This article has been reviewed according to Science X's editorial process and policies. Editors have highlighted the following attributes while ensuring the content's credibility:
fact-checked
proofread
How AI could improve speed, accuracy of response to infectious disease outbreaks in hospitals and even prevent them
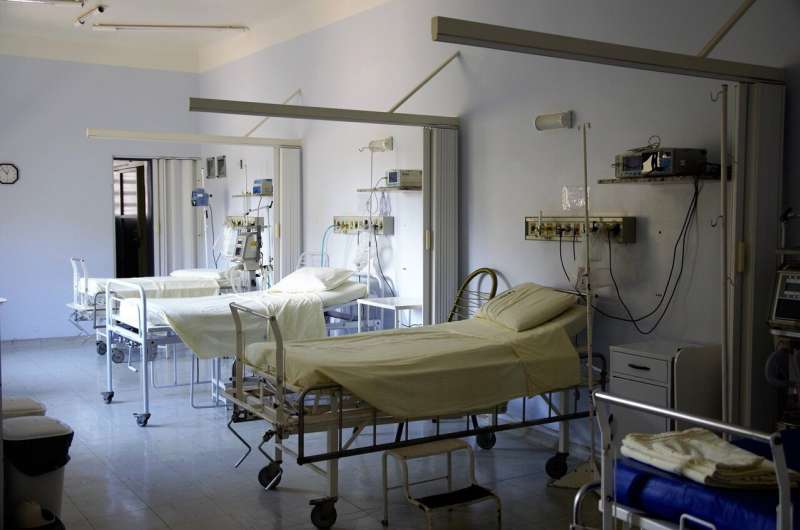
A new research review to be given at a pre-congress day for this year's European Congress of Clinical Microbiology and Infectious Diseases (ECCMID 2024) will highlight the potential artificial intelligence (AI) has to improve the speed and accuracy of investigations into infectious disease outbreaks in hospitals, and potentially provide real-time information to stop or prevent them. The talk will be by Dr. Jonas Marschall, Division of Infectious Diseases, Washington University School of Medicine, St. Louis, MO, U.S.
Dr. Marschall uses the example of an outbreak of vancomycin-resistant Enterococcus faecium (VRE) that began in late 2017, in Bern University Hospital, Switzerland, and went on until July 2020, receiving substantial media attention and becoming Switzerland's largest-ever multidrug-resistant organism outbreak.
The investigations into the outbreak revealed most VRE affected patients were colonized and not infected (a "silent outbreak"); that isolation of VRE patients was costly (requiring isolation rooms, personal protective equipment and bed closures); and that screening for VRE required a substantial logistical and financial effort (screening were proximity-based, captured entire floors, or were even hospital-wide).
Dr. Marschall and colleagues then re-analyzed the medical records generated during the first two years of the outbreak period (1/2018–12/2019) and identified (and mostly confirmed) risk factors for VRE colonization by using various statistical methods and then moved to a framework called network graph theory and graph neural networks (a type of AI).
Briefly, network graphs inspect the connections between discrete "nodes" in a network (which could be patients, rooms, devices) and determines, for example, which nodes are most connected or which nodes have the shortest path to another node (and thus play a larger role in the outbreak).
Dr. Marschall explains, "The more traditional methods for analyzing outbreaks might yield signals that tend to confirm previously known and often generic risk factors, without adding the detail needed to make specific interventions."
Extrapolating from their preliminary work in the field, Dr. Marschall thinks AI-based analyses could ultimately provide answers to key questions in an outbreak—for example, their study showed that the "electrocardiography service" and the "examination room ZZ" were at the center of many interactions, and thus could likely have served as a place of transmission—in consequence, that room/device/person could be the target for disinfection/interventions.
However, in the current study, Dr. Marschall explains that not all employee interactions were captured because not every profession logs their time with a patient as well as, for example, nursing personnel do (which leaves gaps in our understanding of the many interactions happening in an acute care hospital). To extract the maximum of information, all interactions between patients, employees, visitors, rooms, and devices would have to be captured.
Also, Dr. Marschall and colleagues based their work on the local data infrastructure in Bern—while the general approach they describe can be used for any setting, a given hospital would need to ensure its data is in an analyzable format and labeled so as to facilitate interpretation.
To make AI input into infectious disease outbreaks a success, Dr. Marschall explains that hospital teams must pivot from research to operations, meaning implementing and refining AI tools as an outbreak happens in real time (which is not easy to predict).
He states, "This approach could even help with individual patients infected with a multidrug-resistant organism or small clusters of such patients because it could identify surrounding patients/employees and rooms/devices that would need to addressed, either by screening or by targeted disinfection. The beauty of AI in outbreak management (and where its greatest power lies) is to make real-time or near real-time operational decisions easier, quicker and more precise."
In his talk, Dr. Marschall will highlight that novel approaches to medical data (such as network graphs and temporal graph neural networks) can provide us with the framework to elevate outbreak investigations to the next stage. It can identify specific "hot spots" of an outbreak, where transmissions are likely to happen, and give us the tools to target these hot spots in order to fight an outbreak. He concludes, "If this help comes to infection prevention experts in near real time, it will dramatically improve their ability to respond to an outbreak."