the prohibitive costs of manual data annotation.
Professor Zaiqing Nie, from Tsinghua University's Institute for AI Industry Research, emphasizes the enhancement potential of AI in drug discovery through KEDD. This framework synergizes data from molecular structures, knowledge graphs, and biomedical literature, offering a comprehensive approach that transcends the limitations of conventional models.
At its core, KEDD employs robust representation learning models to distill dense features from various data modalities. Following this, it integrates these features through a fusion process and leverages a predictive network to ascertain outcomes, facilitating its application across a spectrum of AI-facilitated drug discovery endeavors.
-
By direct searching from the BMKG dataset, reconstruction with sparse attention, and modality masking, we can obtain knowledge graph features for arbitrary biomolecules, thereby addressing the missing modality problem. Credit: [ Yizhen Luo, Institute for AI Industry Research (AIR), Tsinghua University] -
KEDD consistently outperforms prior approaches in drug-target interaction prediction under warm-start and cold-start scenarios. Credit: Yizhen Luo, Institute for AI Industry Research (AIR), Tsinghua University -
KEDD identifies potential inhibitors for ACE2, an entry receptor of SARS-CoV-2. KEDD jointly reasons over molecular structure features in c, knowledge graph embeddings in d, and textual representations in e. Credit: Yizhen Luo, Institute for AI Industry Research (AIR), Tsinghua University
The study substantiates KEDD's effectiveness, showcasing its ability to outperform existing AI models in critical drug discovery tasks. Notably, KEDD demonstrates resilience in the face of the 'missing modality problem,' where a lack of documented data on new drugs or proteins could undermine analytical processes. This resilience stems from its innovative use of sparse attention and modality masking techniques, which harness the power of existing knowledge bases to inform predictions and analyses.
Looking forward, Yizhen Luo, a key contributor to the KEDD project, outlines ambitious plans to enhance the framework's capabilities, including the exploration of multimodal pre-training strategies. The overarching objective is to cultivate a versatile, knowledge-driven AI ecosystem that accelerates biomedical research, delivering timely insights and recommendations to advance therapeutic discovery and development.
More information: Yizhen Luo et al, Toward Unified AI Drug Discovery with Multimodal Knowledge, Health Data Science (2024). DOI: 10.34133/hds.0113