New modeling framework developed to improve infectious disease control
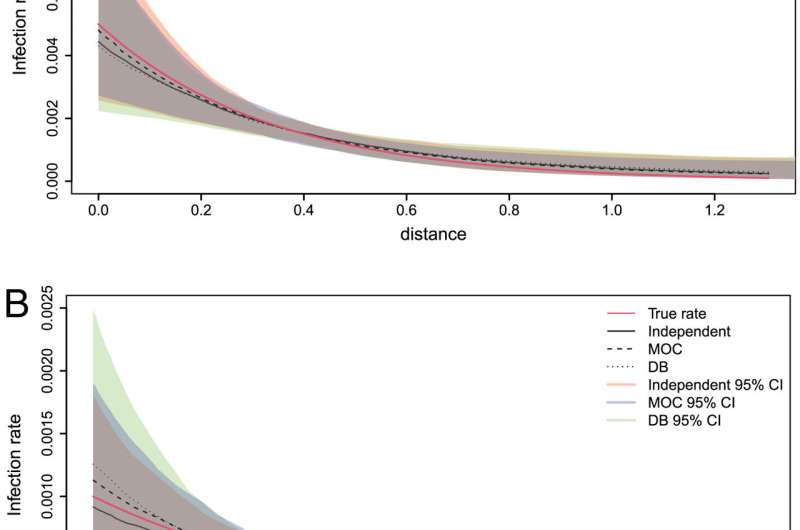
A new model to analyze infectious disease outbreak data has been developed by mathematicians that could be used to improve disease tracking and control.
Researchers from the University of Nottingham developed a new data-driven framework for modeling how infectious diseases spread through a population that could reduce errors in decisions made about disease control measures. Their findings have been published in PNAS.
The COVID-19 pandemic has highlighted that the ability to unravel the dynamics of the spread of infectious diseases is profoundly important for designing effective control strategies and assessing existing ones. Mathematical models of how infectious diseases spread continue to play a vital role in understanding, mitigating, and preventing outbreaks.
Dr. Rowland Seymour led the study and explains: "Most of the infectious disease models contain specific assumptions about how transmission occurs within a population. These assumptions can be arbitrary, particularly when it comes to describing how transmission varies between individuals of different types or in different locations and can be lacking in appropriate biological or epidemiological justification. this can lead to erroneous scientific conclusions and misleading predictions. Infectious diseases both within human and animal populations continue to pose serious health and socioeconomic risks. We have developed a suite of contemporary statistical methods that dispenses with the need for the underlying transmission assumptions of existing models. Our approach enables instead the analysis to be driven by evidence in the data and hence allowing policy makers to make data-driven decisions about controlling the spread of a disease. Our work is another tool in the fight against the spread of infectious diseases and we are excited to develop this framework further."
The researchers developed a data-driven framework for modeling how infectious diseases spread through a population by avoiding strict modeling assumptions which are often difficult to justify. The researchers used the method to enhance understanding of the 2001 UK Foot and Mouth outbreak in which over 6 million animals were culled with a cost to the public and private purse of over £8 billion.
The proposed methodology is very general making it applicable to a wide class of models, including those which take into account the population's structure (e.g. households, workplaces) and individual's characteristics (e.g. location and age).
This work has opened several avenues for further research in this area, including improving its computationally efficiency and being applicable in real-time, i.e. when the outbreak is still ongoing. The latter is of material importance for policy makers and government authorities, so they can be responsive to the data that is emerging from the outbreak.
More information: Rowland G. Seymour et al, Bayesian nonparametric inference for heterogeneously mixing infectious disease models, Proceedings of the National Academy of Sciences (2022). DOI: 10.1073/pnas.2118425119