Cancer's many faces examined in new study
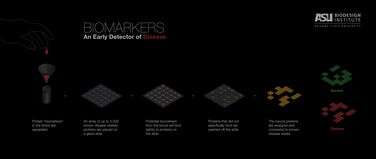
(Medical Xpress)—This year, according to the American Cancer Society, some 232,340 new cases of invasive breast cancer will be diagnosed in women. Some of these patients will succumb to the disease, while others survive and live healthy lives for decades.
Part of the enormous disparity in outcome has to do with the differing ways diseases like cancer affect individuals based on age, ethnicity, lifestyle, environmental conditions, genetic predisposition and other factors.
According to Garrick Wallstrom, a researcher at Arizona State University's Biodesign Institute, how we study an illness can also depend on a feature of the disease itself – one known as heterogeneity.
Heterogeneous diseases are those composed of multiple molecular subgroups - each producing distinct manifestations of illness - differing in severity, prognosis and recurrence. Breast cancer is one such example of a heterogeneous disease.
"Our ability to differentiate and understand subgroups of disease is fundamental to personalized medicine," Wallstrom says. "But disease heterogeneity presents a real challenge in medical research because a set of patients in a study may actually have very different diseases at the molecular level. What we've shown is that researchers need to carefully consider heterogeneity early on, when they are designing their studies."
In new research appearing in the journal Cancer Epidemiology, Biomarkers and Prevention, Wallstrom and colleagues evaluate the statistical reliability of biomarkers – protein factors used to pinpoint the presence of disease at an early, pre-symptomatic stage. Their work reveals for the first time that disease heterogeneity profoundly affects biomarker performance.
While multiple subtypes of diseases like breast cancer have long been recognized, the implications for biomarker discovery and validation have not been systematically evaluated prior to the current study. Wallstrom and his colleagues determined that a two-fold larger sample size is typically required to establish strong biomarker candidates for heterogeneous diseases, compared with monotypic diseases (those with just a single underlying molecular pathology).
The study also established that specific statistical tests used to screen biomarkers differ markedly in their predictive reliability, depending on whether the disease under study is monotypic or heterogeneous.
The work has implications for the design of experiments aimed at identifying new biomarkers, as well as for drug-discovery studies and drug trials. (Certain anti-cancer drugs are already recognized for their preferential effectiveness depending on disease subtype. Herceptin for example, is an effective drug for breast cancer patients who test positive for the HER-2/neu biomarker. For others, it is ineffective.)
A persistent scourge
Among women, breast cancer is the most frequently occurring malignancy and the second leading cause of cancer-related death in the United States. About one in eight women in the US (12 percent) will develop invasive breast cancer during their lifetime. Currently, there are no validated plasma/serum biomarkers for the disease. Only a few biomarkers (such as HER-2/neu, estrogen receptor and progesterone receptor) have so far shown clinical effectiveness for diagnosis and prognosis. The need for new diagnostic biomarkers is therefore acute.
Mammography remains the most effective clinical screening method for breast cancer, though lesions less than .5 cm in size remain undetectable. Further, mammography has a fairly low ratio of sensitivity to specificity. This accounts for the fact that roughly four times as many women undergo biopsy for benign breast lesions as those with actual malignancy.
Detecting breast cancer at a preinvasive state offers the best hope for controlling malignancy, as it provides clinical options, including surgical resection, before the disease has undergone metastasis. Expanding the pool of biomarker candidates and validating them for clinical use is therefore a central mission for cancer diagnosticians.
Changing paradigm
The emerging picture presented in the new study is one in which each disease subtype possesses its own set of unique biomarkers. A given biomarker may therefore be highly effective at detecting a particular subtype of the disease while displaying very low sensitivity for another subtype. Depending on the molecular nature of a patient's disease, the biomarker may or may not be diagnostically useful. Ensuring that all relevant subtypes of a disease are represented in a screening study thus requires much larger sample sizes.
Wallstrom's group used a statistical method known as Monte Carlo simulation to compare the performance of eight selection methods commonly used to identify cancer biomarkers. To theoretically assess the effect of disease heterogeneity on biomarker selection, the eight methods were applied to both monotypic and heterogeneous diseases using single-stage and two-stage designs. Next, the group applied the chosen selection methods to an actual biomarker screening study of heterogeneous breast cancer cases.
Traditionally, protein biomarkers are established through the examination of a large pool of candidates, using disease-positive cases and disease-negative controls. When the number of candidate biomarkers to be screened is very large, a two-stage strategy may be used. In this case, stage one reduces the biomarker library to a manageable number of best cases using a moderate number of patients and controls, while stage two further narrows the biomarker pool using the remaining patients and controls.
The new study examined the statistical power of each selection method. (Statistical power measures the likelihood that the test will detect a particular effect when such an effect exists.) In the case of homogeneous diseases, biomarker performance depends on small distributional shifts between healthy and disease-positive cases. For heterogeneous diseases however, a large statistical signal is observed in a small subpopulation of cases.
For larger studies, two-stage selection methods proved the most efficient, providing nearly the same statistical power as single-stage studies, at significantly lower cost. In both single- and two-stage studies, Wallstrom considered a pool of 10,000 candidate biomarkers. Roughly twice as many cases and controls were required for heterogeneous disease, and the most effective of the eight statistical screening methods differed for monotypic and heterogeneous disease.
In follow-up experiments, the group compared the performance of the eight selection methods for an actual breast cancer screening study. Blood samples from 102 early stage breast cancer patients and 77 controls were used to screen 761 antigens. The experiments were carried out using a microarray technology known as NAPPA, developed by Joshua LaBaer, who directs the Biodesign Institute's Virginia G. Piper Center for Personalized Diagnostics. (With NAPPA, DNA templates are printed on the microarray slide, allowing proteins to be expressed at the time of experiment, rather than laboriously purified beforehand.)
Revising tactics for diagnosis
Results of the new research clearly underline the fact that selection of an optimal screening method for biomarker discovery is critically dependent on the monotypic or heterogeneous nature of the disease in question. For example, a method known as PAUC produced poor results for homogeneous disease, but delivered the best results of the eight methods for heterogeneous diseases, given large sample sizes. Similarly, the Mann-Whitney and AUC tests produced good results for homogenous diseases, but very poor results for heterogeneous disease.
The research demonstrates that 70 percent statistical power may be achieved with 50 cases and 50 controls, provided the disease is monotypic. For heterogeneous diseases like breast cancer however, the same sample sizes yielded only 15 percent power – much too low to be useful. In fact, twice as many samples were required for heterogeneous diseases to achieve the same statistical power.
The authors stress that the study's intention was not to find the single, optimal method of biomarker screening, but instead to underscore the decisive role played by disease heterogeneity for biomarker screening. They further suggest that an evaluation of a method's statistical power should be integral to the design of future screening studies.