This article has been reviewed according to Science X's editorial process and policies. Editors have highlighted the following attributes while ensuring the content's credibility:
fact-checked
proofread
Machine learning informs a new tool to guide treatment for acute decompensated heart failure
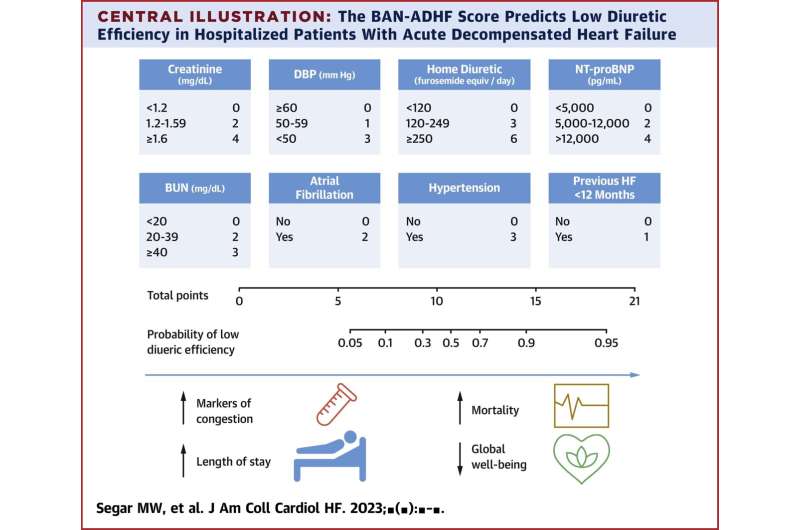
A recent study co-authored by Dr. Matthew Segar, a third-year cardiovascular disease fellow at The Texas Heart Institute and led by his research and residency mentor, University of Texas Southwestern Medical Center's Dr. Ambarish Pandey, utilized a machine learning-based approach to identify, understand, and predict diuretic responsiveness in patients with acute decompensated heart failure (ADHF).
The study, "A Phenomapping Tool and Clinical Score to Identify Low Diuretic Efficiency in Acute Decompensated Heart Failure," published in JACC: Heart Failure, leverages decades of clinical and registry datasets.
The researchers leveraged machine-learning-based approaches to develop a prediction tool called the BAN-ADHF score, which showed promising results in accurately predicting diuretic response. After validation in other clinical populations, implementing this tool could potentially lead to personalized strategies for effectively managing the congestion of patients hospitalized with ADHF.
There remains a lack of agreement among experts regarding the most effective approach to address diuretic resistance in patients with heart failure who are stable hemodynamically and have an excess volume of fluid. It is generally recommended to optimize the dosage of loop diuretics before considering combination therapy; however, there is no consensus on how much the dosage should be increased before introducing another diuretic.
"Inefficient diuretic response in hospitalized patients can hinder treatment progress and increase the risk of post-discharge rehospitalization and mortality. It's crucial to identify individuals with low diuretic efficiency early on to tailor decongestion strategies and improve clinical outcomes," according to Dr. Segar.
ADHF is a public health issue that is becoming increasingly concerning. The disease results in emergency room visits, hospital admissions, and associated high health care costs. ADHF is characterized by the body having too much fluid, which often requires hospitalization or changing a patient's current treatment plan.
"Today, a primary goal of treating ADHF is to relieve congestion using loop diuretic drugs. However, there is still uncertainty about the best dose of these agents to administer. Additionally, because of the heterogeneity of ADHF patients, a more personalized approach to predicting optimal dosing strategies is needed," said Dr. Joseph G. Rogers, President and CEO of The Texas Heart Institute.
In the study, researchers from institutions across the United States utilized machine learning (ML) algorithms to identify subgroups of patients with acute heart failure based on their responsiveness to diuretic therapy. Specifically, the researchers developed a diuretic efficiency phenomapping approach for patients with ADHF by using publicly available and deidentified data from several clinical trials and registries, including DOSE, ROSE-AHF, CARRESS-HF, ATHENA-HF, ESCAPE, and the American Heart Association Precision Medicine Platform Get with the Guidelines-HF (GWTG-HF) registry.
This participant-level pooled data enabled the investigators to develop a phenomapping approach and diuretic efficiency score. The patients within each subgroup shared similar characteristics but were clinically distinct from other subgroups, particularly in their response to diuretic therapy.
In addition to differences in their diuretic response, the patient subgroups also had meaningfully different clinical outcomes, highlighting the prognostic utility of the phenogrouping approach. The investigators subsequently developed and validated the BAN-ADHF score to predict the probability of being in the phenogroup with the least diuretic response.
"We know the BAN-ADHF score can accurately identify, characterize, and predict diuretic resistance among individuals with ADHF mathematically. Now we must take this medical knowledge and conduct a clinical study to evaluate whether implementing the BAN-ADHF score in our care protocols improves outcomes for patients hospitalized with acute decompensated heart failure," said Dr. Segar.
More information: Matthew W. Segar et al, A Phenomapping Tool and Clinical Score to Identify Low Diuretic Efficiency in Acute Decompensated Heart Failure, JACC: Heart Failure (2023). DOI: 10.1016/j.jchf.2023.09.029