This article has been reviewed according to Science X's editorial process and policies. Editors have highlighted the following attributes while ensuring the content's credibility:
fact-checked
peer-reviewed publication
trusted source
proofread
Using machine learning to predict brain tumor progression
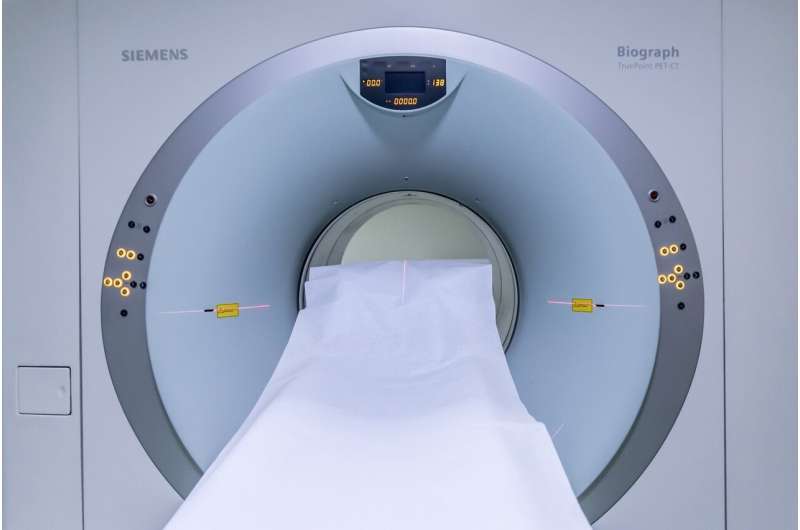
Researchers at the University of Waterloo have created a computational model to predict the growth of deadly brain tumours more accurately.
Glioblastoma multiforme (GBM) is a brain cancer with an average survival rate of only one year. It is difficult to treat due to its extremely dense core, rapid growth, and location in the brain. Estimating these tumours' diffusivity and proliferation rate is useful for clinicians, but that information is hard to predict for an individual patient quickly and accurately.
Researchers at the University of Waterloo and the University of Toronto have partnered with St. Michael's Hospital in Toronto to analyze MRI data from multiple GBM sufferers. They're using machine learning to fully analyze a patient's tumour, to better predict cancer progression.
Researchers analyzed two sets of MRIs from each of five anonymous patients suffering from GBM. The patients underwent extensive MRIs, waited several months, and then received a second set of MRIs. Because these patients, for undisclosed reasons, chose not to receive any treatment or intervention during this time, their MRIs provided the scientists with a unique opportunity to understand how GBM grows when left unchecked.
The researchers used a deep learning model to turn the MRI data into patient-specific parameter estimates that inform a predictive model for GBM growth. This technique was applied to patients' and synthetic tumours, for which the true characteristics were known, enabling them to validate the model.
"We would have loved to do this analysis on a huge data set," said Cameron Meaney, a Ph.D. candidate in Applied Mathematics and the study's lead researcher. "Based on the nature of the illness, however, that's very challenging because there isn't a long life expectancy, and people tend to start treatment. That's why the opportunity to compare five untreated tumours was so rare—and valuable."
Now that the scientists have a good model of how GBM grows untreated, their next step is to expand the model to include the effect of treatment on the tumours. Then the data set would increase from a handful of MRIs to thousands.
Meaney emphasizes that access to MRI data—and partnership between mathematicians and clinicians—can have huge impacts on patients going forward.
"The integration of quantitative analysis into healthcare is the future," Meaney said.
The study co-authored by Meaney, Sunit Das, Errol Colak, and Mohammad Kohandel, appears in the Journal of Theoretical Biology.
More information: Cameron Meaney et al, Deep learning characterization of brain tumours with diffusion weighted imaging, Journal of Theoretical Biology (2022). DOI: 10.1016/j.jtbi.2022.111342