This article has been reviewed according to Science X's editorial process and policies. Editors have highlighted the following attributes while ensuring the content's credibility:
fact-checked
trusted source
proofread
An AI strategy for identifying new immunotherapy targets
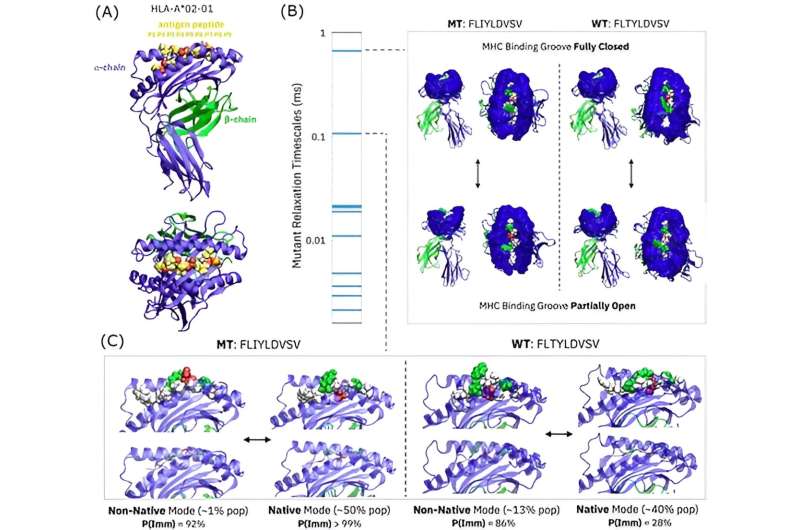
Researchers from Cleveland Clinic and IBM have published a strategy for identifying new targets for immunotherapy through artificial intelligence (AI). This is the first peer-reviewed publication from the two organizations' Discovery Accelerator partnership, designed to advance research in health care and life sciences.
The team worked together to develop supervised and unsupervised AI to reveal the molecular characteristics of peptide antigens, small pieces of protein molecules immune cells use to recognize threats. Project members came from diverse groups led by Cleveland Clinic's Timothy Chan, M.D., Ph.D., as well as IBM's Jeff Weber, Ph.D., Senior Research Scientist, and Wendy Cornell, Ph.D., Manager and Strategy Lead for Healthcare and Life Sciences Accelerated Discovery .
"In the past, all our data on cancer antigen targets came from trial and error," says Dr. Chan, chair of Cleveland Clinic's Center for Immunotherapy and Precision Immuno-Oncology and Sheikha Fatima Bint Mubarak Endowed Chair in Immunotherapy and Precision Immuno-Oncology. "Partnering with IBM allows us to push the boundaries of artificial intelligence and health sciences research to change the way we develop and evaluate targets for cancer therapy."
For decades, scientists have been researching how to better identify antigens and use them to attack cancer cells or cells infected with viruses. This task has proved challenging because antigen peptides interact with immune cells based on specific features on the surface of the cells, a process that is still not well understood.
Research has been limited by the sheer number of variables that affect how immune systems recognize these targets. Identifying these variables is difficult and time-intensive with regular computing, so current models are limited and at times inaccurate.
Published in Briefings in Bioinformatics, the study found that AI models that account for changes in molecular shape over time can accurately depict how immune systems recognize a target antigen. Through these models, researchers could home in on what processes are critical to target with immunotherapy treatments such as vaccines and engineered immune cells.
Researchers can incorporate these insights into other AI models moving forward to identify more effective immunotherapy targets.
"These discoveries are an example of what makes this partnership successful—combining IBM's cutting-edge computational resources with Cleveland Clinic's medical expertise," Dr. Weber says. "These findings resulted from a key collaboration between everyone from a world-class expert in cancer immunotherapy to our physics-based simulation and AI experts. Collaboration when combined with innovation has terrific potential."
More information: Jeffrey K Weber et al, Unsupervised and supervised AI on molecular dynamics simulations reveals complex characteristics of HLA-A2-peptide immunogenicity, Briefings in Bioinformatics (2024). DOI: 10.1093/bib/bbad504