How does the brain learn?
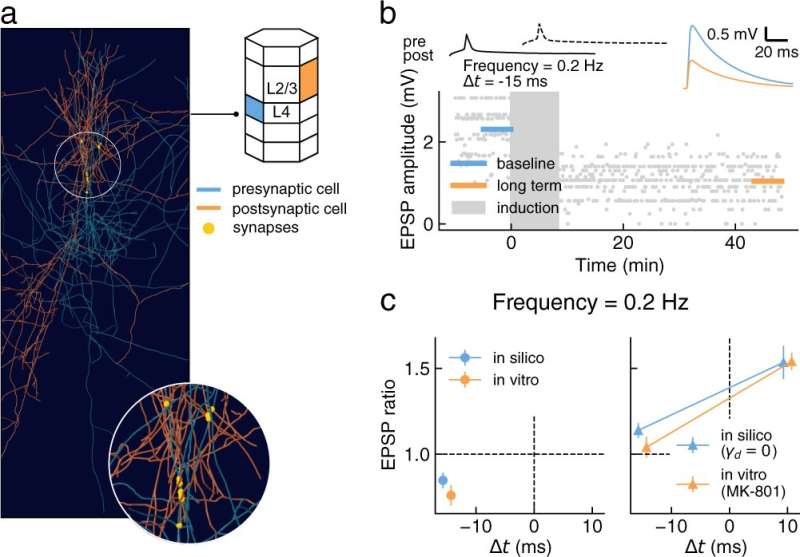
Everyone knows the human brain is extremely complex—but how does it learn, exactly? Well, the answer may be a lot simpler than commonly believed.
An international research team involving Université de Montréal has achieved a major advance in accurately simulating the synaptic changes in the neocortex that are thought to be key to learning, opening the door to a greater understanding of the brain.
The scientists' study—featuring an open-source model—was published June 1 in Nature Communications.
"This opens up a world of new directions for scientific inquiry into how we learn," said Eilif Muller, an IVADO assistant research professor at UdeM and a Canada CIFAR AI Chair, who co-led the study at the Blue Brain Project of the École polytechnique fédérale de Lausanne (EPFL), in Switzerland.
Muller moved to Montreal in 2019 and is pursuing his research at the Architectures of Biological Learning Laboratory, which he founded at the CHU Sainte-Justine Research Center in association with UdeM and Mila, the Quebec Artificial Intelligence Institute.
"Neurons are shaped like trees, and synapses are the leaves on their branches," said Muller, the study's co-senior author.
"Prior approaches to model plasticity have ignored this tree structure, but now we have the computational tools to test the idea that synaptic interactions on branches play a fundamental role in guiding learning in vivo," he said.
"This has important implications for understanding the mechanisms of neurodevelopmental disorders such as autism and schizophrenia, but also for developing powerful new AI approaches inspired by neuroscience."
Muller collaborated with a group of scientists from the EPFL's Blue Brain Project, Université de Paris, Hebrew University of Jerusalem, Instituto Cajal (Spain), and Harvard Medical School to come up with a model of synaptic plasticity in the neocortex based on data-constrained postsynaptic calcium dynamics.
How does it work? It's complicated—but ultimately, simpler than you might think.
The brain is made up of billions of neurons that communicate with each other by forming trillions of synapses. These connection points between neurons are complex molecular machines that are constantly changing as a result of external stimuli and internal dynamics, a process commonly referred to as synaptic plasticity.
In the neocortex, a key area associated with learning of high-level cognitive functions in mammals, pyramidal cells (PCs) account for 80 to 90 percent of neurons and are known to play a major role in learning. Despite their importance, the long-term dynamics of their synaptic changes have been experimentally characterized between only a few types of PCs, and shown to be diverse.
As a result, there has been only limited understanding of the complex neural circuits that they form, especially across the stereotypical cortical layers, which dictate how the diverse regions of the neocortex interact. Muller and his colleagues' innovation was to use computational modeling to come up with a more comprehensive view of the synaptic plasticity dynamics governing learning in these neocortical circuits.
By comparing their results to the available experimental data, they showed in their study that their synaptic plasticity model can capture the varied plasticity dynamics of the diverse PCs making up the neocortical microcircuit. And they did so using only one unified model parameter set, indicating the plasticity rules of the neocortex could be shared across pyramidal cell-types, and thus be predictable.
Most of these plasticity experiments were performed on brain slices of rodents in vitro, where the calcium dynamics driving synaptic transmission and plasticity are significantly altered compared to learning in the intact brain in vivo. Importantly, the study predicts qualitatively different plasticity dynamics from the reference experiments performed in vitro. If confirmed by future experiments, the implications for our understanding of plasticity and learning in the brain would be profound, Muller and his team believe.
"What is exciting about this study is that this is further confirmation for scientists that we can overcome gaps in experimental knowledge using a modeling approach when studying the brain," said EPFL neuroscientist Henry Markram, the Blue Brain Project's founder and director.
"In addition, the model is open source, available on the Zenodo platform," he added.
"Here we have shared hundreds of plastic pyramidal cell connections of different types. Not only is it the most extensively validated plasticity model to date, but it also represents the most comprehensive prediction of the differences between plasticity observed in a petri dish, and in an intact brain.
"This leap is made possible because of our collaborative team-science approach. Moreover, the community can take it further and develop their own versions by modifying or adding to it—this is open science, and it will accelerate progress."
More information: Giuseppe Chindemi et al, A calcium-based plasticity model for predicting long-term potentiation and depression in the neocortex, Nature Communications (2022). DOI: 10.1038/s41467-022-30214-w