Harnessing reliability for neuroscience research
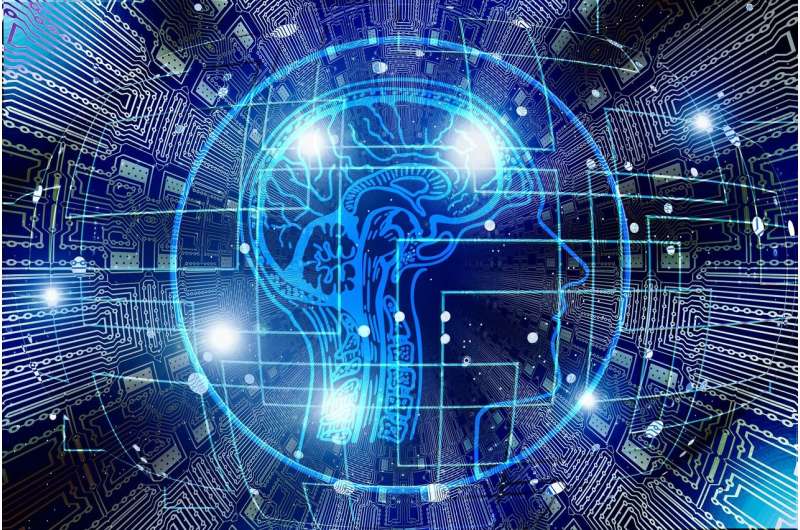
The neuroimaging community has made significant strides toward collecting large-scale neuroimaging datasets, which, until the past decade, had seemed out of reach. Between initiatives focused on the aggregation and open sharing of previously collected datasets and de novo data generation initiatives tasked with the creation of community resources, tens of thousands of datasets are now available online. These span a range of developmental statuses and disorders, and many more will soon be available.
Such open data sources are allowing researchers to increase the scale of their studies, to apply various learning strategies (for example, artificial intelligence) with ambitions of brain-based biomarker discovery, and to address questions regarding the reproducibility of findings, all at a pace that is unprecedented in imaging. However, based on the findings of recent works, few of the datasets generated to date contain enough data per subject to achieve highly reliable measures of brain connectivity. A new examination of this critical deficiency focuses on the field of neuroimaging, but the implications of its argument and the statistical principles discussed are broadly applicable.
Scoping the problem
The concern is simple: Researchers are amassing large-scale datasets through data sharing and coordinated data generation initiatives, but failing to optimize their data collections for relevant reliabilities (for example, test-retest, between raters, etc.). They may be collecting larger amounts of suboptimal data rather than smaller amounts of higher-quality data, a trade-off that does not bode well for the field, particularly when it comes to making inferences and predictions at the individual level. The new paper asserts that this misstep can be avoided by making upfront critical assessments of reliability.
The trade-off occurring in neuroimaging reflects a general tendency in neuroscience. Statistical power is fundamental to studies of individual differences, as it determines the ability to detect effects of interest. While sample size is readily recognized as a key determinant of statistical power, measurement reliabilities are less commonly considered, and at best are only indirectly considered when estimating required sample sizes. This is unfortunate, as statistical theory dictates that reliability places an upper limit on the maximum detectible effect size.
The interplay between reliability, sample size and effect size in determinations of statistical power is commonly underappreciated in the field. To facilitate a more direct discussion of these factors, Fig. 1 depicts the impact of measurement reliability and effect size on the sample sizes required to achieve desirable levels of statistical power (for example, 80 percent); these relations are not heavily dependent on the specific form of statistical inference employed (for example, two-sample t-test, paired t-tests, three-level ANOVA). Estimates were generated using the pwr package in R and are highly congruent with results from Monte Carlo simulations5. With respect to neuroscience, where the bulk of findings report effect sizes ranging from modest to moderate6, the figure makes obvious the point that increasing reliability can dramatically reduce the sample size requirements (and therefore cost) for achieving statistically appropriate designs.
In neuroimaging, the reliability of the measures employed in experiments can vary substantially. In MRI, morphological measures are known to have the highest reliability, with the most voxels in the brain exhibiting reliabilities measured as intraclass correlation >0.8 for core measures (for example, volume, cortical thickness and surface area). For functional MRI (fMRI) approaches, reliability tends to be lower and more variable, heavily dependent on the experimental design, the nature of the measure employed and—most importantly—the amount of data obtained (for example, for basic resting-state fMRI measures, the mean intra-class correlation obtained across voxels may increase by two to four times as one increases from five minutes to 30 minutes of data). Limited interindividual variability may be a significant contributor to findings of low reliability for fMRI, as its magnitude relative to within-subject variation is a primary determinant of reliability. Such a concern has been raised for task fMRI, which directly borrows behavioural task designs from the psychological literature.
Potential implications
From a statistical perspective, the risks of underpowered samples yielding increased false negatives and artificially inflated effect sizes (i.e., the 'winner's curse' bias) are well known. More recently, the potential for insufficiently powered samples to generate false positives has been established, as well. All these phenomena reduce the reproducibility of findings across studies, a challenge that other fields (for example, genetics) have long worked to overcome. In the context of neuroimaging or human brain mapping, an additional concern is that researchers may be biased to overvalue those brain areas for which measurement reliability is greater. For example, the default and frontoparietal networks receive more attention in clinical and cognitive neuroscience studies of individual and group differences. This could be appropriate, but it could also reflect the higher reliabilities of these networks.
Solutions
The goal is to draw greater attention to the need for assessment and optimization of reliability, which is typically underappreciated in neuroscience research. Whether focusing on imaging, electrophysiology, neuroinflammatory markers, microbiomics, cognitive neuroscience paradigms or on-person devices, it is essential that scientists consider measurement reliability and its determinants.
For MRI-based neuroimaging, a repeated theme across the various modalities (for example, diffusion, functional, morphometry) is that higher quality data require more time to collect, whether due to increased resolution or repetitions. As such, investigators would benefit from assessing the minimum data requirements to achieve adequately reliable measurements before moving forward. An increasing number of resources are available for such assessments of reliability (for example, Consortium for Reliability and Reproducibility, MyConnectome Project, Healthy Brain Network Serial Scanning Initiative, Midnight Scan Club, Yale Test-Retest Dataset, PRIMatE Data Exchange). It is important to note that these resources are primarily focused on test-retest reliability, leaving other forms of reliability less explored (for example, interstate reliability, inter-scanner reliability; see recent efforts from a Research Topic on reliability and reproducibility in functional connectomics).
Importantly, reliability will differ depending on how a given imaging dataset is processed and which brain features are selected. A myriad of processing strategies and brain features have emerged, but they are rarely compared with one another to identify those most suitable for studying individual differences. In this regard, efforts to optimize analytic strategies for reliability are essential, as they make it possible to decrease the minimum data required per individual to achieve a target level of reliability. This is critically important for applications in developing, aging and clinical populations, where scanner environment tolerability limits the ability to collect time-intensive datasets. An excellent example of quantifying and optimizing for reliability comes from functional connectomics. Following convergent reports that at least 20-30 min of data are needed to obtain test- retest reliability for traditional pairwise measures of connectivity, recent works have suggested the feasibility of combining different fMRI scans in a session (for example, rest, movie, task) to make up the differential in calculating reliable measures of functional connectivity.
Cognitive and clinical neuroscientists should be aware that many cognitive paradigms used inside and outside of the scanner have never been subject to proper assessments of reliability, and the quality of reliability assessments for questionnaires (even proprietary) can vary substantially.
As such, the reliability of data being used on the phenotyping side is often an unknown in the equation and can limit the utility of even the most optimal imaging measures, a reality that also affects other fields (for example, genetics) and inherently compromises such efforts. Although not always appealing, an increased focus on the quantification and publication of minimum data requirements and their reliabilities for phenotypic assessments is a necessity, as is exploration of novel approaches to data capture that may increase reliability (for example, sensor-based acquisition via wearables and longitudinal sampling via smartphone apps).
Finally, and perhaps most critically, there is marked diversity in how the word 'reliability' is used, and a growing number of separate reliability metrics are appearing. This phenomenon is acknowledged in a recent publication by an Organization for Human Brain Mapping workgroup tasked with generating standards for improving reproducibility. The researchers suggest it would be best to build directly on the terminology and measures well-established in other literatures (for example, statistics, medicine) rather than start anew. They particularly want to avoid confusions in terminology, particularly those between 'reliability' and 'validity," two related but distinct concepts that are commonly used interchangeably in the literature.
A confusion to avoid
It is crucial that researchers acknowledge the gap between reliability and validity, as a highly reliable measure can be driven by artifact rather than meaningful (i.e., valid) signal. As illustrated in Fig. 2, this point becomes obvious when one considers the differing sources of variance associated with the measurement of individual differences. First, there is the portion of the variance measured across individuals that is the trait of interest (Vt) (for example, between-subject differences in grey matter volume within left inferior frontal gyrus). Second, there is variance related to unwanted contaminants in the measurement that can systematically vary across individuals (Vc) (for example, between-subject differences in head motion). Finally, there is random noise (Vr), which is commonly treated as within-subject variation. Reliability is the proportion of the total variance that can be attributed to systematic variance across individuals (including both Vt and Vc; see equation 1); in contrast, validity is the proportion of the total variance that can be attributed specifically to the trait of interest alone (Vt; see equation 2).
- Reliability= (Vt+Vc)/(Vt+Vc +Vr ) (1)
- Validity =Vt/(Vt+Vc +Vr ) (2)
As discussed in prior work, this framework indicates that a measure cannot be more valid than reliable (i.e., reliability provides an upper bound for validity). So, while it is possible to have a measurement that is sufficiently reliable and completely invalid (for example, a reliable artifact), it is impossible to have a measurement with low reliability that has high validity.
A specific challenge for neuroscientists is that while reliability can be readily quantified, validity cannot, as it is not possible to directly measure Vt. As such, various indirect forms of validity are used, which differ in the strength of the evidence required. At one end is criterion validity, which compares the measure of interest to an independent measure designated as the criterion or 'gold standard' measurement (for example, comparison of individual differences in tracts identified by diffusion imaging to postmortem histological findings, or comparison of differences in fMRI-based connectivity patterns to intracranial measures of neural coupling or magnetoencephalography).
At the other extreme is face validity, in which findings are simply consistent with 'common sense' expectations (for example, does my functional connectivity pattern look like the motor system?). Intermediate to these are concepts such as construct validity, which test whether a measure varies as would be expected if it is indexing the desired construct (i.e., convergent validity) and not others (i.e., divergent validity) (for example, do differences in connectivity among individuals vary with developmental status and not head motion or other systematic artifacts?).
An increasingly common tool in the imaging community is predictive validity, where researchers test the ability to make predictions regarding a construct of interest (for example, do differences in the network postulated to support intelligence predict differences in IQ?). As can be seen from the examples provided, different experimental paradigms offer differing levels of validity, with the more complex and challenging offering the highest forms. From a practical perspective, what researchers can do is make best efforts to measure and remove artifact signals such as head motion and work to establish the highest form of validity possible using the methods available.
As neuroscientists make strides in their efforts to deliver clinically useful tools, it is essential that assessments and optimizations for reliability become common practice. This will require improved research practices among investigators, as well as support from funding agencies in the generation of open community resources upon which these essential properties can be quantified.
More information: Xi-Nian Zuo et al, Harnessing reliability for neuroscience research, Nature Human Behaviour (2019). DOI: 10.1038/s41562-019-0655-x
The code is available on GitHub at github.com/TingsterX/power__re … iability_sample_size