This article has been reviewed according to Science X's editorial process and policies. Editors have highlighted the following attributes while ensuring the content's credibility:
fact-checked
peer-reviewed publication
trusted source
proofread
A new model to identify and predict chronic pain
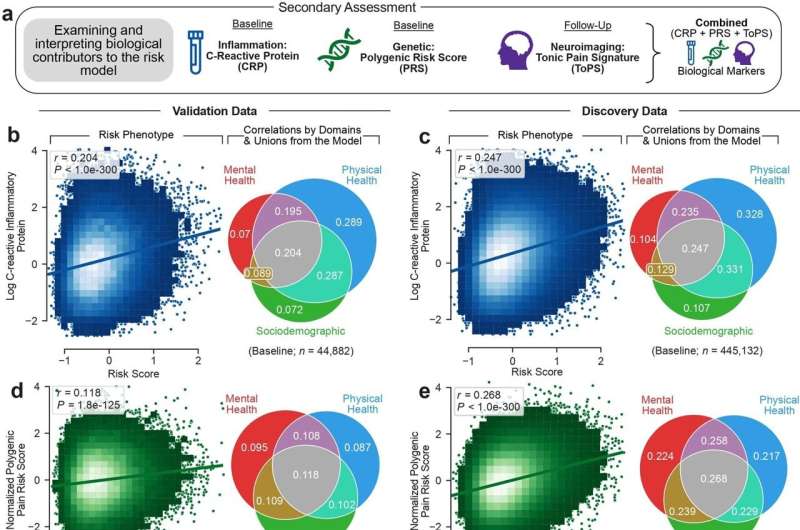
Chronic pain is a complex condition that affects millions of individuals worldwide and understanding its causes and predicting its trajectory remains difficult. However, findings from a recent McGill-led study published in Nature Medicine could improve the understanding and management of chronic pain.
The researchers developed a model that can predict one's likelihood of developing chronic pain as well as the severity and spread of that pain. The study also identified several key risk factors associated with increased chronic pain, including sleeplessness, feelings of distress, fatigue, stressful life events, and a body mass index (BMI) above 30.
Using data from the UK Biobank, researchers found that pain tends to spread from one area of the body to another. They developed a model that can predict the number of different pain sites a person may have. With the help of machine-learning, this risk score can distinguish long-lasting pain and predict whether someone will develop widespread chronic pain, how the pain will spread across their body, and whether the pain will be severe.
The implications of this study are far-reaching. "By identifying common biopsychosocial factors associated with chronic pain, health care professionals could better personalize treatment plans and improve patient outcomes," says Dr. Etienne Vachon-Presseau, Assistant Professor in the Faculty of Dental Medicine and Oral Health Sciences and co-author of the study.
More information: Christophe Tanguay-Sabourin et al, A prognostic risk score for development and spread of chronic pain, Nature Medicine (2023). DOI: 10.1038/s41591-023-02430-4