This article has been reviewed according to Science X's editorial process and policies. Editors have highlighted the following attributes while ensuring the content's credibility:
fact-checked
proofread
Deep-learning filter improves precision of cell mutation detection, accuracy of cancer diagnoses
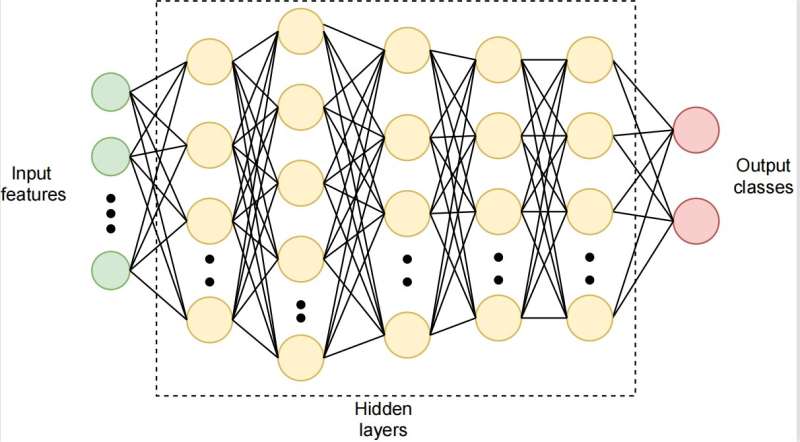
Next-generation cancer strategies rely on next-generation gene sequencing (NGS), which paves the way for new techniques and tools to detect mutations and determine patient therapy. A team of Chinese researchers proposed a more effective strategy to filter false positive results, which improves the accuracy and efficiency of cancer diagnosis and treatment.
The research team proposed DeepFilter, a deep-learning based filter for removing false positives in somatic variants in NGS data.
Their study was published on January 6, 2023 in Tsinghua Science and Technology.
Finding somatic mutations, or alterations in normal tissue, is key to understanding lethal genetic diseases of the human genome such as cancer. Next-generation gene sequencing accelerates the search for somatic mutations by employing technologies that separate DNA/RNA into multiple pieces and identify sequences in parallel, producing thousands or millions of sequences concurrently. This technique improves accuracy while reducing the cost and time of sequencing.
Powerful "calling tools" comb through NGS data and track down tumors or other mutations by comparing sequences to a reference genome from related tissue in the same individual.
VarDict is a somatic variant calling tool used commonly in clinical research. Previous studies have shown that VarDict achieves higher accuracy rates and detects more true variants than similar calling tools. However, VarDict also generates a higher number of false positives than other callers, which can skew results.
"An error rate of 1:10,000 in a genome with 3 billion positions would result in many false calls, which may lead to inaccurate clinical diagnoses," said Zekun Yin, a study author from Shandong University. "However, filtering true positives may also lead to missed diagnoses."
Typically, researchers filter out some of the false positives manually—an onerous, costly process that the Chinese research team set out to alleviate.
"It will save a lot of time and money if we provide an automatic method to effectively filter out most of the false positives," said Hao Zhang, a study author from Shandong University.
Inspired by recent successes integrating machine-learning based methods to call genetic variants from NGS data, the Chinese research team introduced a deep-learning based variant filter. Dubbed DeepFilter, the filter is designed to effectively sift through false positive variants generated by VarDict while also ensuring high calling sensitivity.
DeepFilter treats the task of distinguishing whether a variant is true or false as a binary classification problem. The researchers used three types of datasets to train and test DeepFilter: real-world tumor-normal sample data, a mixture of two golden-standard data, and synthetic data.
The experimental results based on both synthetic and real-world NGS data were promising:
"DeepFilter outperformed other filters in terms of false positive variant filter tasks, which made VarDict more valuable in practical clinical research and greatly facilitated downstream analysis in biological research and patient treatment," said Zhang.
The team plans to wade deeper into the problem of false-positive variant filtering, looking specifically at the positive and negative sample imbalance problem and incorporating other machine learning and deep-learning methods for filtering.
"Our ultimate goal is to solve the problem of running efficiency and accuracy of variation calling and provide a state-of-the-art variation detection tool," said Yin.
More information: Hao Zhang et al, DeepFilter: A Deep Learning Based Variant Filter for VarDict, Tsinghua Science and Technology (2023). DOI: 10.26599/TST.2022.9010032