This article has been reviewed according to Science X's editorial process and policies. Editors have highlighted the following attributes while ensuring the content's credibility:
fact-checked
proofread
Can AI predict spatiotemporal distribution of dengue fever outbreaks with remote sensing data?
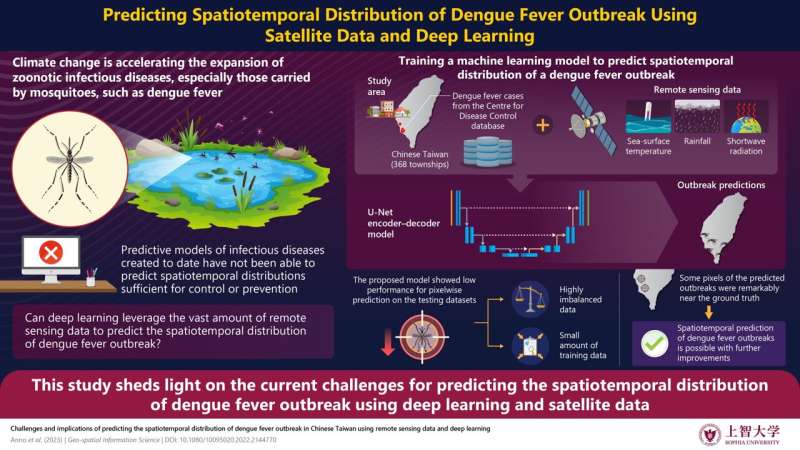
Outbreaks of zoonotic diseases, which are those transmitted from animals to humans, are globally on the rise due to climate change. In particular, the spread of diseases transmitted by mosquitoes is very sensitive to climate change, and Taiwan has seen a worrisome increase in the number of cases of dengue fever in recent years.
As for most known diseases, the popular saying "an ounce of prevention is worth a pound of cure" also rings true for dengue fever. Since there is still no safe and effective vaccine for all on a global scale, dengue fever prevention efforts rely on limiting places where mosquitoes can lay their eggs and giving people an early warning when an outbreak is likely to happen. However, thus far, there are no mathematical models that can accurately predict the location of dengue fever outbreaks ahead of time.
To address this issue, a research team including Professor Sumiko Anno from Sophia University, Japan, sought to combine artificial intelligence (AI) with remote sensing data to predict the spatiotemporal distribution of dengue fever outbreaks in Taiwan. This work, which was published in Geo-spatial Information Science, was co-authored by Hirakawa Tsubasa, Satoru Sugita, and Shinya Yasumoto, all from Chubu University, Ming-An Lee from National Taiwan Ocean University, and Yoshinobu Sasaki and Kei Oyoshi from the Japan Aerospace Exploration Agency (JAXA), Japan.
First, the team gathered climatic data of Taiwan from 2002 to 2020, including data on rainfall, sea-surface temperature, and shortwave radiation. They also gathered information on the place of residence of all reported dengue fever cases registered in the Taiwan Center for Disease Control. This enabled the researchers to prepare a labeled training dataset for the AI model, which should ideally be capable of finding hidden patterns between dengue fever cases and climatic parameters.
The AI model in question was a convolutional neural network (CNN) with a U-Net-based encoder–decoder architecture. "The U-Net model works with remarkably few training images and yields more precise semantic segmentation when provided with the location information," says Prof. Anno about the choice of AI model for their study. This well-established design usually performs well in image segmentation tasks, even when trained with few samples. After training the model, the team attempted to validate it using the remaining gathered data.
Unfortunately, the model did not perform as well as the researchers hoped it would. Most of the pixels on the map of Taiwan marked as predicted dengue fever outbreak locations did not match the original data. However, not all hope is lost for this approach, as Prof. Anno says, "While most of the predicted outbreak pixels did not overlap with the ground truth, some of them were located quite close to actual outbreak locations. This implies that the spatiotemporal prediction of dengue fever outbreaks using remote sensing data is possible."
Despite the low accuracy of the AI model, this study brought to light some of the current challenges of using remote sensing data for predicting the spatiotemporal distribution of zoonotic disease outbreaks. The research team believes that using a different model architecture, finding a way of balancing the training dataset, and gathering higher-resolution satellite data could all be promising ways to achieve the necessary performance.
More work will be required before we can use machine learning as a tool to pinpoint potential disease outbreak zones based on climatic data, but we must not falter. "Spatiotemporal visualizations generated by deep learning models could potentially guide the implementation of effective measures against disease outbreaks at the optimal time and location for disease prevention and control," concludes Prof. Anno.
More information: Sumiko Anno et al, Challenges and implications of predicting the spatiotemporal distribution of dengue fever outbreak in Chinese Taiwan using remote sensing data and deep learning, Geo-spatial Information Science (2023). DOI: 10.1080/10095020.2022.2144770