New diagnostic method may predict relapse risk for recovering prescription opioid addicts
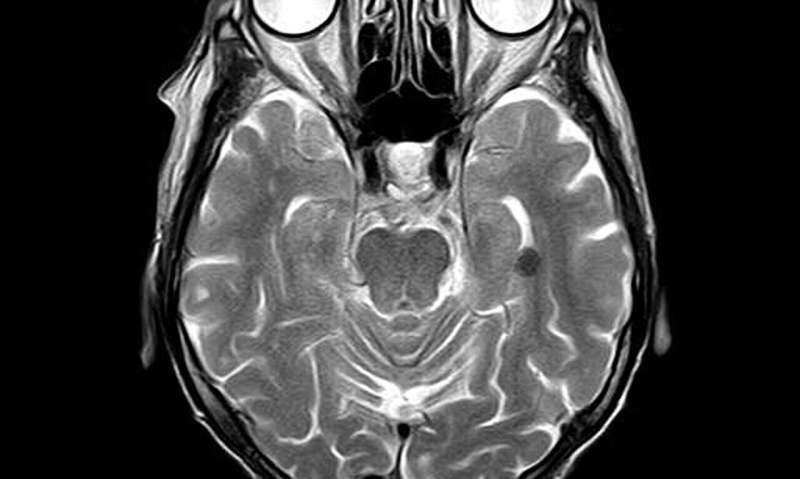
For the first time, Rutgers scientists have used a diagnostic technique in the opioid addiction field that they believe has the potential to determine which opioid-addicted patients are more likely to relapse.
Using an algorithm that looks for patterns in brain structure and functional connectivity, researchers were able to distinguish prescription opioid users from healthy participants. If treatment is successful, their brains will resemble the brain of someone not addicted to opioids.
"People can say one thing, but brain patterns do not lie," said Suchismita Ray, lead researcher and an associate professor in the Department of Health Informatics at Rutgers School of Health Professions. "The brain patterns that the algorithm identified from brain volume and functional connectivity biomarkers from prescription opioid users hold great promise to improve over current diagnosis."
In the study, published in NeuroImage: Clinical, Ray and her colleagues used MRIs to look at the brain structure and function in people diagnosed with prescription opioid use disorder who were seeking treatment compared to individuals with no history of using opioids.
The scans looked at the brain network believed to be responsible for drug cravings and compulsive drug use. At the completion of treatment, if this brain network has not changed, more treatment is needed.
"Our approach was able to segregate prescription opioid users from healthy participants based on both brain volume and functional connectivity data. We would not have been able to detect functional connectivity differences between the groups without the machine learning analysis. Opioid use disorder has reached epidemic proportions in the United States, raising an urgent need for diagnostic biological tools that can improve predictions of disease characteristics," Ray said in the study.
Individuals with opioid use disorder are currently being diagnosed based on self-reporting data they provide, which researchers say can be subject to biases. This machine learning algorithm, which quickly mines massive amounts of data, offers the potential to detect whether brain functional connectivity and structure in a recovering opioid user has returned to normal or near-to-normal levels following treatment.
Given the high rate of overdose deaths and relapse in opioid users, it is crucial to accurately diagnose opioid-addicted patients to improve treatment outcome and avoid overdose deaths, the researchers said.
More information: Ravi D. Mill et al, Structural MRI and functional connectivity features predict current clinical status and persistence behavior in prescription opioid users, NeuroImage: Clinical (2021). DOI: 10.1016/j.nicl.2021.102663