This article has been reviewed according to Science X's editorial process and policies. Editors have highlighted the following attributes while ensuring the content's credibility:
fact-checked
trusted source
proofread
How AI could help optimize nutrient consistency in donated human breast milk
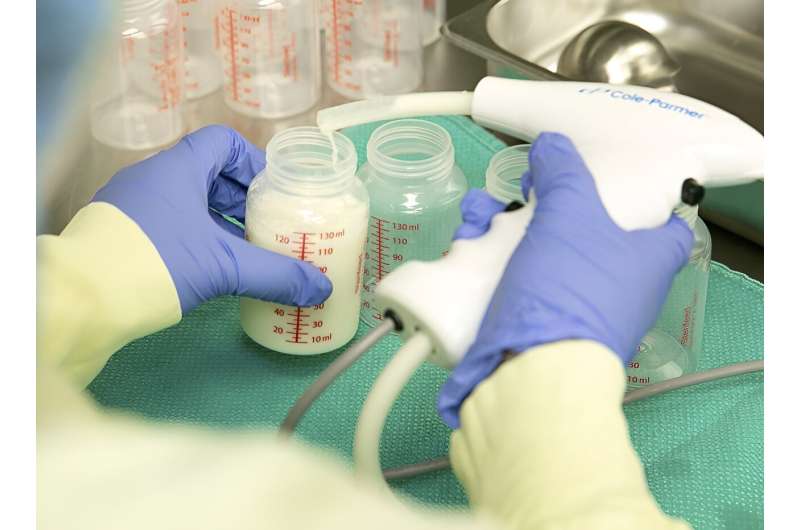
A team of University of Toronto Engineering researchers, led by Professor Timothy Chan, is leveraging machine learning to optimize the macronutrient content of pooled human donor milk recipes.
The researchers introduce their data-driven optimization model in a new paper published in Manufacturing and Systems Operations Management.
Chan and his team worked with Mount Sinai Hospital's Rogers Hixon Ontario Human Milk Bank—which provides donor milk to preterm and sick babies who are hospitalized across Ontario—as well as Dr. Debbie O'Connor, a professor at the Temerty Faculty of Medicine.
"For a variety of reasons, many hospitalized infants do not have a full supply of mother's milk. In this instance human donor milk can be lifesaving particularly as it helps to protect preterm infants from necrotizing enterocolitis, a life-threatening bowel disease," says Dr. Sharon Unger, a neonatologist and the medical director of the Rogers Hixon Ontario Human Milk Bank.
"The new program developed by Dr. Chan helps to ensure that each batch of human donor milk meets the protein and calorie needs of preterm infants."
Currently, many milk banks, including Mount Sinai's, rely on individual decision making when pooling donor milk. This presents a significant challenge in producing a consistent donor milk product that contains sufficient macronutrients for premature and sick babies in neonatal intensive care units.
"It takes a lot of time to create these recipes without a defined method," says Chan.
"While there are studies that show that milk that comes from donors who are early in their postpartum period tends to be more protein rich, our approach provides a good prediction of the actual macronutrient content that will allow milk bank employees to make better pooling decisions."
Given that milk banks are often non-profit entities operating on lean budgets, a low-cost alternative to obtaining a consistent, nutrient-balanced product could be useful across the entire sector.
Devices known as human milk analyzers can be used to measure the exact macronutrient content of each milk sample at a milk bank. However, these devices are costly and require extensive regulatory approval for use, with the result that only half of all milk banks in North America use one. On top of that, analyzing every donation is a costly endeavor that is labor and resource intensive.
"Our data-driven framework bypasses the need for a device to analyze the donor milk by using an artificial intelligence model to predict the macronutrient content of each donation," says Rachel Wong, a lead researcher of the study.
"In addition, by using an optimization model to choose which donations to pool together, we can increase the consistency of macronutrient content in the donor milk product."
The multi-phased study included a one-year implementation trial at the Rogers Hixon milk bank that was designed to test whether AI-informed models could help to fill the gap.
In the first phase, researchers collected the necessary data to create a machine learning model to predict the macronutrient content of the pooled recipes, and then designed an optimization model to create the recipes based on macronutrient requirements, that is, the necessary levels of protein and fat.
The team then created a simulation model to test the method before embarking on an experiment in the milk bank, which took place over 16 months in 2021 and 2022.
"Since our study was performed in the milk bank during regular operating hours, rather than in a controlled environment, there were a number of unexpected challenges that we had to adapt to," says Wong.
"During the COVID-19 pandemic, the volume of donations fluctuated based on the provincial restrictions—during the lockdown periods there was an unprecedented increase in the number and volume of donations.
"We also needed to adapt the AI decisions that had already been proposed to ensure that we abided with the milk bank's operating protocols."
The last phase of the study began by observing the milk bank's operation for six months and measuring the fat, protein and bacteria levels in the pooled recipes.
For the following six months, the milk bank used the data-driven optimization framework to create the pooled milk recipes. At the end of the year, the researchers compared the optimized recipes to the previous ones to assess which recipes met the macronutrient targets.
"We found that our pooled recipes met the bar for protein and fat simultaneously up to 75% more often, without compromising other factors like an increase in bacteria," says Chan. "And it took us 60% less time to make the recipes."
The team's optimized recipes also have an added benefit for pre-term and sick babies, who have underdeveloped digestive systems that make it especially crucial to ensure that the milk they are consuming isn't overly rich in protein or fat.
Chan's team is currently working towards expanding this research to measure other nutrients in human donor milk to see if their models can optimize them. The research has won INFORMS's 2023 Pierskalla Best Paper Award and an Excellence in Quality and Safety award from Sinai Health.
"Our ultimate goal is to show that our tool is applicable to other milk banks," says Chan. "We would like to design a system that can plug into hospital systems to optimize recipes in a way that is sustainable for milk bank staff."
Wong says that the entire team is grateful to all those who have made the project possible.
"We couldn't have done this without all of the mothers who donate to the milk bank and the staff who work incredibly hard to provide donor milk to infants across Ontario and beyond," she says.
"I hope that this research will provide a framework to help milk banks across North America increase the consistency of macronutrient content in their donor milk product. The eventual end goal would be to see a downstream impact of improved growth and developmental outcomes for the infants that receive this donor milk."
More information: Timothy C. Y. Chan et al, Got (Optimal) Milk? Pooling Donations in Human Milk Banks with Machine Learning and Optimization, Manufacturing & Service Operations Management (2023). DOI: 10.1287/msom.2022.0455