Decoding syllables to show the limits of artificial intelligence
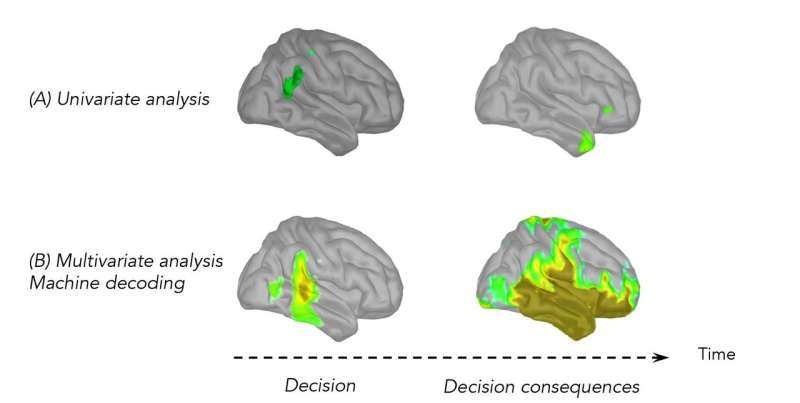
For the last decade, researchers have been using machine learning to decode human brain activity. Applied to neuroimaging data, these algorithms can reconstitute what we see, hear, and even what we think. For example, they show that words with similar meanings are grouped together in zones in different parts of our brain. However, by recording brain activity during a simple task—whether one hears "BA" or "DA"—neuroscientists from the University of Geneva (UNIGE), Switzerland, and the Ecole normale supérieure (ENS) in Paris now show that the brain does not necessarily use the regions of the brain identified by machine learning to perform a task. These regions reflect the mental associations related to the task. While machine learning is thus effective for decoding mental activity, it is not necessarily effective for understanding the specific information processing mechanisms in the brain. The results are available in the Proceedings of the National Academy of Sciences.
Modern neuroscientific data techniques have recently showed that the brain spatially organises the portrayal of word sounds, which researchers were able to map by region of activity. UNIGE neuroscientists thus asked how these spatial maps are used by the brain itself when it performs specific tasks. "We have used all the available human neuroimagery techniques to try to answer this question," says Anne-Lise Giraud, a professor at the Department of Basic Neurosciences of the UNIGE Faculty of Medicine.
UNIGE neuroscientists had 50 people listen to a continuum of syllables ranging from "BA" to "DA." The central phonemes were ambiguous and it was difficult to distinguish between the two options. They then used a functional MRI and magnetoencephalography to see how the brain behaves when the acoustic stimulus is clear or when it is ambiguous and requires an active mental representation of the phoneme and its interpretation by the brain. "We have observed that regardless of how difficult it is to classify the syllable that was heard, between 'BA' and 'DA,' the decision always engages a small region of the posterior superior temporal lobe," says Anne-Lise Giraud.
Neuroscientists then double-checked their results on a patient with an injury in the specific region of the posterior superior temporal lobe used to distinguish between "BA" and "DA." "And indeed, although the patient did not appear to have symptoms, he was no longer able to distinguish between the 'BA' and 'DA' phonemes ... this confirms that this small region is important in processing this type of phoneme information," says Sophie Bouton, a researcher from Anne-Lise Giraud's team.
But is the information on the identity of the syllable just locally present, or is it present more generally in the brain, as suggested by the maps produced via machine learning? To answer this question, the neuroscientists reproduced the "BA" / "DA" task with people who have electrodes directly implanted in their brains for medical reasons. This technique can collect very focal neural activity. A univariate analysis made it possible to see which region of the brain was solicited during the task, electrode by electrode, contact by contact. Solely the contacts in the posterior superior temporal lobe were active, thus confirming the results of the Geneva study.
However, when a machine-learning algorithm was applied to all of the data, thus making a multivariate decoding of data possible, positive results were observed in the entire temporal lobe, and even beyond it. "Learning algorithms are intelligent but ignorant," specifies Anne-Lise Giraud. "They are very sensitive and use all of the information in the signals. However, they do not allow us to know whether this information was used to perform the task, or if it reflects the consequences of this task—in other words, spreading information in our brain," continues Valérian Chambon, researcher at the Departement d'études cognitives at the ENS. The mapped regions outside of the posterior superior temporal lobe are thus false positives, in a way. These regions retain information on the decision that the subject makes ("BA" or "DA"), but aren't solicited to perform this task.
This research offers a better understanding of how our brain portrays syllables and, by showing the limits of artificial intelligence in certain research contexts, fosters welcome reflection on how to interpret data produced by machine learning algorithms.
More information: Sophie Bouton et al. Focal versus distributed temporal cortex activity for speech sound category assignment, Proceedings of the National Academy of Sciences (2018). DOI: 10.1073/pnas.1714279115