Researchers say use of artificial intelligence in medicine raises ethical questions
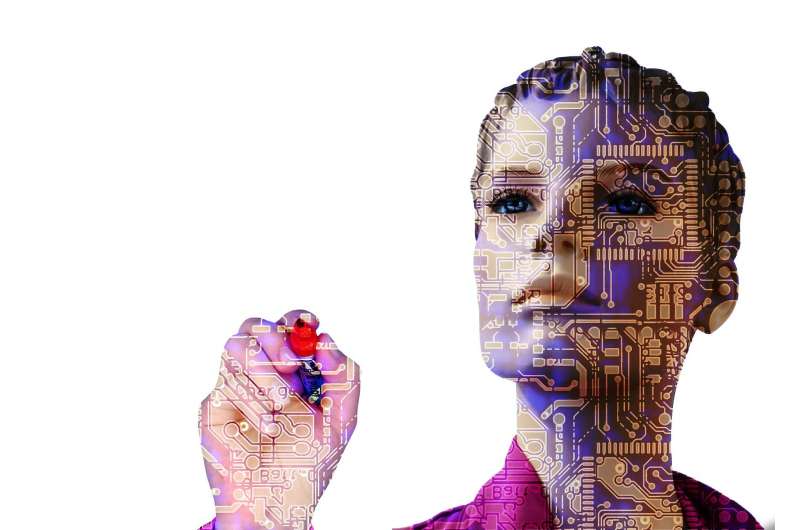
In a perspective piece, Stanford researchers discuss the ethical implications of using machine-learning tools in making health care decisions for patients.
Artificial intelligence is hard at work crunching health data to improve diagnostics and help doctors make better decisions for their patients. But researchers at the Stanford University School of Medicine say the furious pace of growth in the development of machine-learning tools calls for physicians and scientists to carefully examine the ethical risks of incorporating them into decision-making.
In a perspective piece published March 15 in the New England Journal of Medicine, the authors acknowledged the tremendous benefit that machine learning can have on patient health. But they cautioned that the full benefit of using this type of tool to make predictions and take alternative actions can't be realized without careful consideration of the accompanying ethical pitfalls.
"Because of the many potential benefits, there's a strong desire in society to have these tools piloted and implemented into health care," said the lead author, Danton Char, MD, assistant professor of anesthesiology, perioperative and pain medicine. "But we have begun to notice, from implementations in non-health care areas, that there can be ethical problems with algorithmic learning when it's deployed at a large scale."
Among the concerns the authors raised are:
- Data used to create algorithms can contain bias that is reflected in the algorithms and in the clinical recommendations they generate. Also, algorithms might be designed to skew results, depending on who's developing them and on the motives of the programmers, companies or health care systems deploying them.
- Physicians must adequately understand how algorithms are created, critically assess the source of the data used to create the statistical models designed to predict outcomes, understand how the models function and guard against becoming overly dependent on them.
- Data gathered about patient health, diagnostics and outcomes become part of the "collective knowledge" of published literature and information collected by health care systems and might be used without regard for clinical experience and the human aspect of patient care.
- Machine-learning-based clinical guidance may introduce a third-party "actor" into the physician-patient relationship, challenging the dynamics of responsibility in the relationship and the expectation of confidentiality.
"We need to be cautious about caring for people based on what algorithms are showing us," Char said. "The one thing people can do that machines can't do is step aside from our ideas and evaluate them critically."
Sources of bias
In discussing designer intent, which is one source of bias, the authors pointed to private-sector examples of algorithms meant to ensure specific outcomes, such as Volkswagen's algorithm that allowed vehicles to pass emissions tests by reducing their nitrogen oxide emissions during the tests.
David Magnus, Ph.D., senior author of the piece and director of the Stanford Center for Biomedical Ethics, said bias can play into health data in three ways: human bias; bias that is introduced by design; and bias in the ways health care systems use the data.
"You can easily imagine that the algorithms being built into the health care system might be reflective of different, conflicting interests," said Magnus, who is also the Thomas A. Raffin Professor of Medicine and Biomedical Ethics. "What if the algorithm is designed around the goal of saving money? What if different treatment decisions about patients are made depending on insurance status or their ability to pay?"
The authors called for a national conversation about the "perpetual tension between the goals of improving health and generating profit … since the builders and purchasers of machine-learning systems are unlikely to be the same people delivering bedside care."
They also put the responsibility for finding solutions and setting the agenda on health care professionals.
"Ethical guidelines can be created to catch up with the age of machine learning and artificial intelligence that is already upon us," the authors wrote. "Physicians who use machine-learning systems can become more educated about their construction, the data sets they are built on and their limitations. Remaining ignorant about the construction of machine-learning systems or allowing them to be constructed as black boxes could lead to ethically problematic outcomes."
The authors acknowledge the social pressure to incorporate the latest tools in order to provide better health outcomes for patients.
"Artificial intelligence will be pervasive in health care in a few years," said co-author Nigam Shah, MBBS, Ph.D., associate professor of medicine. But health care systems need to be aware of the pitfalls that have happened in other industries, he added.
Shah noted that models are only as trustworthy as the data being gathered and shared. "Be careful about knowing the data from which you learn," he said.
Could data become the doctor?
The authors wrote that what physicians learn from the data needs to be heavily weighed against what they know from their own clinical experience. Overreliance on machine guidance might lead to self-fulfilling prophesies.
For example, they said, if clinicians always withdraw care in patients with certain diagnoses, such as extreme prematurity or brain injury, machine-learning systems may learn that such diagnoses are always fatal. Conversely, machine-learning systems, properly deployed, may help resolve disparities in health care delivery by compensating for known biases or by identifying where more research is needed to balance the underlying data.
Magnus said the example of a current pilot study of an algorithm developed at Stanford to predict the need for a palliative care consultation illustrates how collaborative, careful consideration in the design of an algorithm and use of the data can guard against the misinterpretation of data in making care decisions.
Shah is helping to lead the pilot study. In this case, Magnus said, physicians and designers work closely to ensure that the incorporation of the predictions into the care equation includes guarantees that the physician "has a full understanding that the patient problems are answered and well-understood."
The insertion of an algorithm's predictions into the patient-physician relationship also introduces a third party, turning the relationship into one between the patient and the health care system.
It also means significant changes in terms of a patient's expectation of confidentiality.
"Once machine-learning-based decision support is integrated into clinical care, withholding information from electronic records will become increasingly difficult, since patients whose data aren't recorded can't benefit from machine-learning analyses," the authors wrote.
Magnus said the pressure to turn to data for answers is especially intense in fields that are growing quickly, such as genetic testing and sequencing.
"In a situation where you're looking for any evidence in informing your decision-making that you can get, and now you have all this genetics information and you don't know how to deal with," having clear data can be enormously helpful, he said.
Char, who is doing research funded by the National Institutes of Health on the ethical and social implications of expanded genetic testing of critically ill children, said it's important for health care professionals to figure out how to minimize negative outcomes of data-based decisions in all fields.
"I think society has become very breathless in looking for quick answers," he said. "I think we need to be more thoughtful in implementing machine learning."