This article has been reviewed according to Science X's editorial process and policies. Editors have highlighted the following attributes while ensuring the content's credibility:
fact-checked
peer-reviewed publication
trusted source
proofread
Researchers use AI to triage patients with chest pain
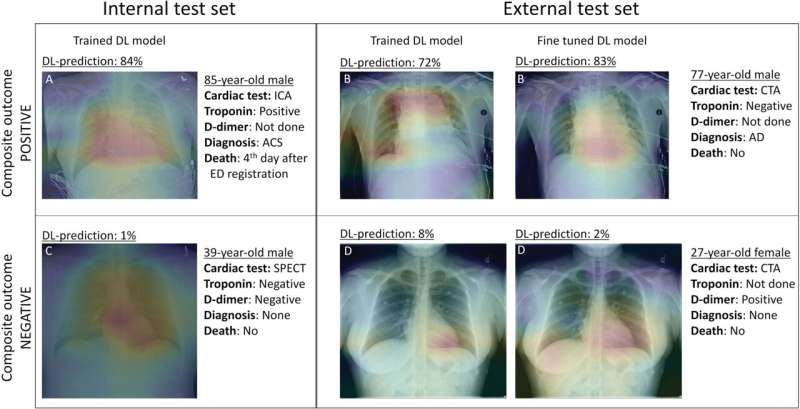
Artificial intelligence (AI) may help improve care for patients who show up at the hospital with acute chest pain, according to a study published in Radiology.
"To the best of our knowledge, our deep learning AI model is the first to utilize chest X-rays to identify individuals among acute chest pain patients who need immediate medical attention," said the study's lead author, Márton Kolossváry, M.D., Ph.D., radiology research fellow at Massachusetts General Hospital (MGH) in Boston.
Acute chest pain syndrome may consist of tightness, burning or other discomfort in the chest or a severe pain that spreads to your back, neck, shoulders, arms, or jaw. It may be accompanied by shortness of breath.
Acute chest pain syndrome accounts for over 7 million emergency department visits annually in the United States, making it one of the most common complaints.
Fewer than 8% of these patients are diagnosed with the three major cardiovascular causes of acute chest pain syndrome, which are acute coronary syndrome, pulmonary embolism or aortic dissection. However, the life-threatening nature of these conditions and low specificity of clinical tests, such as electrocardiograms and blood tests, lead to substantial use of cardiovascular and pulmonary diagnostic imaging, often yielding negative results.
As emergency departments struggle with high patient numbers and shortage of hospital beds, effectively triaging patients at very low risk of these serious conditions is important.
Deep learning is an advanced type of artificial intelligence (AI) that can be trained to search X-ray images to find patterns associated with disease.
For the study, Dr. Kolossváry and colleagues developed an open-source deep learning model to identify patients with acute chest pain syndrome who were at risk for 30-day acute coronary syndrome, pulmonary embolism, aortic dissection or all-cause mortality, based on a chest X-ray.
The study used electronic health records of patients presenting with acute chest pain syndrome who had a chest X-ray and additional cardiovascular or pulmonary imaging and/or stress tests at MGH or Brigham and Women's Hospital in Boston between January 2005 and December 2015. For the study, 5,750 patients (mean age 59, including 3,329 men) were evaluated.
The deep-learning model was trained on 23,005 patients from MGH to predict a 30-day composite endpoint of acute coronary syndrome, pulmonary embolism or aortic dissection and all-cause mortality based on chest X-ray images.
The deep-learning tool significantly improved prediction of these adverse outcomes beyond age, sex and conventional clinical markers, such as d-dimer blood tests. The model maintained its diagnostic accuracy across age, sex, ethnicity and race. Using a 99% sensitivity threshold, the model was able to defer additional testing in 14% of patients as compared to 2% when using a model only incorporating age, sex, and biomarker data.
"Analyzing the initial chest X-ray of these patients using our automated deep learning model, we were able to provide more accurate predictions regarding patient outcomes as compared to a model that uses age, sex, troponin or d-dimer information," Dr. Kolossváry said. "Our results show that chest X-rays could be used to help triage chest pain patients in the emergency department."
According to Dr. Kolossváry, in the future such an automated model could analyze chest X-rays in the background and help select those who would benefit most from immediate medical attention and may help identify patients who may be discharged safely from the emergency department.
More information: Márton Kolossváry et al, Deep Learning Analysis of Chest Radiographs to Triage Patients with Acute Chest Pain Syndrome, Radiology (2023). DOI: 10.1148/radiol.221926
dx.doi.org/Jin Mo Goo, Triaging: Another Vital Application of the Deep Learning Technique on Chest Radiographs at the Emergency Department, Radiology (2023). dx.doi.org/10.1148/radiol.223112