This article has been reviewed according to Science X's editorial process and policies. Editors have highlighted the following attributes while ensuring the content's credibility:
fact-checked
peer-reviewed publication
trusted source
proofread
New study shows how machine learning can improve care for people with Rett syndrome
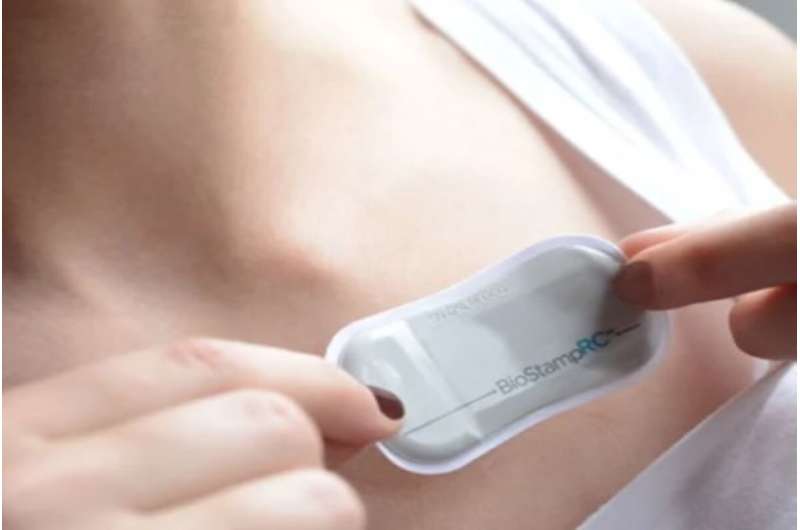
Over the last decade there has been a surge in the use of both medical-grade and consumer-grade wearable devices that measure an individual's physiology. The ability to monitor our own activities and health is more accessible than ever before.
While the application of these devices in biomedical research has largely been contained to cardiology, a new study demonstrates how the use of a wearable electronic chest patch combined with advancements in machine learning and artificial intelligence could aid in the development of new treatments for Rett syndrome, as well as a number of other health conditions.
Publishing in PLOS One, Dr. Gari Clifford and his research team at Emory University and the Georgia Institute of Technology used the MC10 Biostamp patch to analyze the movement and cardiac activity of people living with Rett syndrome.
Rett syndrome is a rare genetic neurodevelopmental disorder characterized by severe impairments, affecting a person's ability to speak, walk, eat and breathe normally. There is currently no cure for Rett syndrome and the ability to detect objective patterns of symptoms and disease progression directly in patients is lacking.
During the study period, the wearable patch monitored cardiac activity and movement of the study participants. Data collected over a continuous 48-hour period were then used to develop a machine learning algorithm, which identified patterns in physiology and movement that are specific to Rett syndrome severity. In particular, Clifford and his team found three specific patterns in the movement and heart rate (and how they influenced each other) that allowed them to accurately differentiate high- from low-symptom-severity individuals.
This resulted in a system that has the potential to objectively grade the severity of symptoms across the population based on their movement and cardiac activity. These data have major implications on efforts to improve therapeutic options for people living with Rett syndrome in future clinical trials.
Current options for measuring a treatment's effect rely on clinician- or parent-completed questionnaires. A physiologic measure derived from cardiac and movement activity that matches a patient's overall severity could be an important biomarker that indicates whether or not a treatment could help with other symptoms, such as communication and mobility, later on.
"This algorithm provides an objective metric that could be used to automatically assess the effect of a medication or other intervention on the symptoms experienced by a Rett syndrome sufferer," Clifford said. "We are excited that these biomarkers could potentially allow for more personalized and effective treatment in this population, and perhaps others."
The Rett Syndrome Research Trust (RSRT), the leading nonprofit organization dedicated to finding a cure for Rett syndrome, is sponsoring the research and providing additional funding to Clifford and his team to continue validating the work in a larger population.
"The results of this initial study exceeded our expectations and clearly demonstrate that direct and objective measures of patient symptoms are not only possible but sensitive enough to distinguish symptom severity, even with a small number of patients," said RSRT's Chief Scientific Officer, Jana von Hehn, Ph.D. "This work has the potential to shorten clinical trial timelines with more sensitive assessment of treatment effects and fewer patients. We are very excited to continue this important work with Dr. Clifford and his team of experts."
The use of artificial intelligence and machine learning has gained increased attention in clinical care in recent years for its ability to improve diagnosis and treatment for many diseases. This is the first time machine learning has been applied for people with Rett syndrome, but Clifford sees an opportunity to apply this model to other populations, such as people living with autism.
More information: Pradyumna Byappanahalli Suresha et al, Rett syndrome severity estimation with the BioStamp nPoint using interactions between heart rate variability and body movement, PLOS ONE (2023). DOI: 10.1371/journal.pone.0266351