Machine learning that predicts anti-cancer drug efficacy
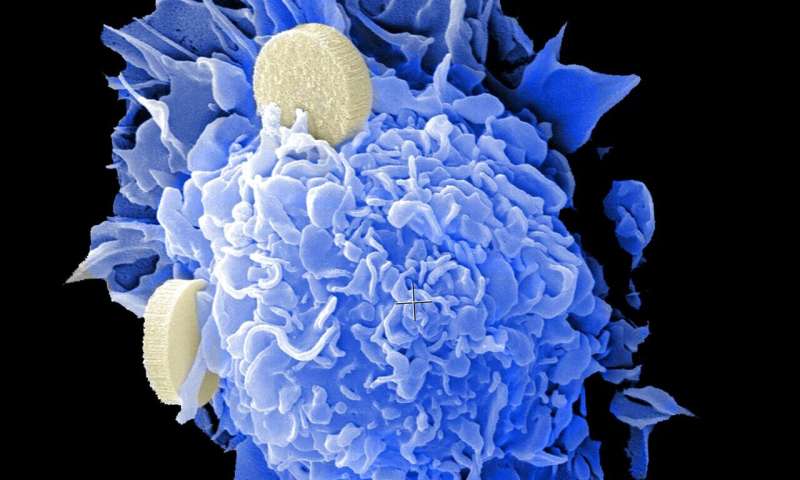
With the advent of pharmacogenomics, machine learning research is well underway to predict patients' drug response that varies by individual from the algorithms derived from previously collected data on drug responses. Entering high-quality learning data that can reflect a person's drug response as much as possible is the starting point for improving the accuracy of the prediction. Previously, preclinical studies of animal models were used which were relatively easier to obtain compared to human clinical data.
In light of this, a research team led by Professor Sanguk Kim in the Department of Life Sciences at POSTECH is drawing attention by successfully increasing the accuracy of anti-cancer drug response predictions by using data closest to a real person's response. The team developed this machine learning technique through algorithms that learn the transcriptome information from artificial organoids derived from actual patients instead of animal models. These research findings were published in the international journal Nature Communications on October 30.
Even patients with the same cancer have different reactions to anti-cancer drugs so customized treatment is considered paramount in treatment development. However, the current predictions were based on genetic information of cancer cells, limiting their accuracy. Due to unnecessary biomarker information, machine learning had an issue of learning based on false signals.
To increase the predictive accuracy, the research team introduced machine learning algorithms that use protein interaction network that can interact with target proteins as well as the transcriptome of individual proteins that are directly related with drug targets. It induces learning the transcriptome production of a protein that is functionally close to the target protein. Through this, only selected biomarkers can be learned instead of false biomarkers that the conventional machine learning had to learn, which increases the accuracy.
In addition, data from patient-derived organoids—not animal models—were used to narrow the discrepancy of responses in actual patients. With this method, colorectal cancer patients treated with 5-fluorouracil and bladder cancer patients treated with cisplatin were predicted to be comparable to actual clinical results.
More information: JungHo Kong et al, Network-based machine learning in colorectal and bladder organoid models predicts anti-cancer drug efficacy in patients, Nature Communications (2020). DOI: 10.1038/s41467-020-19313-8