Harnessing the power of machine learning for earlier autism diagnosis
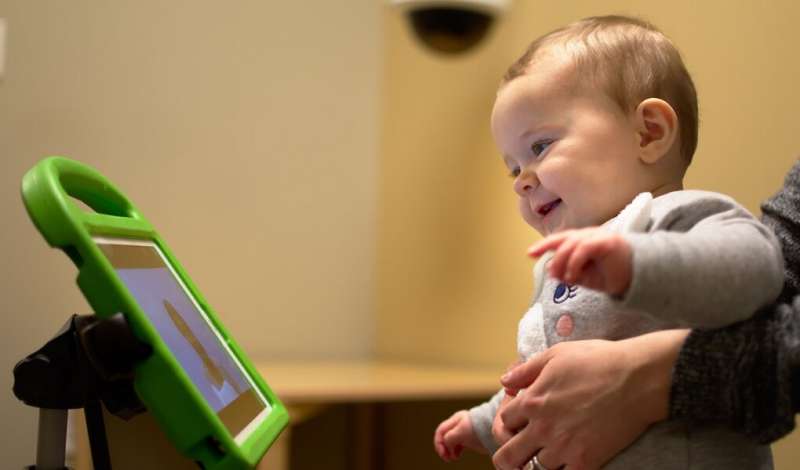
When Grayson Kollins was two and a half years old—just shortly after the birth of his younger sister—his parents noticed that he had all but stopped uttering the sentences and phrases that up until then he had been using to communicate. In addition, his daycare provider mentioned that Grayson had begun repeating phrases over and over, and lacked interest in playing with other children.
Grayson's father Scott Kollins, Ph.D., a clinical psychologist and professor of psychiatry and behavioral sciences in the School of Medicine at Duke, was well aware of the symptoms of autism spectrum disorder, or ASD, a neurodevelopmental disorder that affects the ability to socially interact and communicate with others. Although it usually manifests early in life, it is a lifelong condition and can have profound effects on learning, employment, and personal relationships.
Prompted by these early symptoms, Grayson's parents subsequently had him assessed, and he received a clinical diagnosis of ASD. Around the same time in 2013, Duke was recruiting Geraldine (Geri) Dawson, Ph.D., to join the faculty. Dawson is a clinical psychologist whose pioneering work on the early diagnosis and treatment of ASD, together with that of her colleague Sally Rogers, had resulted in the creation of the first comprehensive behavioral intervention for toddlers with ASD.
"Her book was sitting on my nightstand," recalls Kollins, referring to An Early Start for Your Child with Autism, a book that Dawson co-authored in 2012. "Now," he remembers thinking, "I have this world expert rock star to bounce things off of."
As its name suggests, ASD encompasses a spectrum of possible symptoms and behaviors, ranging from relatively mild difficulties with social interactions in some, to a complete inability to verbalize in others. Persons with ASD manifest difficulty in interacting with other people and reading social cues. They may also engage in repetitive behaviors, or become fixated on particular things or interests, or experience an extreme sensitivity to environmental stimuli such as loud noises. But the cues that hint at ASD are not always obvious, especially in younger children, and often emerge in different ways in different people.
ASD affects roughly one in every 59 children in the United States and occurs more often in boys than girls. Although ASD is seen in all races and ethnic groups, children from ethnic and racial minority backgrounds tend to get diagnosed at a later age than white children, and thus often miss out on early intervention.
"If you've met one person with ASD, you've met one person with ASD," says Dawson, who in addition to being a professor of psychiatry in the School of Medicine is also director of the Duke Center for Autism and Brain Development and the Duke Institute for Brain Sciences. "It's a very heterogeneous disorder."
"One of the first symptoms of autism is that an infant does not pay attention to the social world," Dawson notes. "Right after birth, most infants are really interested in faces and voices, but infants with autism don't develop that natural preference."
Instead, she continues, infants with autism tend to be more drawn to the world of objects. But this dynamic can disrupt the normal pathway of brain development.
"During the infant-toddler period, the brain is rapidly developing—the brain systems that allow us to read facial expressions and understand language develop throughout this time," says Dawson.
During this period, babies need social interaction and language input from their parents and others around them to fuel that development.
"If the babies aren't paying attention, then they are not getting stimulation to those brain systems."
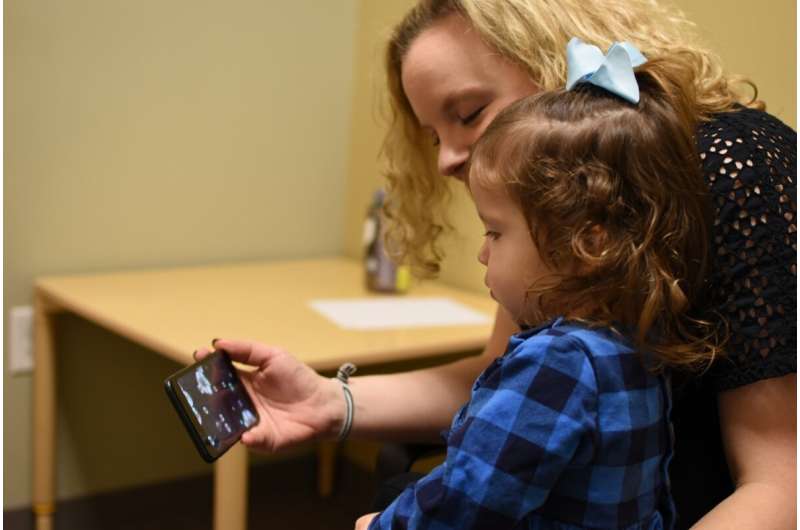
Dawson first became involved in the field of autism research decades ago. Since then, a great deal has been discovered about the symptoms, development, and prevalence of what was once thought to be a very rare disorder. But there remains much to learn about the exact constellation of factors that contribute to causing autism, or how best to intervene.
Still, there's one thing that autism experts are increasingly confident of: owing to the brain's inherent malleability—its neuroplasticity—early detection and intervention are critical to improving outcomes in ASD, especially in terms of language and social skills. While this may sound straightforward, it can be challenging.
"About 50 percent of kids [with autism] also have attention deficit hyperactivity disorder, or ADHD," explains Kollins, who also directs the Duke ADHD Program. He notes that the presence of ADHD—which is often marked by difficulty in sustaining focused attention—can mask symptoms of ASD and delay its diagnosis, sometimes for years.
"Early intervention is important no matter what," Kollins adds, although he emphasizes that it's even more important in the case of ASD, due to the impact on language and socialization. "It leads to better outcomes across the board."
Decades of research into ASD and other neurodevelopmental disorders are starting to yield innovative treatments that can make a significant difference in the lives of those affected by them. But clinicians and families are still left with the question of how to obtain an accurate diagnosis as early as possible so that these interventions can do the most good.
Like Kollins, Dawson has long had an intense interest in finding ways to identify ASD and other neurodevelopmental disorders as early as possible.
The answer to that question, it turns out, may be hiding in plain sight—but it may take the help of machine intelligence to spot it.
Dawson and Kollins began to explore the possibility of applying modern computational resources to the problem. They knew that the field of machine learning, in which computer algorithms are applied to problems that involve sifting enormous amounts of data in order to find hidden patterns and associations, could offer the tools they needed. Kollins and Dawson assembled a group of researchers at Duke to hunt for associations present in the information contained in patient health records. Working under conditions that ensure data security and strict protections for patient privacy, the team hopes to identify patterns that could help diagnose ASD earlier and potentially open the door to new options for treatment.
"We've learned over the last couple of decades that there are a number of early risk factors and risk predictors," says Dawson. "We believe that these are routinely recorded in the EHR [electronic health record] – things like birth history, early developmental history, familial risk factors, significant infectious disease involving high fever, perinatal complications including anoxia…"
"Premature birth, maternal complications during pregnancy, delayed motor activity…" agrees Kollins, ticking off more of the known risk predictors for ASD. None of these factors in isolation says much about any person's likelihood of having ASD or ADHD. Put them all together, however, and a sharper picture begins to emerge.
Dawson and Kollins realized that within a child's medical records was a trove of data already being collected as part of routine health care, data that could be used to develop a risk algorithm. That algorithm in turn could alert physicians to a child who was at higher risk for developing ASD and/or ADHD, prompting intensified screening and surveillance and helping to get effective interventions to children earlier. It could also alert physicians to be on the lookout for other medical conditions that are often associated with neurodevelopmental disorders, such as eating and sleep difficulties.
In fact, Dawson's earlier work had already shown that knowledge of some of these risk factors, such as family history, made it possible to identify signs of ASD as early as 1 year of age—much earlier than the average diagnosis age of 4-5 years (or more, as Kollins noted, in cases where ADHD may be masking symptoms of ASD).
"If we can detect early and provide stimulation, many children do very well," says Dawson, noting that early intervention consisting of behavioral and in some cases medical therapy is associated with substantially better communication skills and learning outcomes, including an average 15-to-17-point increase in IQ score. However, she adds, in many parts of the U.S, there are long waitlists to see a professional with expertise in ASD and, thus, getting a clinical diagnosis of ASD may take up to a year. Further, many people with ASD and ADHD still struggle despite receiving good care, and 30 percent of individuals with autism never learn to speak.
Initially, Kollins recalls, Duke researchers had hoped that EHRs could simply be scanned for diagnosis codes indicative of autism. But such diagnoses, which Kollins describes as "squishy" and not always reliable, do not represent the "ground truth" needed to power a higher-quality algorithm.
"To reliably assess that there may be important relationships that you're not aware of, is where data science comes in," notes Kollins.
With initial support from Duke Forge, Duke's center for health data science, Dawson and Kollins' team developed a pilot program to assess whether such an approach could be used to reliably identify children at risk for neurodevelopmental disorders at an early age and provide information that could be used to guide decision-making about intervention and treatment for doctors and parents. The project had the additional goal of reducing racial and ethnic disparities in ASD/ADHD prevention and treatment.
"We're in the beginning stages," Kollins explains. "We created a data mart of all individuals since Epic [Duke's EHR system] went online and are using those historical data to evaluate whether we can apply a machine learning algorithm to determine which children are likely to develop autism, ADHD, or both."
Dawson notes that in addition to scanning thousands or tens of thousands of medical records for known risk factors, machine learning algorithms are also capable of uncovering previously unknown or unguessed associations lurking in the data—although, as always in machine learning, it's crucial to be able to discriminate meaningful associations from spurious coincidence.
Another aspect of the project also hinged upon the economics of early treatment for ASD and other neurodevelopmental disorders. Early intervention in ASD is not only associated with better outcomes for the individual; it also has the potential to avoid the additional $1.2 million lifetime cost currently associated with ASD. With support from a grant from the National Institutes of Health, the team also aimed to evaluate the degree of added value as well as the cost-effectiveness of the approaches they were developing.
"We have a bigger vision, one of being able to raise a flag in the first year of life that says this is a kid we need to pay attention to," says Matthew Engelhard, MD, Ph.D., a Duke Forge research fellow and member of Dawson and Kollins' team. "If we can raise a flag that gets a substantial number of kids getting diagnosed and treated earlier, then that is success."
Although the team has at its disposal all of the data within Duke's Epic system, not all of it is created equal. Structured data—for instance, a field in the EHR that collects a numerical value such as weight or head circumference—is inherently easier for machines to cope with. However, some of the most important diagnostic clues might be lurking in unstructured data, such as a free-text narrative note from the treating physician. Relatively simple approaches, such as keyword matching, may yield insights. However, training a machine-learning algorithm to read and "understand" free text, a specialized field of AI known as natural language processing, remains a more ambitious goal.
For Dawson, the key metric of success for their project is "being able to empower physicians with more information and advice about how to personalize the care of young patients." She ticks off multiple positive outcomes that this approach might also enable—reducing racial and ethnic disparities in early diagnosis, increasing surveillance and developing digital tools to improve the accuracy of screening that until now has relied largely on imprecise questionnaires that can also be influenced by literacy barriers and lack of parental knowledge about child development.
There are challenges, but Kollins is enthusiastic about "leveraging data science to do some awesome stuff."
"It's a huge commitment," Kollins admits. But he also recalls his early conversations with Dawson as they were first fleshing out their ideas, and remembers clearly what they concluded:
"We can't not do this."