This article has been reviewed according to Science X's editorial process and policies. Editors have highlighted the following attributes while ensuring the content's credibility:
fact-checked
trusted source
proofread
Using machine learning to identify patients with cancer that would benefit from immunotherapy
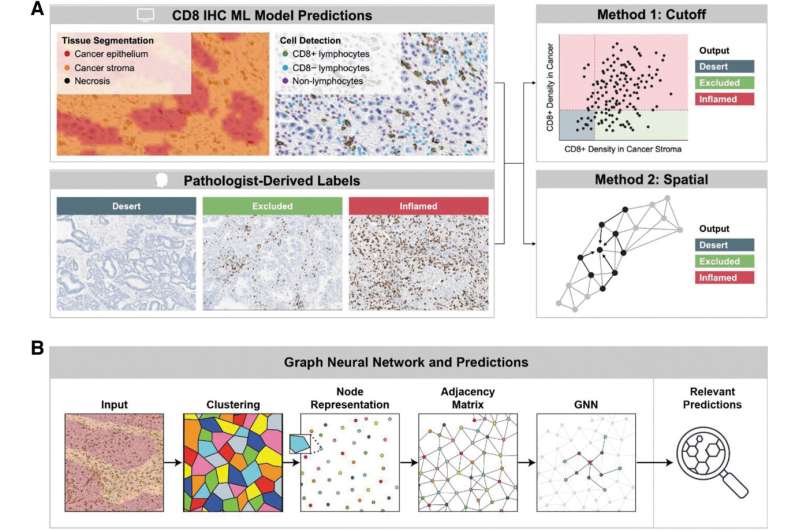
A new study examines the development of two machine learning models to classify the immunophenotype of a cancer specimen.
The digital pathology approach presented can characterize and classify cancer immunophenotypes in a reproducible and scalable fashion, holding promise for the application of such a. method to identify patients that may benefit from immunotherapy in non-small cell lung cancer (NSCLC), according to the study published in AI in Precision Oncology.
The cellular composition of the tumor immune microenvironment is a key contributor to the response of the tumor to immunotherapy. TGF-ß signaling is known to promote immune exclusion, where CD8+ T cells are in the surrounding stromal tissue but not within the tumor itself.
To better identify patients who are immune-excluded, Rui Wang, from Sanofi, and co-authors developed two machine learning models to quantify CD8+ cell positivity and classify the immunophenotype of a cancer specimen in patients with NSCLC.
"Our results support the potential use of machine learning-predicted cancer immunophenotypes to identify patients that may benefit from immunotherapy and TGF-ß blockage in NSCLC," concluded the investigators.
"This research points towards improvements in patient identification for drug candidacy, utilizing AI and machine learning to pinpoint precise biomarkers for immunotherapy in NSCLC. It signifies progress towards personalized medicine, promising treatments tailored to individual patient profiles for greater effectiveness and minimized side effects."
"Essentially, it emphasizes the importance of directing new treatments to the right patients, paving the way for a new era of precision in cancer care," says Douglas Flora, MD, Editor-in-Chief of AI in Precision Oncology.
More information: Robert J. Pomponio et al, Classification of the Tumor Immune Microenvironment Using Machine-Learning-Based CD8 Immunophenotyping As a Potential Biomarker for Immunotherapy and TGF-β Blockade in Nonsmall Cell Lung Cancer, AI in Precision Oncology (2024). DOI: 10.1089/aipo.2023.0008