This article has been reviewed according to Science X's editorial process and policies. Editors have highlighted the following attributes while ensuring the content's credibility:
fact-checked
peer-reviewed publication
trusted source
proofread
Machine learning helps to tackle long COVID
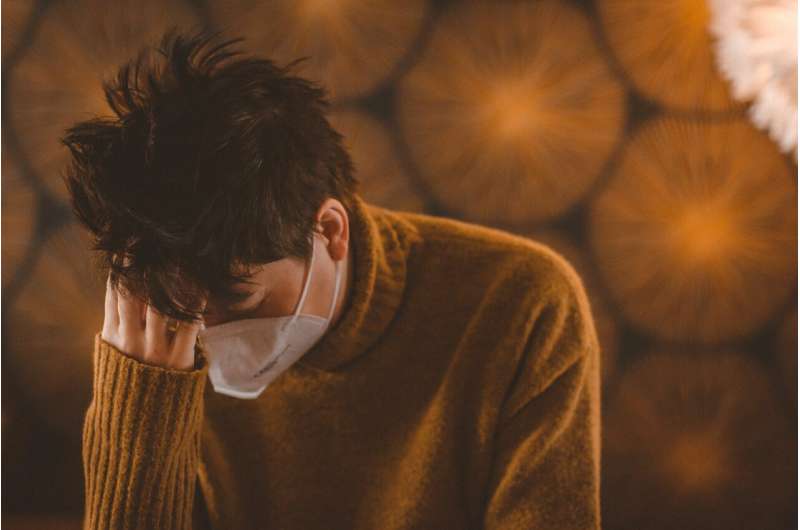
Long COVID has emerged as a pandemic within the pandemic. As scientists work to untangle the many remaining unanswered questions about how the initial infection impacts the body, they must now also investigate why some people develop debilitating, chronic symptoms that last months to years longer.
A new machine learning tool is here to help.
Developed by a team of researchers from institutions across the country, led by Justin Reese of Berkeley Lab and Peter Robinson of Jackson Lab, the software analyzes entries in electronic health records (EHRs) to find symptoms in common between people who have been diagnosed with long COVID and to define subtypes of the condition. The research, which is described in a new paper in eBioMedicine, also identifies strong correlations between different long COVID subtypes and pre-existing conditions such as diabetes and hypertension.
According to Reese, a computer research scientist in Berkeley Lab's Biosciences Area, this research will help improve our understanding of how and why some individuals develop long COVID symptoms, and may enable more effective treatments by helping clinicians develop tailored therapies for each group. For example, the best treatment for patients experiencing nausea and abdominal pain might be quite different from a treatment for those suffering from persistent cough and other lung symptoms.
The team developed and validated their software using a database of EHR information from 6,469 patients diagnosed with long COVID after confirmed COVID-19 infections. "Basically, we found long COVID features in the EHR data for each long COVID patient, and then assessed patient-patient similarity using semantic similarity, which essentially allows 'fuzzy matching' between features—for example, 'cough' is not the same as 'shortness of breath,' but they are similar since they both involve lung problems," Reese said. "We compare all symptoms for the pair of the patients in this way, and get a score of how similar the two long COVID patients are. We can then perform unsupervised machine learning on these scores to find different subtypes of long COVID."
The researchers applied machine learning to these patient-patient similarity scores to cluster patients into groups, which were then characterized by analyzing relationships between symptoms and pre-existing diseases and other demographic features, such as age, gender, or race.
Reese and his colleagues note that the tool will be convenient for researchers because the machine learning approach at its core self-adapts for different EHR systems, allowing researchers to gather data from a wide variety of medical establishments.
This research builds on previous work to develop the Human Phenotype Ontology, an open-access database and research tool that provides a standardized vocabulary of symptoms and features found in all human diseases.
More information: Justin T. Reese et al, Generalisable long COVID subtypes: Findings from the NIH N3C and RECOVER programmes, eBioMedicine (2022). DOI: 10.1016/j.ebiom.2022.104413