Using AI to diagnose birth defect in fetal ultrasound images
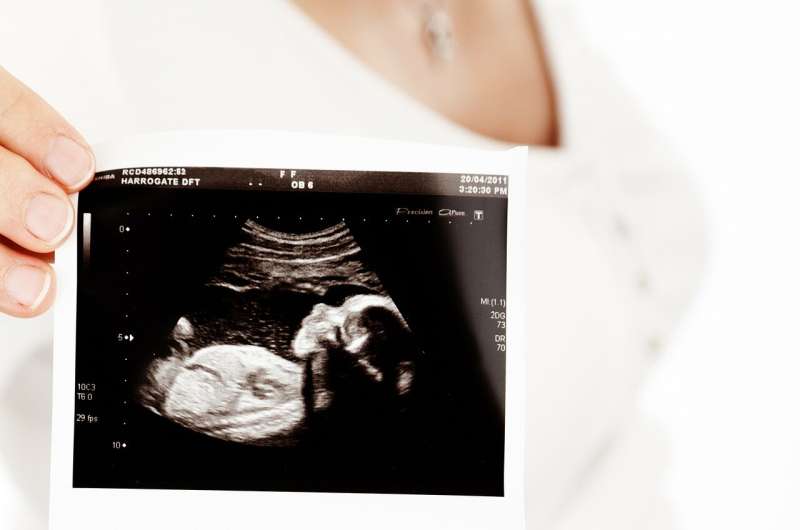
In a new proof-of-concept study led by Dr. Mark Walker at the uOttawa Faculty of Medicine, researchers are pioneering the use of a unique AI-based deep learning model as an assistive tool for the rapid and accurate reading of ultrasound images.
It's trailblazing work because although deep learning models have become increasingly popular in interpreting medical images and detecting disorders, figuring out how its application can work in obstetric ultrasonography is in its nascent stages. Few AI-enabled studies have been published in this field.
The goal of the team's study was to demonstrate the potential for deep-learning architecture to support early and reliable identification of cystic hygroma from first trimester ultrasound scans. Cystic hygroma is an embryonic condition that causes the lymphatic vascular system to develop abnormally. It's a rare and potentially life-threatening disorder that leads to fluid swelling around the head and neck.
The birth defect can typically be easily diagnosed prenatally during an ultrasound appointment, but Dr. Walker and his research group wanted to test how well AI-driven pattern recognition could do the job. The findings are promising.
Using a dataset of nearly 300 fetal ultrasounds collected retroactively at The Ottawa Hospital, images were analyzed using a DenseNet model to correctly identify cases of cystic hygroma compared to normal controls by calculating sensitivity, specificity, and other measurements. Gradient class activation heat maps—which visualize pixels in images—were also generated to assess model interpretability. Overall model accuracy was 93%.
"The model was outstanding, even with a small number of training images. And so, potentially, what we demonstrated was in the field of ultrasound we're able to use the same tools for image classification and identification with a high sensitivity and specificity," Dr. Walker says.
The findings were recently published in PLOS ONE, a peer-reviewed open access journal.
The uOttawa-led research group has high hopes for where this type of study might lead. With further development, including testing in a large multi-site dataset, the team believes their approach might be applied to other fetal anomalies generally identified by ultrasonography.
Down the line, Dr. Walker says the group will aim to develop an international consortium to upload obstetrical ultrasound images to "the cloud"—the euphemism for the use of remote servers to store data and provide computing services to swaths of users. This could ultimately help physicians in low- and middle-income countries achieve cloud-driven interpretation and diagnosis.
"This particular project is really meant to be the beginning of a large body of work," says Dr. Walker. "We've got several papers to follow this one."
Dr. Walker is a high-risk obstetrician and clinical epidemiologist who is a Full Professor and the Vice-Dean of Internationalization and Global Health at the uOttawa Faculty of Medicine. He is co-founder of the OMNI Research Group (Obstetrics, Maternal and Newborn Investigations) at The Ottawa Hospital, the largest maternal and newborn research group in Canada.
His collaborators on this publication include top research talent from uOttawa Faculty of Medicine departments and the Ottawa Hospital Research Institute (OHRI), where Dr. Walker is a senior scientist.
More information: Mark C. Walker et al, Using deep-learning in fetal ultrasound analysis for diagnosis of cystic hygroma in the first trimester, PLOS ONE (2022). DOI: 10.1371/journal.pone.0269323