Artificial intelligence tools quickly detect signs of injection drug use in patients' health records
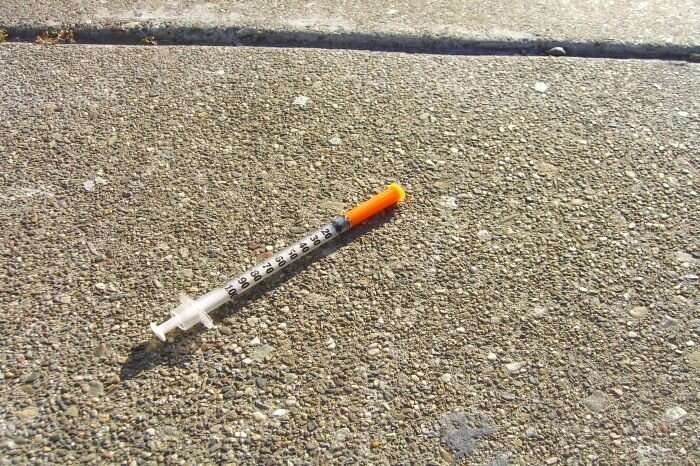
An automated process that combines natural language processing and machine learning identified people who inject drugs (PWID) in electronic health records more quickly and accurately than current methods that rely on manual record reviews.
Currently, people who inject drugs are identified through International Classification of Diseases (ICD) codes that are specified in patients' electronic health records by the health care providers or extracted from those notes by trained human coders who review them for billing purposes. But there is no specific ICD code for injection drug use, so providers and coders must rely on a combination of non-specific codes as proxies to identify PWIDs—a slow approach that can lead to inaccuracies.
The researchers manually reviewed 1,000 records from 2003–2014 of people admitted to Veterans Administration hospitals with Staphylococcus aureus bacteremia, a common infection that develops when the bacteria enters openings in the skin, such as those at injection sites. They then developed and trained algorithms using natural language processing and machine learning and compared them with 11 proxy combinations of ICD codes to identify PWIDs.
Limitations to the study include potentially poor documentation by providers. Also, the dataset used is from 2003 to 2014, but the injection drug use epidemic has since shifted from prescription opioids and heroin to synthetic opioids like fentanyl, which the algorithm may miss because the dataset where it learned the classification does not have many examples of that drug. Finally, the findings may not be applicable to other circumstances given that they are based entirely on data from the Veterans Administration.
Use of this artificial intelligence model significantly speeds up the process of identifying PWIDs, which could improve clinical decision making, health services research, and administrative surveillance.
"By using natural language processing and machine learning, we could identify people who inject drugs in thousands of notes in a matter of minutes compared to several weeks that it would take a manual reviewer to do this," said lead author Dr. David Goodman-Meza, assistant professor of medicine in the division of infectious diseases at the David Geffen School of Medicine at UCLA. "This would allow health systems to identify PWIDs to better allocate resources like syringe services programs and substance use and mental health treatment for people who use drugs."
The study is published in the peer-reviewed journal Open Forum Infectious Diseases.
More information: David Goodman-Meza et al, Natural Language Processing and Machine Learning to Identify People Who Inject Drugs in Electronic Health Records, Open Forum Infectious Diseases (2022). DOI: 10.1093/ofid/ofac471