This article has been reviewed according to Science X's editorial process and policies. Editors have highlighted the following attributes while ensuring the content's credibility:
fact-checked
peer-reviewed publication
trusted source
proofread
AI transformation of medicine: Why doctors are not prepared
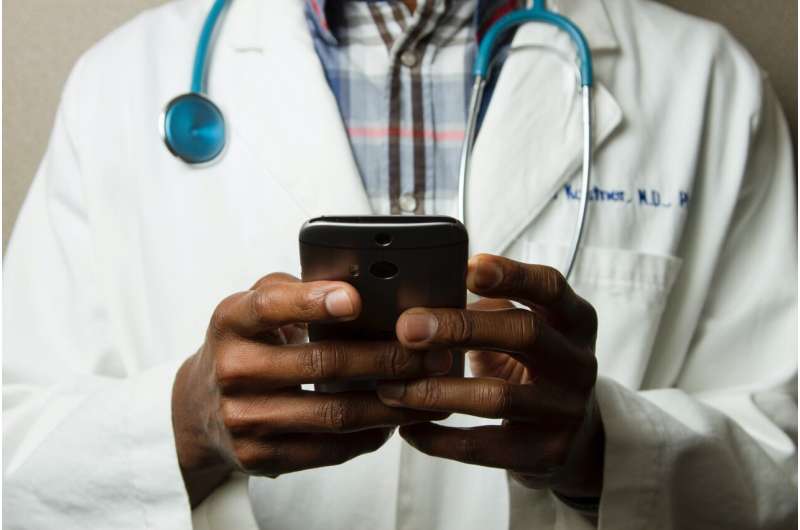
As artificial intelligence systems like ChatGPT find their way into everyday use, physicians will start to see these tools incorporated into their clinical practice to help them make important decisions on diagnosis and treatment of common medical conditions. These tools, called clinical decision support (CDS) algorithms, can be enormously helpful in helping guide health care providers in determining, for example, which antibiotics to prescribe or whether to recommend a risky heart surgery.
The success of these new technologies, however, depends largely on how physicians interpret and act upon a tool's risk predictions—and that requires a unique set of skills that many are currently lacking, according to a new perspective article published in the New England Journal of Medicine that was written by faculty in the University of Maryland School of Medicine (UMSOM).
CDS algorithms, which make predictions under conditions of clinical uncertainty, can include everything from regression-derived risk calculators to sophisticated machine learning and artificial intelligence-based systems. They can be used to predict which patients are most likely to go into life-threatening sepsis from an uncontrolled infection or which therapy has the highest probability of preventing sudden death in an individual heart disease patient.
"These new technologies have the potential to significantly impact patient care, but doctors need to first learn how machines think and work before they can incorporate algorithms into their medical practice," said Daniel Morgan, MD, MS, Professor of Epidemiology & Public Health at UMSOM and co-author of the perspective.
While some clinical decision support tools are already incorporated into electronic medical record systems, health care providers often find the current software to be cumbersome and difficult to use.
"Doctors don't need to be math or computer experts, but they do need to have a baseline understanding of what an algorithm does in terms of probability and risk adjustment, but most have never been trained in those skills," said Katherine Goodman, JD, Ph.D., Assistant Professor of Epidemiology & Public Health at UMSOM and co-author of the perspective.
To address this gap, medical education and clinical training need to incorporate explicit coverage of probabilistic reasoning tailored specifically to CDS algorithms. Drs. Morgan, Goodman, and their co-author Adam Rodman, MD, MPH, at Beth Israel Deaconess Medical Center in Boston, proposed the following:
- Improve probabilistic skills: Early in medical school, students should learn the fundamental aspects of probability and uncertainty and use visualization techniques to make thinking in terms of probability more intuitive. This training should include interpreting performance measures like sensitivity and specificity to better understand test and algorithm performance.
- Incorporate algorithmic output into decision making: Physicians should be taught to critically evaluate and use CDS predictions in their clinical decision-making. This training involves understanding the context in which algorithms operate, recognizing limitations, and considering relevant patient factors that algorithms may have missed.
- Practice interpreting CDS predictions in applied learning: Medical students and physicians can engage in practice-based learning by applying algorithms to individual patients and examining how different inputs affect predictions. They should also learn to communicate with patients about CDS-guided decision making.
The University of Maryland, Baltimore (UMB), University of Maryland, College Park (UMCP) and University of Maryland Medical System (UMMS) recently launched plans for a new Institute for Health Computing (IHC).
The UM-IHC will leverage recent advances in artificial intelligence, network medicine, and other computing methods to create a premier learning health care system that evaluates both de-identified and secure digitized medical health data to enhance disease diagnosis, prevention, and treatment.
Dr. Goodman is beginning a position at IHC, which will be a site that is dedicated to educating and training health care providers on the latest technologies. The Institute plans to eventually offer a certification in health data science among other formal educational opportunities in data sciences.
"Probability and risk analysis is foundational to the practice of evidence-based medicine, so improving physicians' probabilistic skills can provide advantages that extend beyond the use of CDS algorithms," said UMSOM Dean Mark T. Gladwin, MD, Vice President for Medical Affairs, University of Maryland, Baltimore, and the John Z. and Akiko K. Bowers Distinguished Professor.
"We're entering a transformative era of medicine where new initiatives like our Institute for Health Computing will integrate vast troves of data into machine learning systems to personalize care for the individual patient."
More information: Katherine E. Goodman et al, Preparing Physicians for the Clinical Algorithm Era, New England Journal of Medicine (2023). DOI: 10.1056/NEJMp2304839